Multichannel Domain Adaptation Graph Convolutional Networks-Based Fault Diagnosis Method and With Its Application
IEEE Transactions on Industrial Informatics(2023)
摘要
Intelligent fault diagnosis of the complex systems has made great progress based on the availability of massive labeled data. However, due to the diversity of working conditions and the lack of sufficient fault samples in practice, the generalization of the existing fault diagnosis methods are weak. To handle this issue, a multichannel domain adaptation graph convolutional network method is proposed. In the proposed network, a feature mapping layer based on convolutional neural network is used first to extract features from input data, which then are transmitted to the graph generator to construct two association graphs. After that, three distributed graph convolutional networks are used to extract the specific and common embeddings from two association graphs and their combination. Meanwhile, to fuse these embeddings adaptively, an attention mechanism is used to learn importance weights. Besides, a domain discriminator is leveraged to reduce the distribution discrepancy of different data domains. Finally, a label classifier is used to output fault diagnosis results. Two experimental studies with different signal types show that the proposed method not only presents better diagnosis performance than existing methods with few samples, but also can extract domain-invariant features for cross-domain under varying working conditions.
更多查看译文
AI 理解论文
溯源树
样例
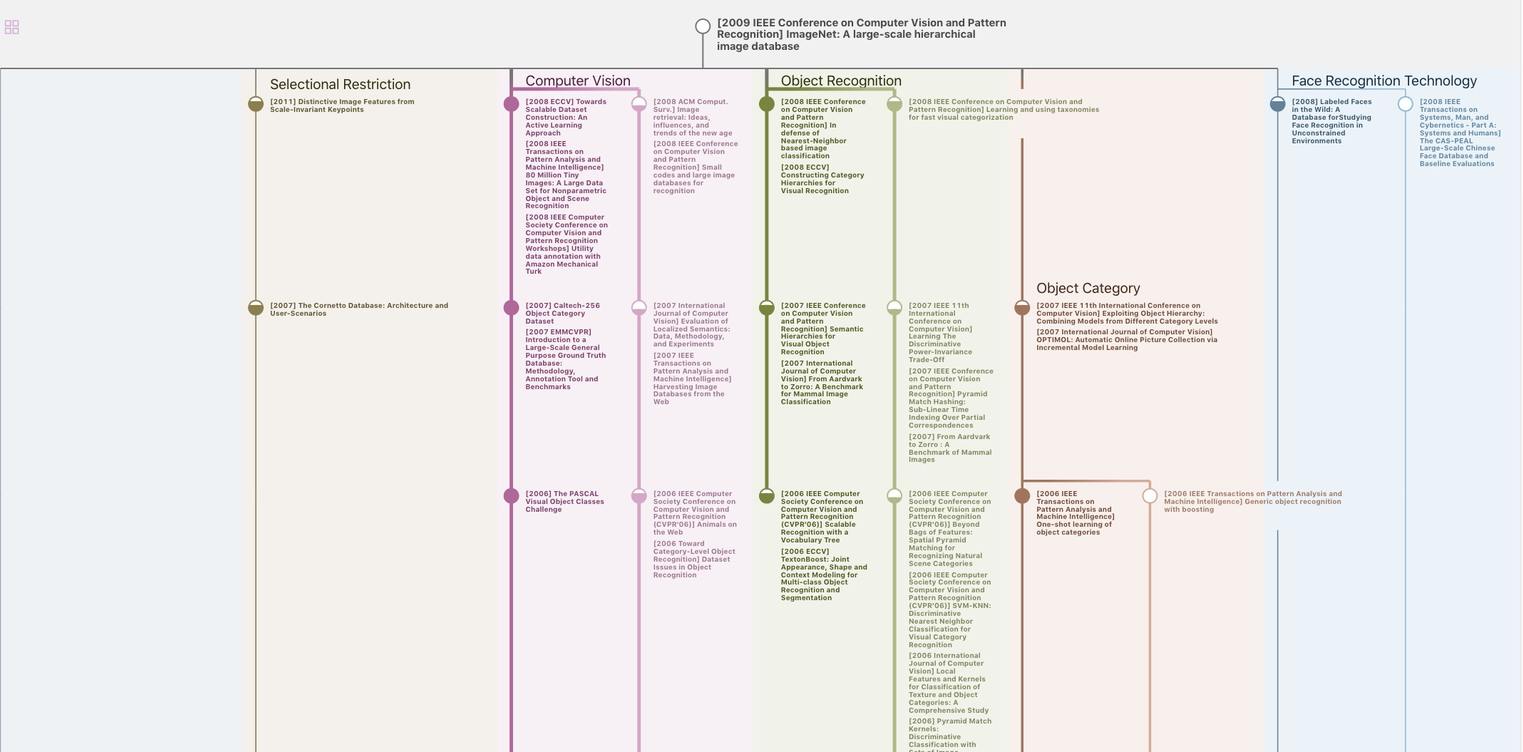
生成溯源树,研究论文发展脉络
Chat Paper
正在生成论文摘要