A CNN model based on innovative expansion operation improving the fault diagnosis accuracy of drilling pump fluid end
MECHANICAL SYSTEMS AND SIGNAL PROCESSING(2023)
摘要
Accurate fault diagnosis is critical to the safe and reliable operation of the drilling pump. The challenge in fault diagnosis of the fluid end of the drilling pump is to extract the fault features more accurately. This paper proposes an innovative expansion operation to convert the one-dimensional (1D) signal into three-dimensional (3D) images with 3 channels, which not only increases the visibility of feature information but also reduces the influence of noise. To increase the identification types and improve the identification accuracy, this paper provides a Con-volutional Neural Network (CNN) drilling pump fluid end fault diagnosis method based on the expansion operation. Firstly, to solve the problem that the feature information is not obvious in the 3D images, the expansion operation converts the 1D vibration signals into 3D images to generate images with more obvious features. Then, the AlexNet model is investigated by changing the number of neurons in the first fully connected layer. Compared to directly generated 3D images, grayscale images generated by Do's method, and grayscale images generated by Do's method combined with expansion operation, the diagnosis accuracy of the AlexNet model based on the 3D images generated by expansion operation is the highest. Finally, the experiment of the proposed fault diagnosis method of the fluid end of the drilling pump is carried out. Results show that the method proposed in this paper identifies 9 types of faults of the drilling pump's fluid end with a mean accuracy of 98.00%, which is significantly higher than that of other diagnostic methods. This research provides a new approach to more accurately diagnose the drilling pump fluid end faults.
更多查看译文
关键词
Drilling pump fluid end,Fault diagnosis,Vibration signal,Expansion operation,CNN AlexNet
AI 理解论文
溯源树
样例
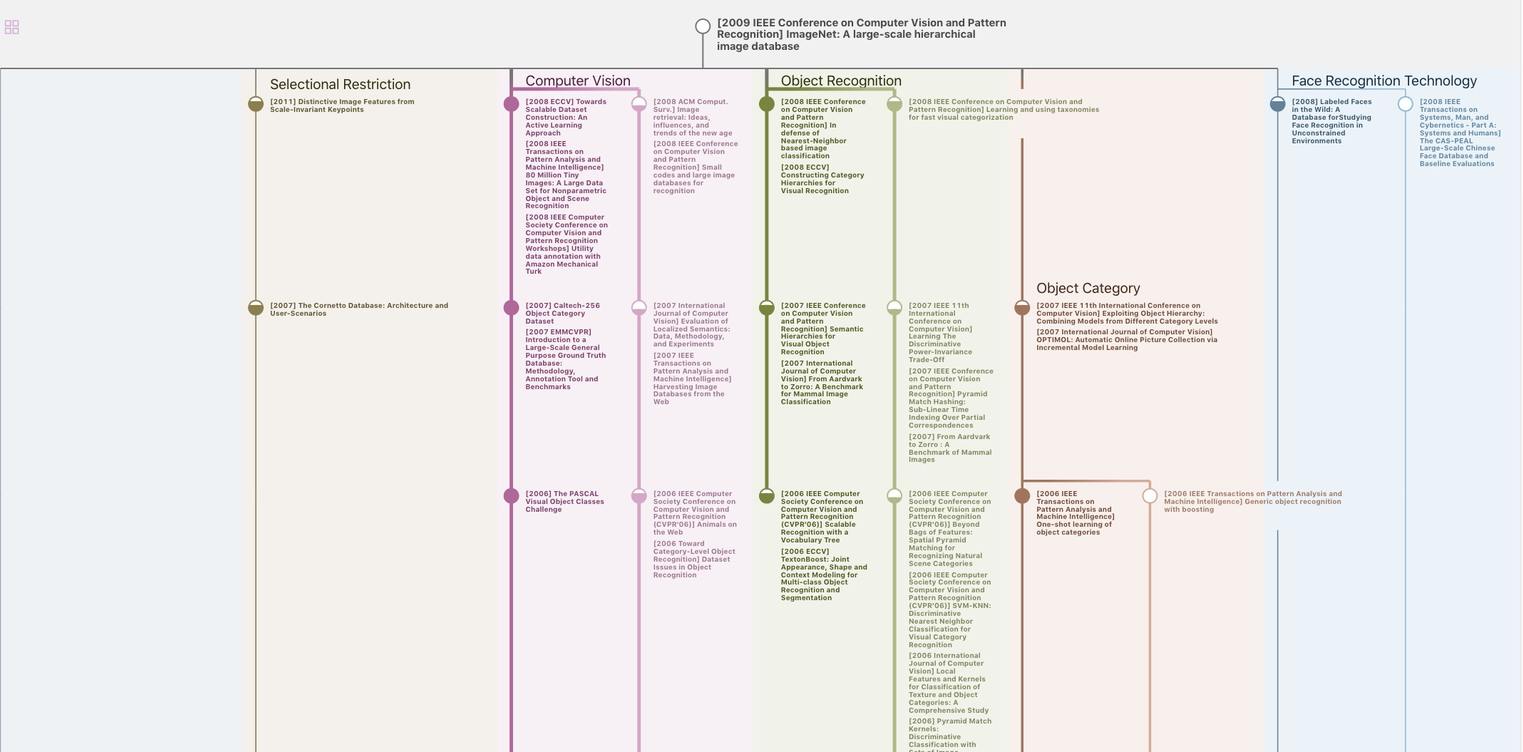
生成溯源树,研究论文发展脉络
Chat Paper
正在生成论文摘要