SkillS: Adaptive Skill Sequencing for Efficient Temporally-Extended Exploration
arxiv(2023)
摘要
The ability to effectively reuse prior knowledge is a key requirement when building general and flexible Reinforcement Learning (RL) agents. Skill reuse is one of the most common approaches, but current methods have considerable limitations.For example, fine-tuning an existing policy frequently fails, as the policy can degrade rapidly early in training. In a similar vein, distillation of expert behavior can lead to poor results when given sub-optimal experts. We compare several common approaches for skill transfer on multiple domains including changes in task and system dynamics. We identify how existing methods can fail and introduce an alternative approach to mitigate these problems. Our approach learns to sequence existing temporally-extended skills for exploration but learns the final policy directly from the raw experience. This conceptual split enables rapid adaptation and thus efficient data collection but without constraining the final solution.It significantly outperforms many classical methods across a suite of evaluation tasks and we use a broad set of ablations to highlight the importance of differentc omponents of our method.
更多查看译文
关键词
Reinforcement Learning,Control,Skills,Priors,Hierarchical Reinforcement Learning
AI 理解论文
溯源树
样例
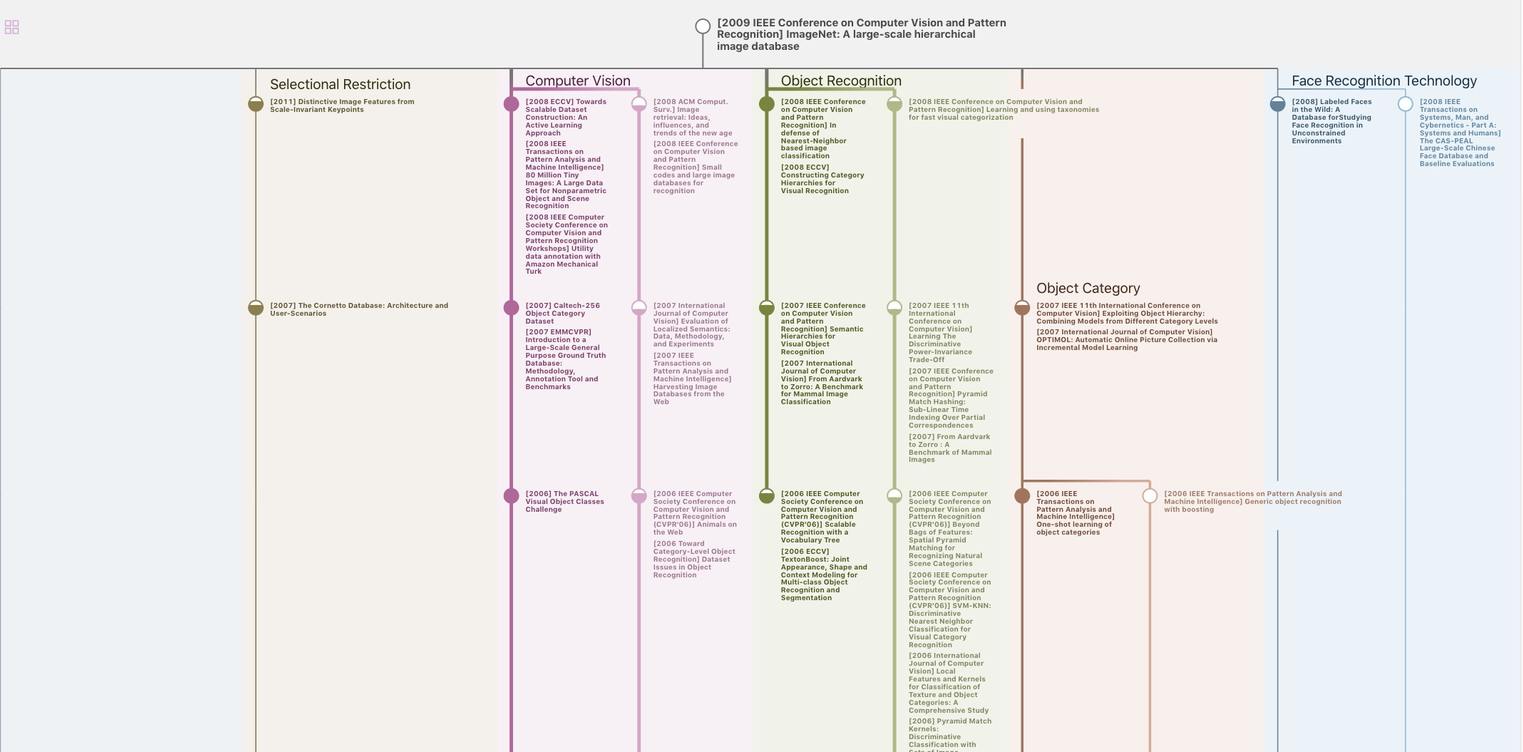
生成溯源树,研究论文发展脉络
Chat Paper
正在生成论文摘要