Deep-learning-assisted Sort-Seq enables high-throughput profiling of gene expression characteristics with high precision
biorxiv(2022)
摘要
As an essential physiological process, gene expression determines the function of each cell. However, owing to the complex nondeterministic and nonlinear nature of gene expression, the steady-state intracellular protein abundance of a clonal population forms a distribution. The characteristics of this distribution, including expression strength and noise, are closely related to cellular behavior. Therefore, quantitative description of these characteristics is an important goal in biology. This task, however, has so far relied on arrayed methods, which are time-consuming and labor-intensive. To address this issue, we propose a deep-learning-assisted Sort-Seq approach (dSort-Seq) in this work, enabling high-throughput profiling of expression properties with high precision. We demonstrated the validity of dSort-Seq for large-scale assaying of the dose‒response relationships of biosensors. In addition, we comprehensively investigated the contribution of transcription and translation to noise production in E. coli, from which we discovered that the expression noise is strongly coupled with the mean expression level instead of translation strength, even in the case of weak transcription. We also discovered that the transcriptional interference caused by overlapping RpoD-binding sites contributes to noise production, which suggested the existence of a simple and feasible noise control strategy in E. coli. Overall, dSort-Seq is able to efficiently determine the strength-noise landscape, which has promising applications in studies related to gene expression.
### Competing Interest Statement
The authors have declared no competing interest.
更多查看译文
关键词
gene expression,gene expression characteristics,deep-learning-assisted deep-learning-assisted,sort-seq,high-throughput
AI 理解论文
溯源树
样例
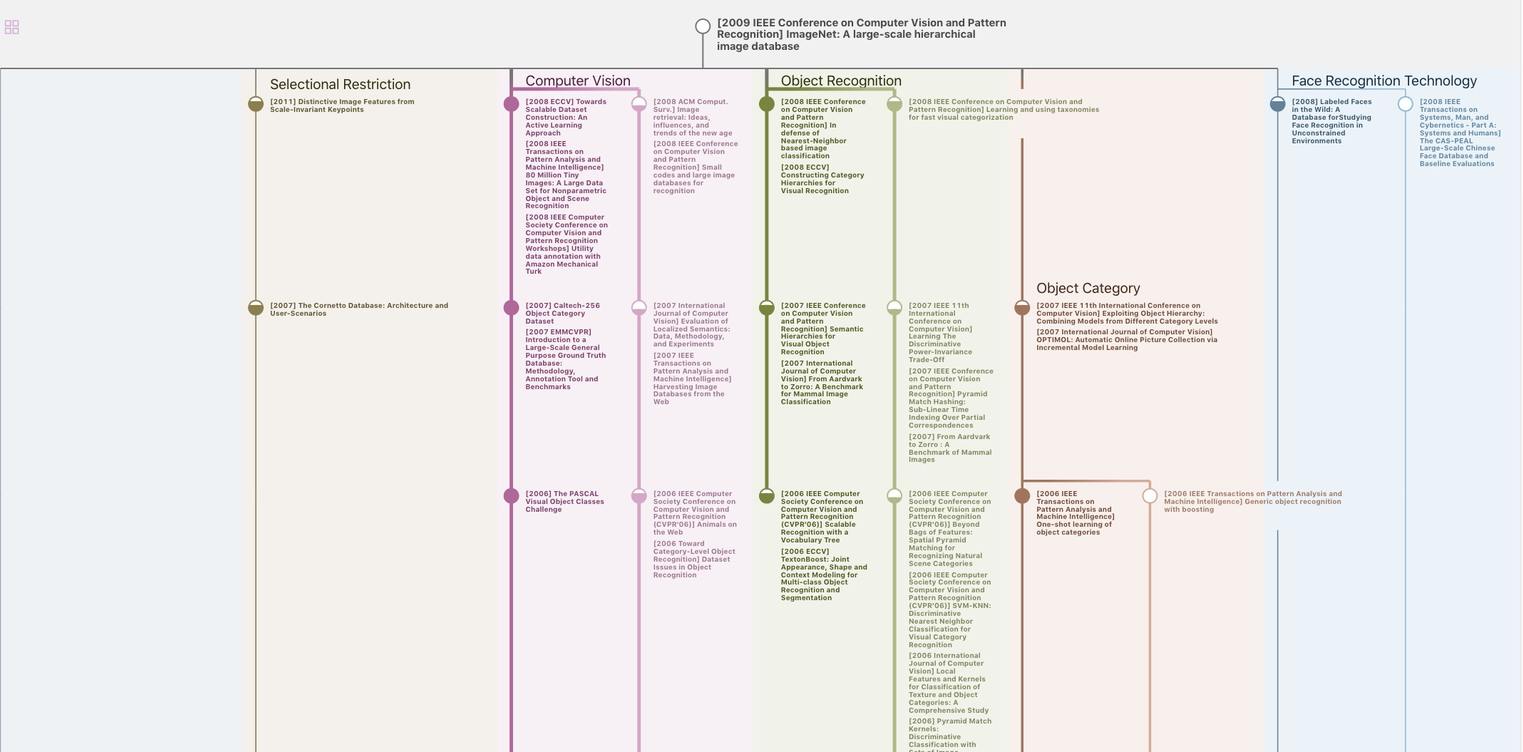
生成溯源树,研究论文发展脉络
Chat Paper
正在生成论文摘要