Health index construction with feature fusion optimization for predictive maintenance of physical systems
STRUCTURAL AND MULTIDISCIPLINARY OPTIMIZATION(2022)
摘要
In any prognostics and health management framework, the key to accurate remaining useful life prediction is finding a signal that directly quantifies the health status of a physical asset. When a direct measurement of an asset’s health is not available, researchers and practitioners develop virtual health indices, fused from many different signals, to quantify a unit’s health status. However, current metrics and methods used to engineer virtual health indices struggle to deal with large signal noises and do not consider health index performance on a population of units wholistically. In response to these challenges, we propose a new trend metric, which more accurately quantifies the monotonicity of a signal in the presence of noise, as well as a new probabilistic method, called meta-probability, for comparing health indices across a population of units to better understand the unit-to-unit variation in health index performance. To demonstrate the utility of our proposed trend metric and probabilistic comparison tool, we formulate a multi-objective optimization problem with the goal of creating the best virtual health index across a population of units by fusing many sensor signals and derived features. The proposed metrics and optimization scheme are evaluated in two case studies considering rolling element bearing run-to-failure data. The proposed optimization method, which considers the newly proposed monotonicity metric as one of the objectives, is found to create a health index that is more optimal for a larger percentage of the units in the population than five existing health index construction methodologies reported in the literature.
更多查看译文
关键词
Health index,Optimization,Prognostics,Remaining useful life prediction,Feature fusion,Time series metrics,Monotonicity
AI 理解论文
溯源树
样例
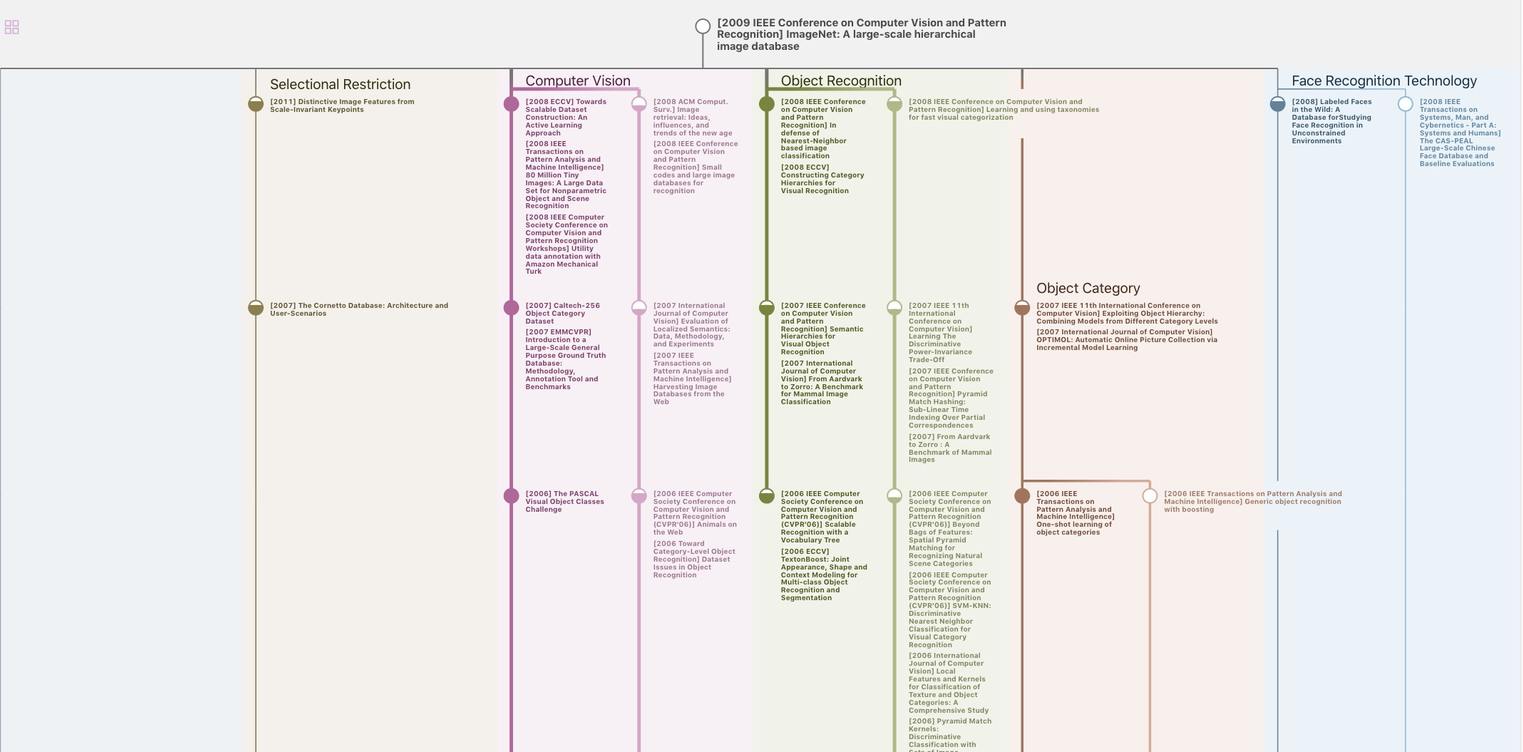
生成溯源树,研究论文发展脉络
Chat Paper
正在生成论文摘要