Prediphant: Short Term Heavy User Prediction
2022 13th International Symposium on Communication Systems, Networks and Digital Signal Processing (CSNDSP)(2022)
摘要
Traffic prediction is of paramount importance for the correct management of network infrastructures. Most research efforts try to forecast the aggregated traffic over the network and over large time windows. In this work, we tackle the problem the other way around. That is, we predict the behaviour of individual users over short time windows. First, we investigate the contribution of the most data eager users to the global network traffic. We do it by analyzing network traces coming from several thousand real users. Then, we design a technique, based on a combination of Natural Language Processing (NLP) and Long Short-Term Memory (LSTM) machine learning models, that leverages past navigation patterns to predict sudden changes in the amount of resources consumed by each user. Finally, we evaluate our method using real data finding it is able to predict about 80% of the users that will rump up their network needs in most realistic scenarios.
更多查看译文
关键词
aggregated traffic,short time windows,data eager users,global network traffic,Natural Language Processing,Long Short-Term Memory machine learning models,Short Term heavy user prediction,traffic prediction,correct management,network infrastructures
AI 理解论文
溯源树
样例
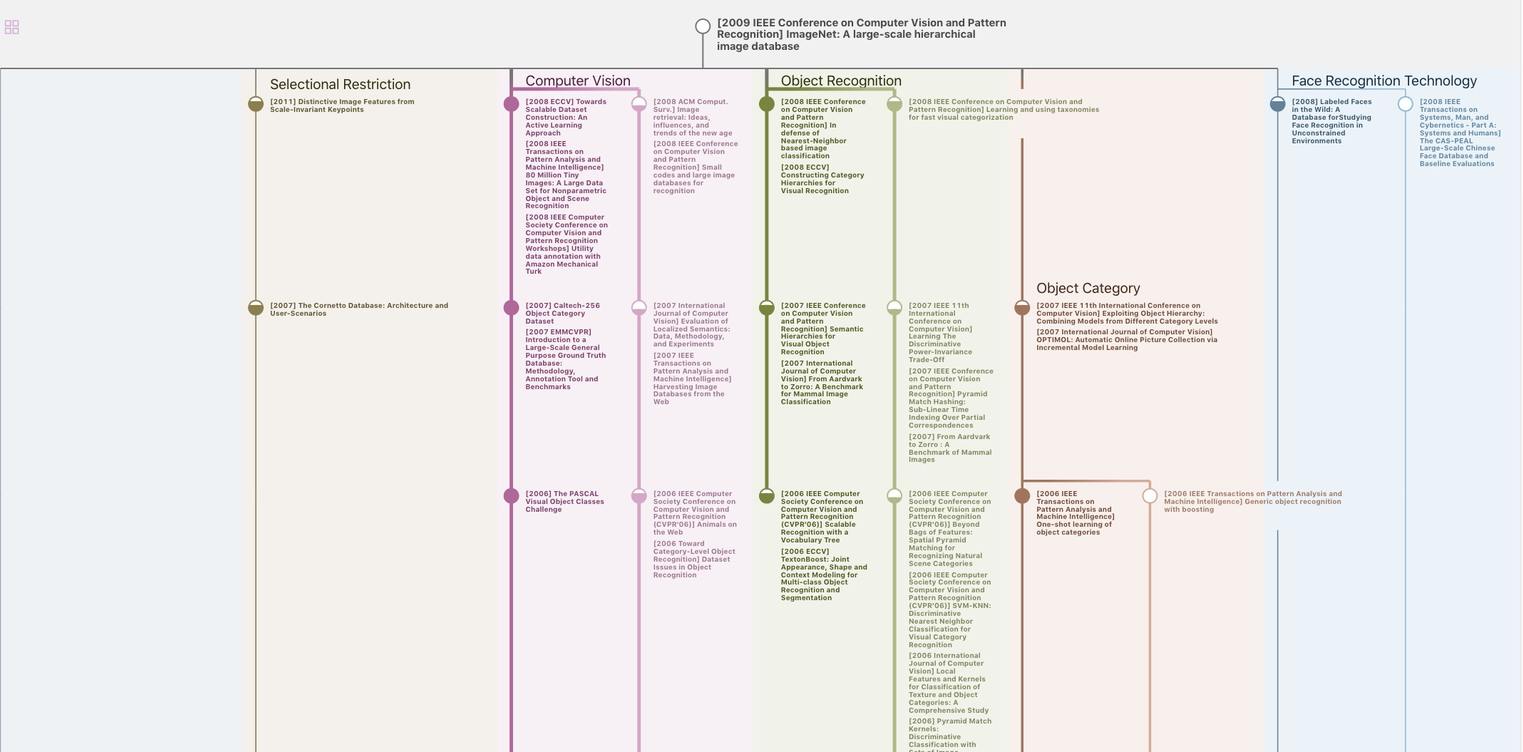
生成溯源树,研究论文发展脉络
Chat Paper
正在生成论文摘要