Relevant Safety Falsification by Automata Constrained Reinforcement Learning.
IEEE Conference on Automation Science and Engineering (CASE)(2022)
摘要
Complex safety-critical cyber-physical systems, such as autonomous cars or collaborative robots, are becoming increasingly common. Simulation-based falsification is a testing method for uncovering safety hazards of such systems already in the design phase. Conventionally, the falsification method takes the form of a static optimization. Recently, dynamic optimization methods such as reinforcement learning have gained interest for their ability to uncover harder-to-find safety hazards. However, these methods may converge to risk-maximising, but irrelevant behaviors. This paper proposes a principled formulation and solution of the falsification problem by automata constrained reinforcement learning, in which rewards for relevant behavior are tuned via Lagrangian relaxation. The challenges and proposed methods are demonstrated in a use-case example from the domain of industrial human-robot collaboration, where falsification is used to identify hazardous human worker behaviors that result in human-robot collisions. Compared to random sampling and conventional approximate Q-learning, we show that the proposed method generates equally hazardous, but at the same time more relevant testing conditions that expose safety flaws.
更多查看译文
关键词
simulation-based falsification,safety hazards,design phase,static optimization,dynamic optimization methods,automata constrained reinforcement learning,relevant behavior,industrial human-robot collaboration,hazardous human worker,human-robot collisions,Q-learning,safety flaws,complex safety-critical cyber-physical systems,autonomous cars,collaborative robots,safety falsification
AI 理解论文
溯源树
样例
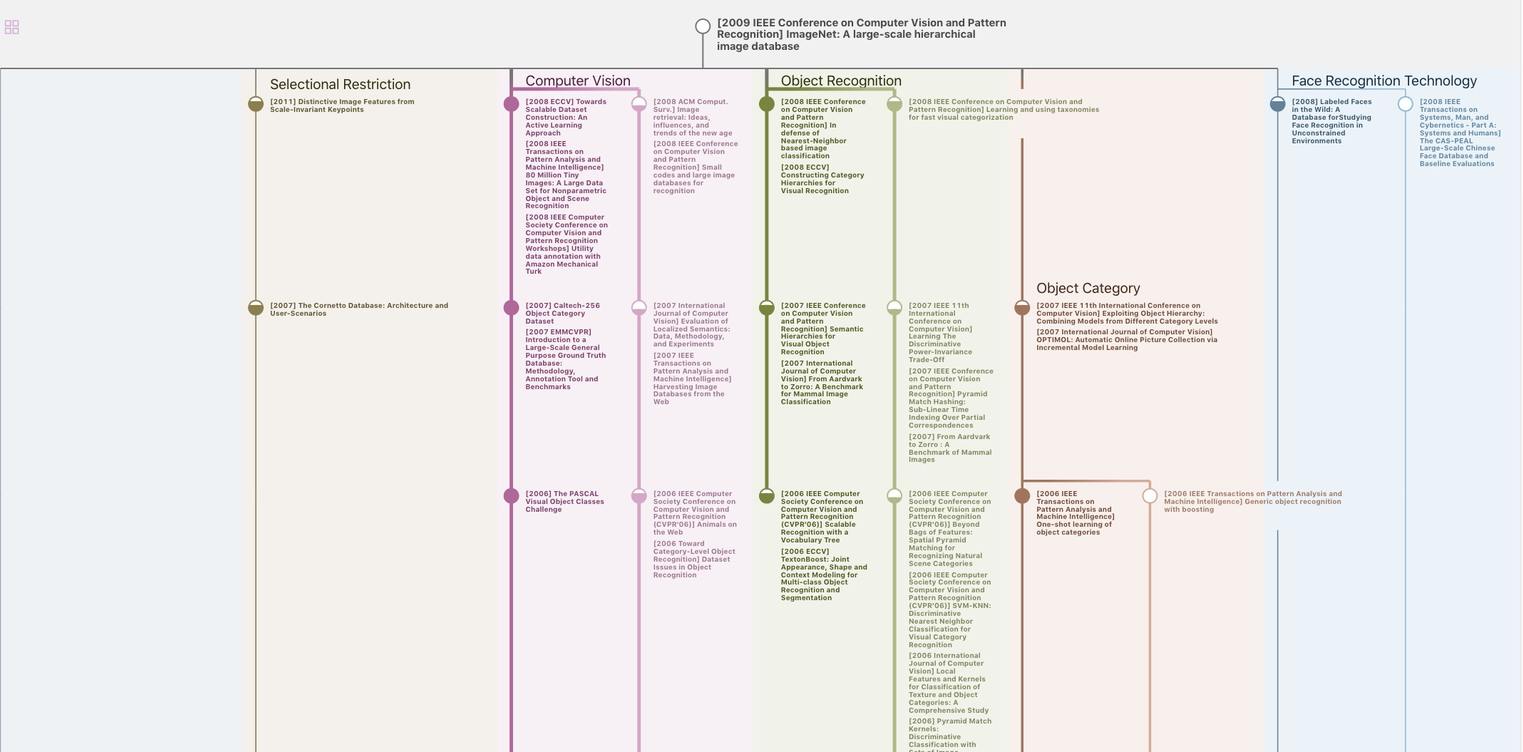
生成溯源树,研究论文发展脉络
Chat Paper
正在生成论文摘要