Speedup Training Artificial Intelligence for Mahjong via Reward Variance Reduction
2022 IEEE Conference on Games (CoG)(2022)
摘要
Despite significant breakthroughs in developing gaming artificial intelligence (AI), Mahjong remains quite challenging as a popular multi-player imperfect information game. Compared with games such as Go and Texas Hold’em, Mahjong has much more invisible information, unfixed game order, and a complicated scoring system, resulting in high randomness and variance of the rewarding signals during the reinforcement learning process. This paper presents a Mahjong AI by introducing Reward Variance Reduction (RVR) into a new self-play deep reinforcement learning algorithm. RVR handles the invisibility via a relative value network which leverages the global information to guide the model to converge to the optimal strategy under an oracle with perfect information. Moreover, RVR improves the training stability using an expected reward network to adapt to the complex, dynamic, and highly stochastic reward environment. Extensive experimental results show that RVR significantly reduces the variance in Mahjong AI training and improves the model performance. After only three days of self-play training on a single server with 8 GPUs, RVR defeats 62.5% opponents on the Botzone platform.
更多查看译文
关键词
Imperfect information game,multi-agent learning,reinforcement learning,Mahjong AI
AI 理解论文
溯源树
样例
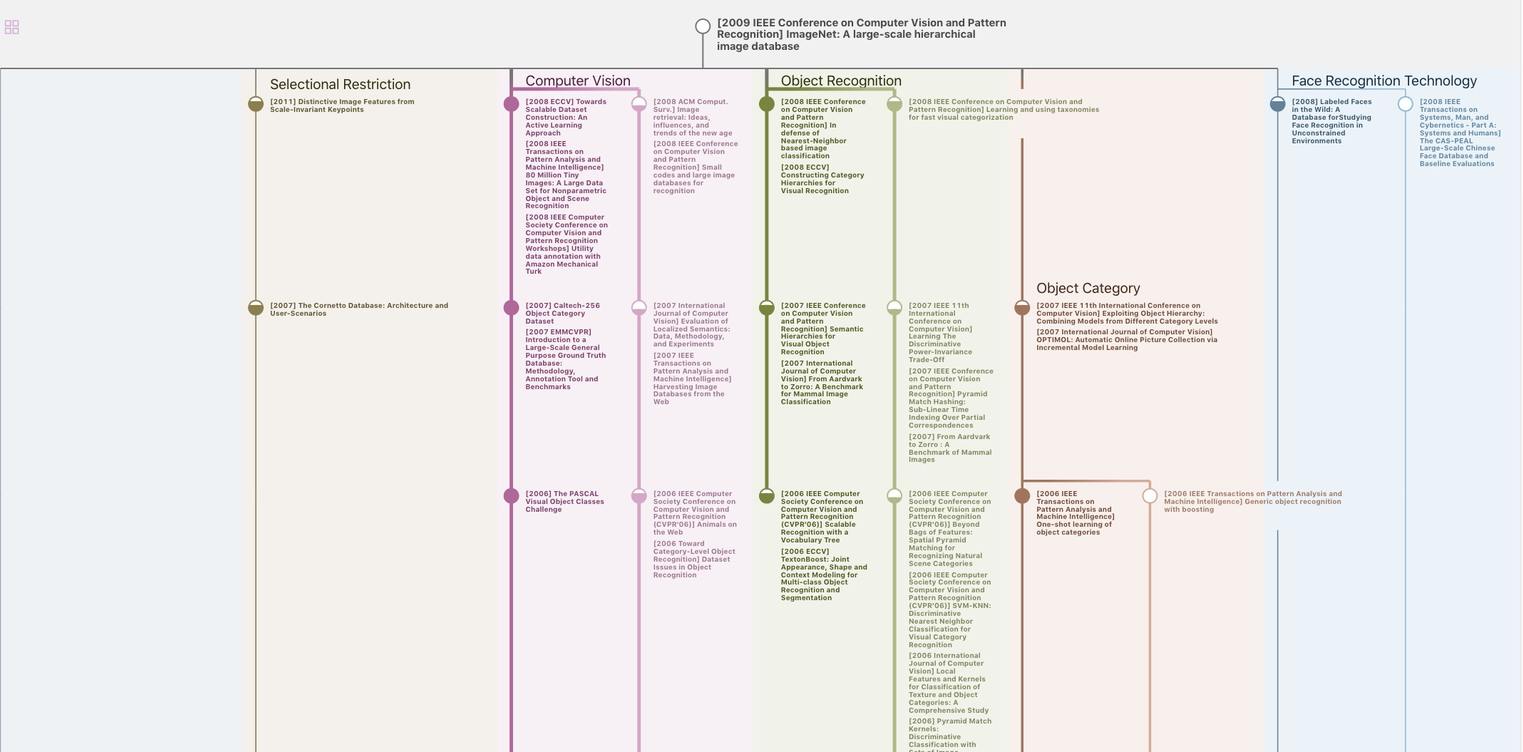
生成溯源树,研究论文发展脉络
Chat Paper
正在生成论文摘要