Privacy-Preserving Student Learning with Differentially Private Data-Free Distillation
2022 IEEE 24th International Workshop on Multimedia Signal Processing (MMSP)(2022)
摘要
Deep learning models can achieve high inference accuracy by extracting rich knowledge from massive well-annotated data, but may pose the risk of data privacy leakage in practical deployment. In this paper, we present an effective teacher-student learning approach to train privacy-preserving deep learning models via differentially private data-free distillation. The main idea is generating synthetic data to learn a student that can mimic the ability of a teacher well-trained on private data. In the approach, a generator is first pretrained in a data-free manner by incorporating the teacher as a fixed discriminator. With the generator, massive synthetic data can be generated for model training without exposing data privacy. Then, the synthetic data is fed into the teacher to generate private labels. Towards this end, we propose a label differential privacy algorithm termed selective randomized response to protect the label information. Finally, a student is trained on the synthetic data with the supervision of private labels. In this way, both data privacy and label privacy are well protected in a unified framework, leading to privacy-preserving models. Extensive experiments and analysis clearly demonstrate the effectiveness of our approach.
更多查看译文
关键词
differential privacy,teacher-student learning,knowledge distillation
AI 理解论文
溯源树
样例
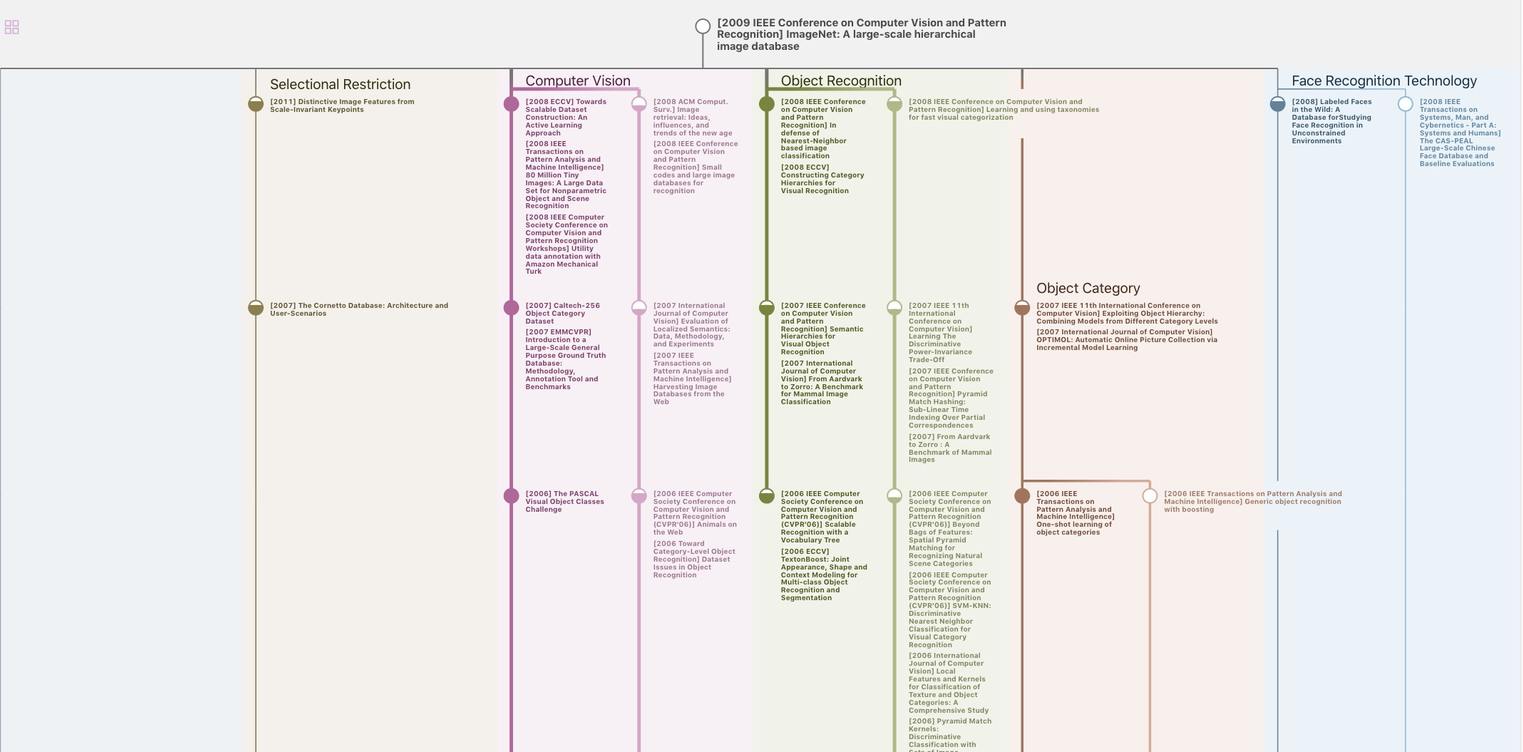
生成溯源树,研究论文发展脉络
Chat Paper
正在生成论文摘要