A Light-speed Linear Program Solver for Personalized Recommendation with Diversity Constraints
arxiv(2022)
摘要
We study a structured linear program (LP) that emerges in the need of ranking candidates or items in personalized recommender systems. Since the candidate set is only known in real time, the LP also needs to be formed and solved in real time. Latency and user experience are major considerations, requiring the LP to be solved within just a few milliseconds. Although typical instances of the problem are not very large in size, this stringent time limit appears to be beyond the capability of most existing (commercial) LP solvers, which can take $20$ milliseconds or more to find a solution. Thus, reliable methods that address the real-world complication of latency become necessary. In this paper, we propose a fast specialized LP solver for a structured problem with diversity constraints. Our method solves the dual problem, making use of the piece-wise affine structure of the dual objective function, with an additional screening technique that helps reduce the dimensionality of the problem as the algorithm progresses. Experiments reveal that our method can solve the problem within roughly 1 millisecond, yielding a 20x improvement in speed over efficient off-the-shelf LP solvers. This speed-up can help improve the quality of recommendations without affecting user experience, highlighting how optimization can provide solid orthogonal value to machine-learned recommender systems.
更多查看译文
关键词
personalized recommendation,diversity,program,light-speed
AI 理解论文
溯源树
样例
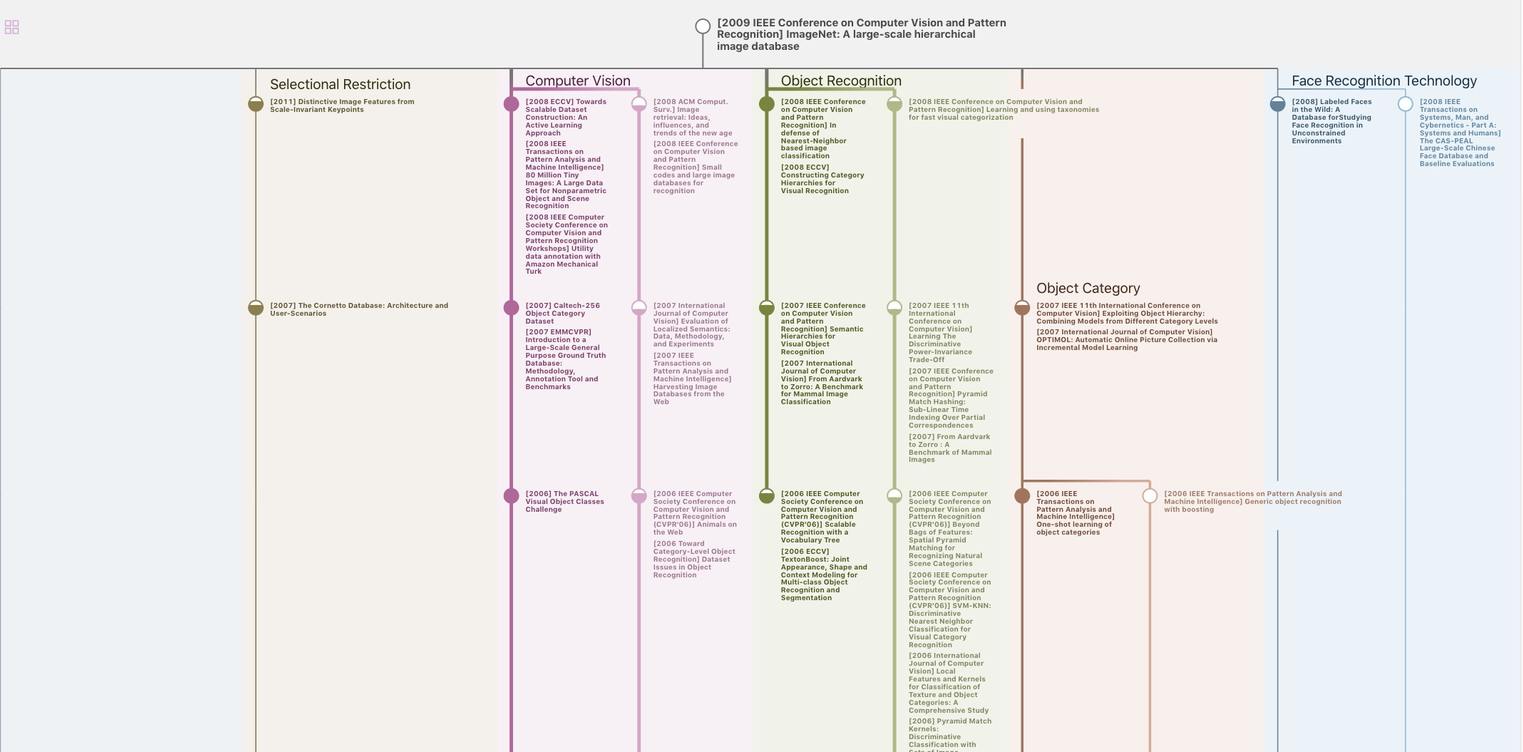
生成溯源树,研究论文发展脉络
Chat Paper
正在生成论文摘要