Towards Automated Polyp Segmentation Using Weakly- and Semi-Supervised Learning and Deformable Transformers
arxiv(2023)
摘要
Polyp segmentation is a crucial step towards computer-aided diagnosis of colorectal cancer. However, most of the polyp segmentation methods require pixel-wise annotated datasets. Annotated datasets are tedious and time-consuming to produce, especially for physicians who must dedicate their time to their patients. To this end, we propose a novel weakly- and semi-supervised learning polyp segmentation framework that can be trained using only weakly annotated images along with unlabeled images making it very cost-efficient to use. More specifically our contributions are: 1) a novel weakly annotated polyp dataset, 2) a novel sparse foreground loss that suppresses false positives and improves weakly-supervised training, 3) a deformable transformer encoder neck for feature enhancement by fusing information across levels and flexible spatial locations.Extensive experimental results demonstrate the merits of our ideas on five challenging datasets outperforming some state-of-the-art fully supervised models. Also, our framework can be utilized to fine-tune models trained on natural image segmentation datasets drastically improving their performance for polyp segmentation and impressively demonstrating superior performance to fully supervised fine-tuning. Code can be found in https://github.com/ic-qialanqian/WS-DefSegNet.
更多查看译文
关键词
automated polyp segmentation,colorectal cancer,computer-aided diagnosis,deformable transformers,fully supervised fine-tuning,natural image segmentation datasets,pixel-wise annotated datasets,semisupervised learning,unlabeled images,weakly annotated images,weakly-supervised training
AI 理解论文
溯源树
样例
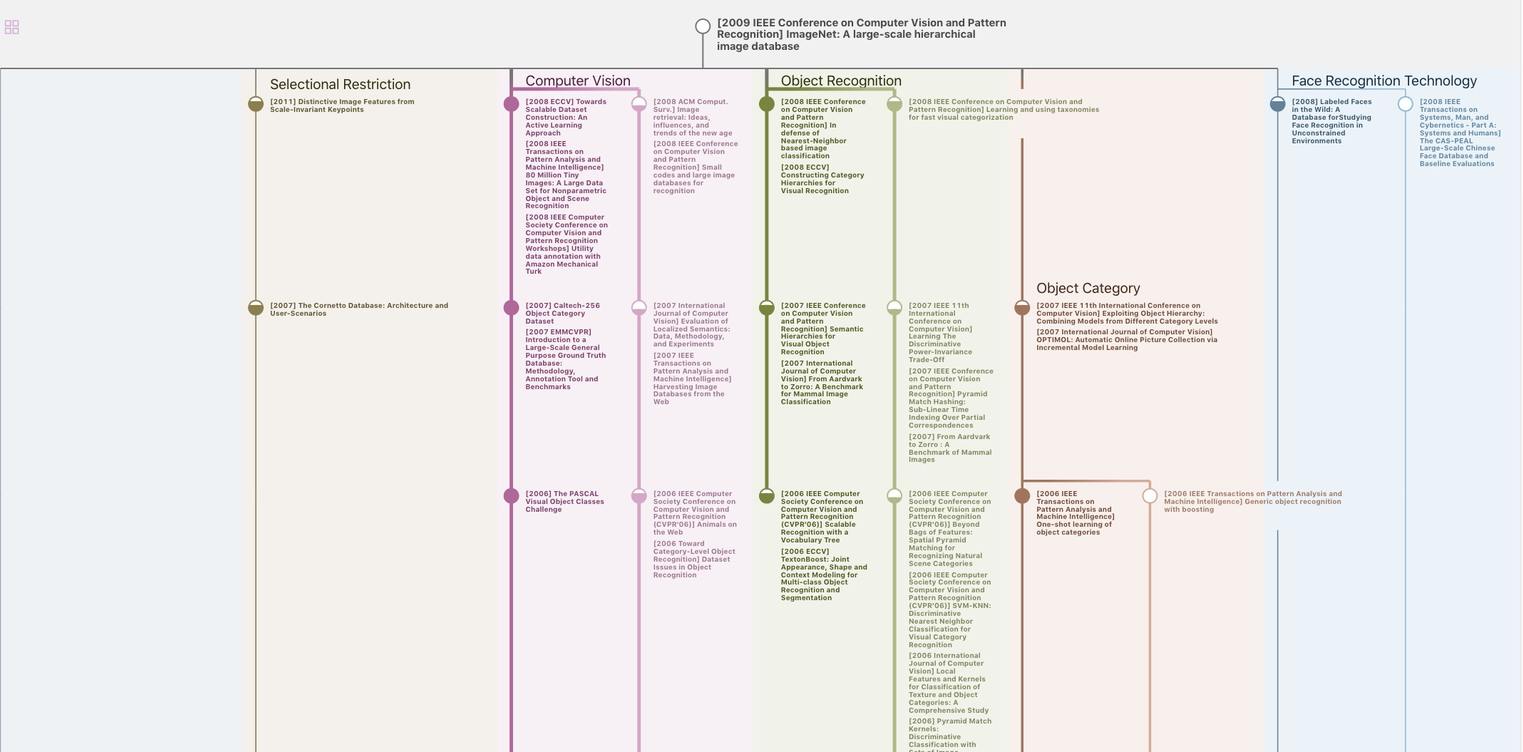
生成溯源树,研究论文发展脉络
Chat Paper
正在生成论文摘要