Adversarial Stimuli: Attacking Brain-Computer Interfaces via Perturbed Sensory Events
arxiv(2022)
摘要
Machine learning models are known to be vulnerable to adversarial perturbations in the input domain, causing incorrect predictions. Inspired by this phenomenon, we explore the feasibility of manipulating EEG-based Motor Imagery (MI) Brain Computer Interfaces (BCIs) via perturbations in sensory stimuli. Similar to adversarial examples, these \emph{adversarial stimuli} aim to exploit the limitations of the integrated brain-sensor-processing components of the BCI system in handling shifts in participants' response to changes in sensory stimuli. This paper proposes adversarial stimuli as an attack vector against BCIs, and reports the findings of preliminary experiments on the impact of visual adversarial stimuli on the integrity of EEG-based MI BCIs. Our findings suggest that minor adversarial stimuli can significantly deteriorate the performance of MI BCIs across all participants (p=0.0003). Additionally, our results indicate that such attacks are more effective in conditions with induced stress.
更多查看译文
关键词
Machine Learning,Machine Learning Models,Visual Stimuli,Sensory Stimuli,Alpha Band,Directions For Further Research,Motor Imagery,Adversarial Attacks,Adversarial Examples,Stimuli In The Form,Brain-computer Interface System,Impact Of Stimuli,Motor Imagery Tasks,Adversarial Perturbations,Attack Vector,Null Hypothesis,Data Privacy,Wheelchair,Effects Of Stimuli,Error-related Negativity,Hardware Components,Environmental Observations,Malicious Activities,Mu Rhythm,Types Of Attacks,CNN Classifier,Attack Surface,Brain-computer Interface Technology,Band Power
AI 理解论文
溯源树
样例
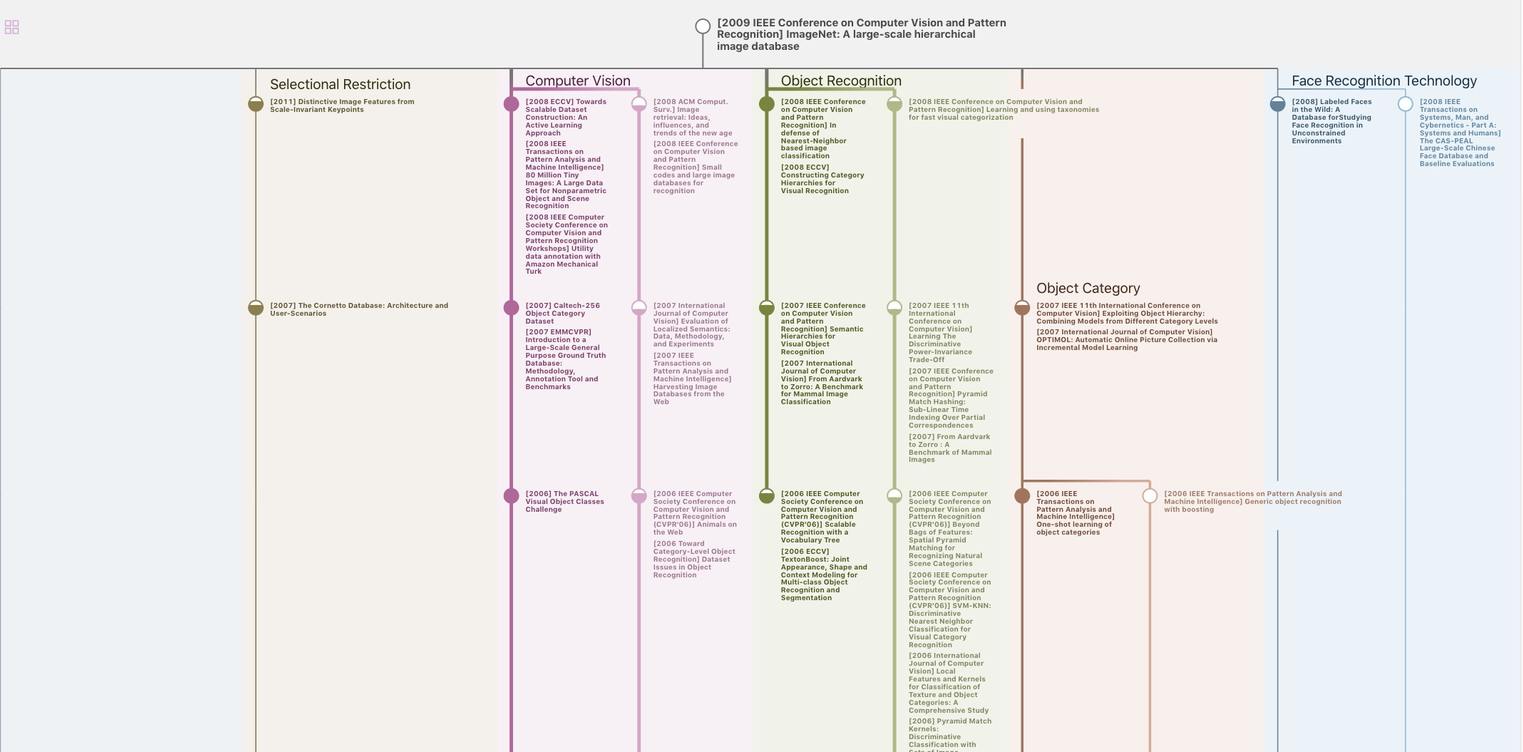
生成溯源树,研究论文发展脉络
Chat Paper
正在生成论文摘要