Incorporating anticipation embedding into reinforcement learning framework for multi-hop knowledge graph question answering
Information Sciences(2022)
摘要
Multi-hop knowledge graph question answering (KGQA) aims to pinpoint answer entities by reasoning across multiple triples in knowledge graphs (KGs). To enhance model interpretability, reinforcement learning (RL) based methods are proposed. However, RL-based solutions suffer from two major issues. The first is aimless exploration due to the limited observation information. The second is delayed and sparse rewards caused by weak supervision. To bridge the gap, we propose an interpretable model based on RL for multi-hop KGQA with weak supervision, which we refer to as Anticipation Reasoning Network (ARN) that incorporates KG embeddings as anticipation information into RL framework to avoid aimlessly traversing in KG. ARN achieves the goal via three steps. (1) KG embedding models represent the entities and relations as low-dimensional vectors. (2) We propose a knowledge embedding based question answering (KEQA) framework to measure the plausibility of the “triple” in embedding space. (3) A policy-guided agent is designed to perform effective path reasoning by selecting the most promising action at each step. Moreover, we utilize reward shaping strategy and actor-critic optimization to alleviate the problem of low-quality rewards. Extensive experiments well demonstrate the effectiveness of our method footnoteFor reproducibility, we publicly release the code at https://github.com/iDylanCui/ARN.
更多查看译文
关键词
Knowledge graph,Question answering,Reinforcement learning,Sparse rewards,Weak supervision
AI 理解论文
溯源树
样例
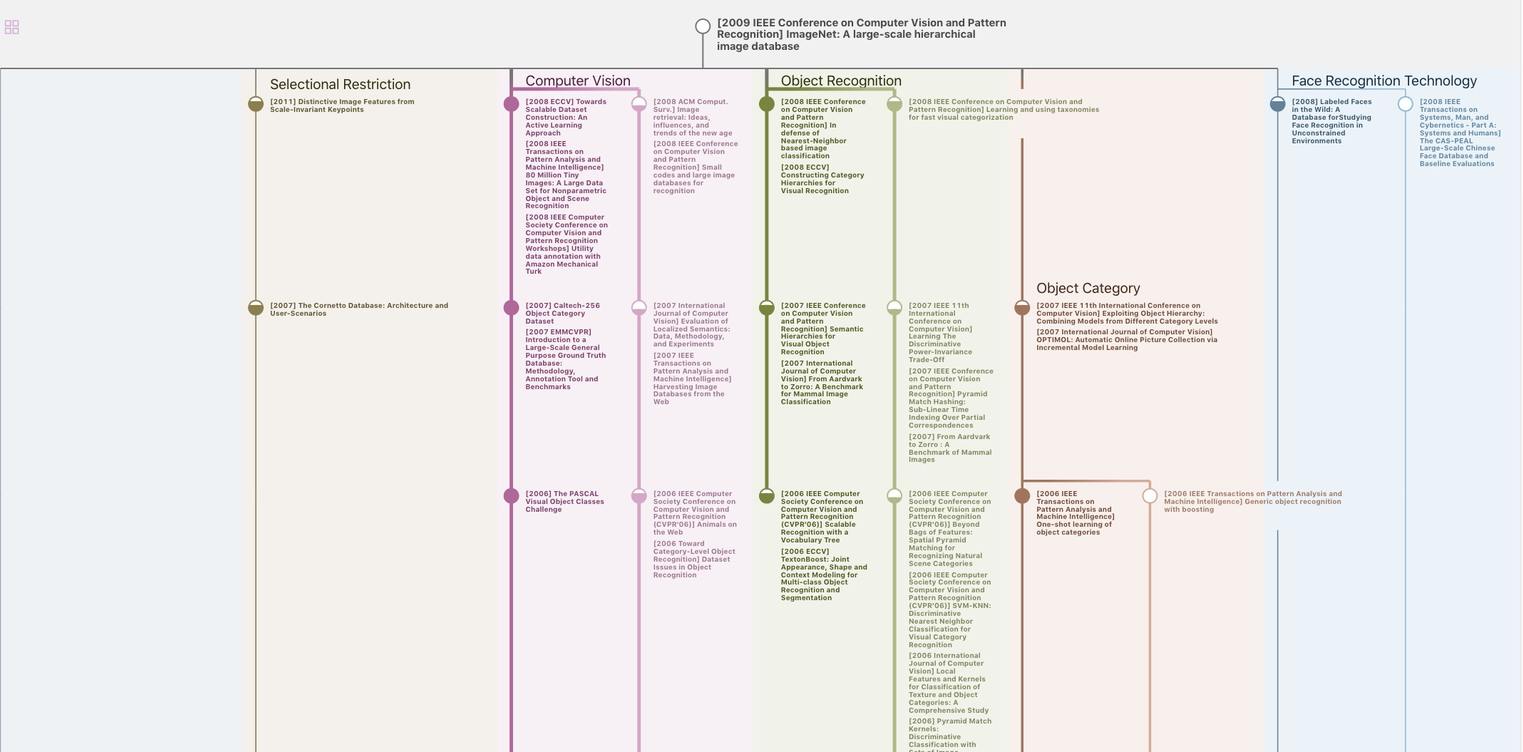
生成溯源树,研究论文发展脉络
Chat Paper
正在生成论文摘要