A systematic data-driven approach for production forecasting of coalbed methane incorporating deep learning and ensemble learning adapted to complex production patterns
Energy(2023)
摘要
Coalbed methane(CBM) as an essential component of clean energy is of strategic significance to global sustainable development, with its production forecast being the basis for reservoirs development. However, due to complex mass transfer mechanism under different geological conditions, traditional physics-based methods are difficult to cope with the complex and various CBM production behavior. Considering the actual production characteristics, this study innovatively combines supervised learning and unsupervised learning to develop an autonomous data governance system based on Local Outlier Factor(LOF) and eXtreme Gradient Boosting (Xgboost), which can perform anomaly detection of dynamic CBM data as well as missing value supplement. Additionally, a data-driven production forecasting model is designed incorporating a Bi-directional Long-Short-Term-Memory(Bi-LSTM) to tackle the various production curve patterns of CBM wells. The production curves from 782 wells of real CBM reservoirs were classified into I, II, III, and IV patterns in terms of their characteristics, with percentages of 32%, 29%, 20%, and 19% respectively. The results reveal that the LOF-Xgboost governance system not only enhances the short-term prediction performance but also significantly improves the long-term prediction robustness. The improved Bi-LSTM-based production model of CBM shows more powerful adaptability and accuracy for both conventional curve patterns and irregular production behavior.
更多查看译文
关键词
Coalbed methane,Production forecast,Deep learning,Local outlier factor,Bi-LSTM,Xgboost
AI 理解论文
溯源树
样例
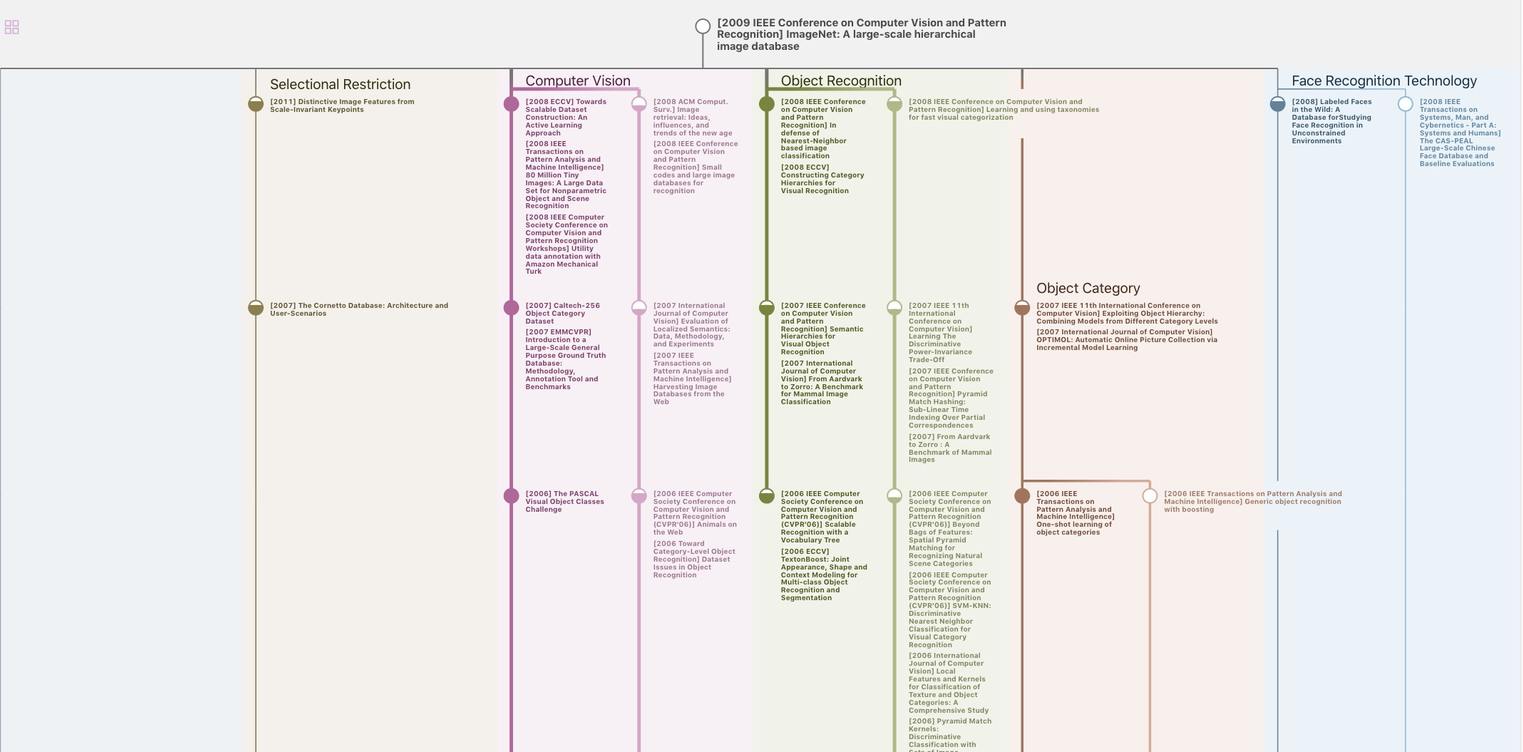
生成溯源树,研究论文发展脉络
Chat Paper
正在生成论文摘要