Interpreting the antecedents of a predicted output by capturing the interdependencies among the system features and their evolution over time
Engineering Applications of Artificial Intelligence(2023)
摘要
Decision support systems (DSS) assist in a wide array of decision-making tasks in different domains. However, one of their common drawbacks is that their working is back box in nature. This means that while they recommend a decision, they cannot explain the ’why’ behind reaching that decision. In prescriptive tasks such as risk management, this does not assist the risk manager in identifying the contributing features leading to the occurrence of a risk output against which corrective action/s needs to be taken. This limitation has sparked interest in explainability, where glass-box methods interpret the contributing features leading to the recommended decision. Approaches which do that however do not model how the contributing features’ evolve over a period of time, till the predicted time period, to determine the output class before interpreting the reason for the decision output. To address these gaps, in this work we propose An Automated Interpretable Artificial Intelligence framework for Proactive Risk Management (AIAI-PRM). AIAI-PRM augments Local Interpretation-Driven Abstract Bayesian Network (LINDA-BN) with Knowledge Graph to determine the inter dependencies among the features, model how they evolve over a period of time and interpret the contributing features leading to the recommended output. In the domain of risk management, we show how this knowledge can be used by the risk manager to determine those key features against which risk management strategies need to be developed. Finally, we compare the AIAI-PRM’s output with that of the most commonly used XAI approaches, namely LIME and SHAP, to prove its superiority.
更多查看译文
关键词
Black-box,Explainable AI (XAI),Glass-box,Interpretable models
AI 理解论文
溯源树
样例
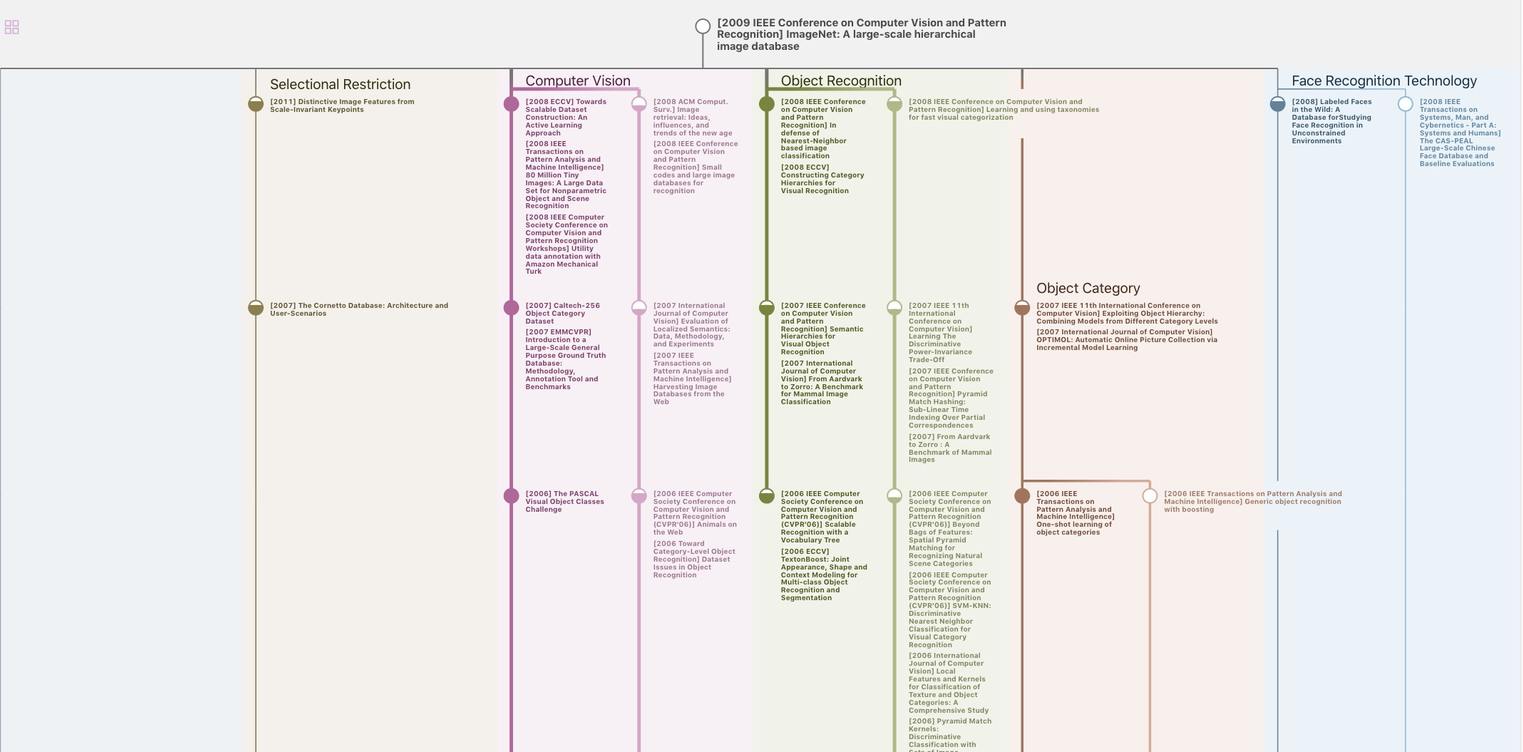
生成溯源树,研究论文发展脉络
Chat Paper
正在生成论文摘要