Simulated Mental Imagery for Robotic Task Planning
arxiv(2022)
摘要
Traditional AI-planning methods for task planning in robotics require symbolically encoded domain description. While powerful in well-defined scenarios, setting this up requires substantial effort. Different from this, most everyday planning tasks are solved by humans intuitively, using mental imagery of the different planning steps. Here we suggest that the same approach can be used for robots, too, in cases which require only limited execution accuracy. In the current study, we propose a novel sub-symbolic method called Simulated Mental Imagery for Planning (SiMIP), which consists of several steps: perception, simulated action, success-checking and re-planning performed on 'imagined' images. We show that it is possible this way to implement mental imagery-based planning in an algorithmically sound way by combining regular convolutional neural networks and generative adversarial networks. With this method, the robot acquires the capability to use the initially existing scene to generate action plans without symbolic domain descriptions, hence, without the need to define an explicit representation of the environment. We create a dataset from real scenes for a packing problem of having to correctly place different objects into different target slots. This way efficiency and success rate of this algorithm could be quantified.
更多查看译文
关键词
mental imagery, deep learning, robotic planning, artificial neural network, human-interpretable
AI 理解论文
溯源树
样例
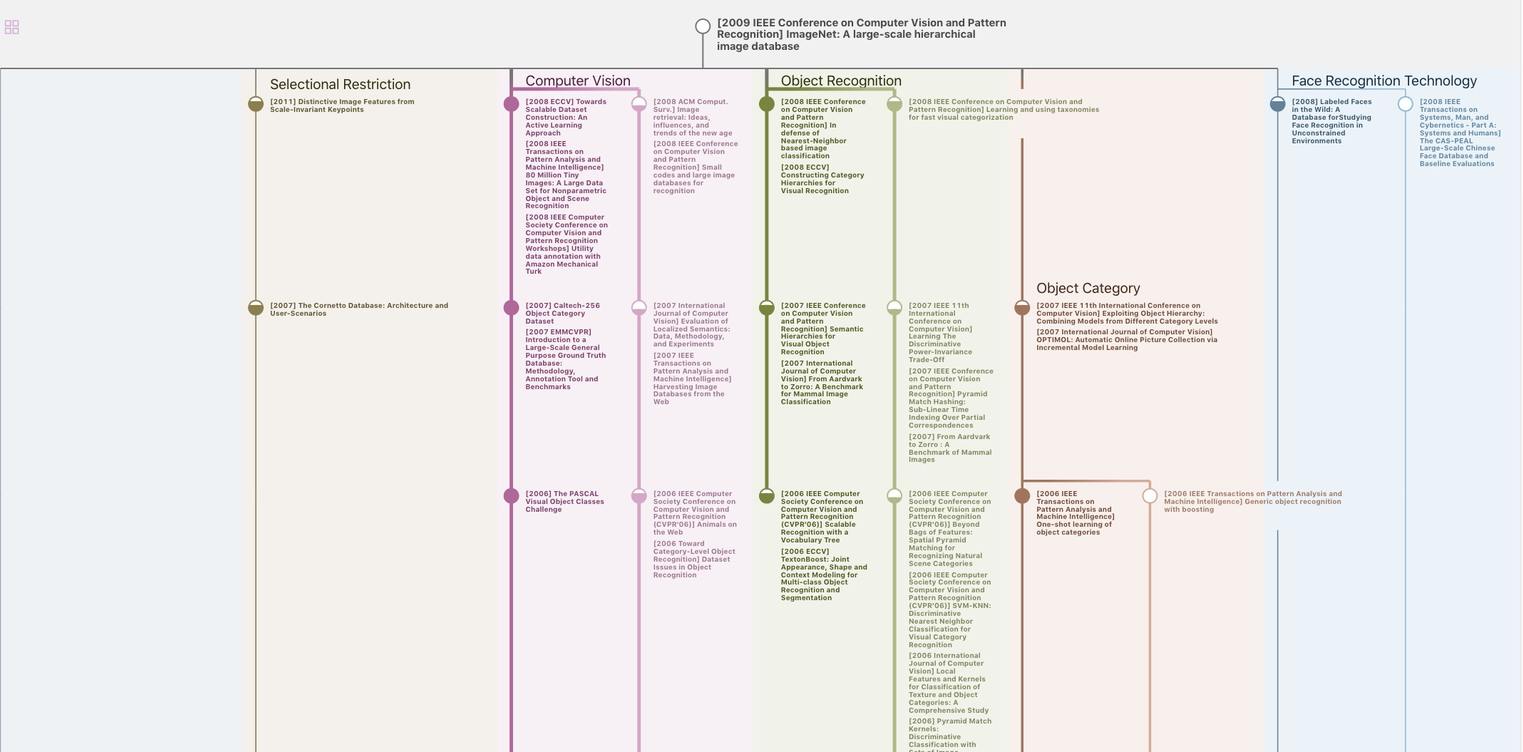
生成溯源树,研究论文发展脉络
Chat Paper
正在生成论文摘要