Datasets and Methods for Boosting Infrastructure Inspection: A Survey on Defect Segmentation and Detection
2022 IEEE 17th International Conference on Control & Automation (ICCA)(2022)
摘要
The lack of publicly available defect datasets with qualified annotations has restricted the development of deep learning-based infrastructure defects inspection. This two-part survey aims to boost research in deep learning-based defects inspection. In the companion paper of this work, we have presented a systematic survey on 11 classification-oriented defect datasets and comprehensively compared the state-of-the-art algorithms for object classification, taking crack as our research interest. Besides, we have summarized the datasets before and incorporated our self-labeled crack data to establish a benchmark dataset and proposed suggestions for building a high-quality dataset for defects classification. In this paper, we first present a systematic survey on 26 publicly available datasets for defect segmentation and detection. We then conduct experiments on the established defect dataset for the crack segmentation and the subsequent detection tasks based on non-maximum suppression. The results show that our proposed method, based on our previous work, has achieved satisfactory performance compared with the state of the arts. Our method also shows superior performance with acceptable efficiency on modern graphic processing units. Moreover, we have also performed a detailed illustration of the typical segmentation networks and a comprehensive comparison between existing state-of-the-art algorithms for crack segmentation, which provides a comprehensive baseline for future research in defects inspection.
更多查看译文
关键词
infrastructure inspection,defect segmentation,publicly available defect datasets,object classification,crack data,benchmark dataset,high-quality dataset,crack segmentation,deep learning-based infrastructure defect inspection,classification-oriented defect datasets,nonmaximum suppression,graphic processing unit,defect detection
AI 理解论文
溯源树
样例
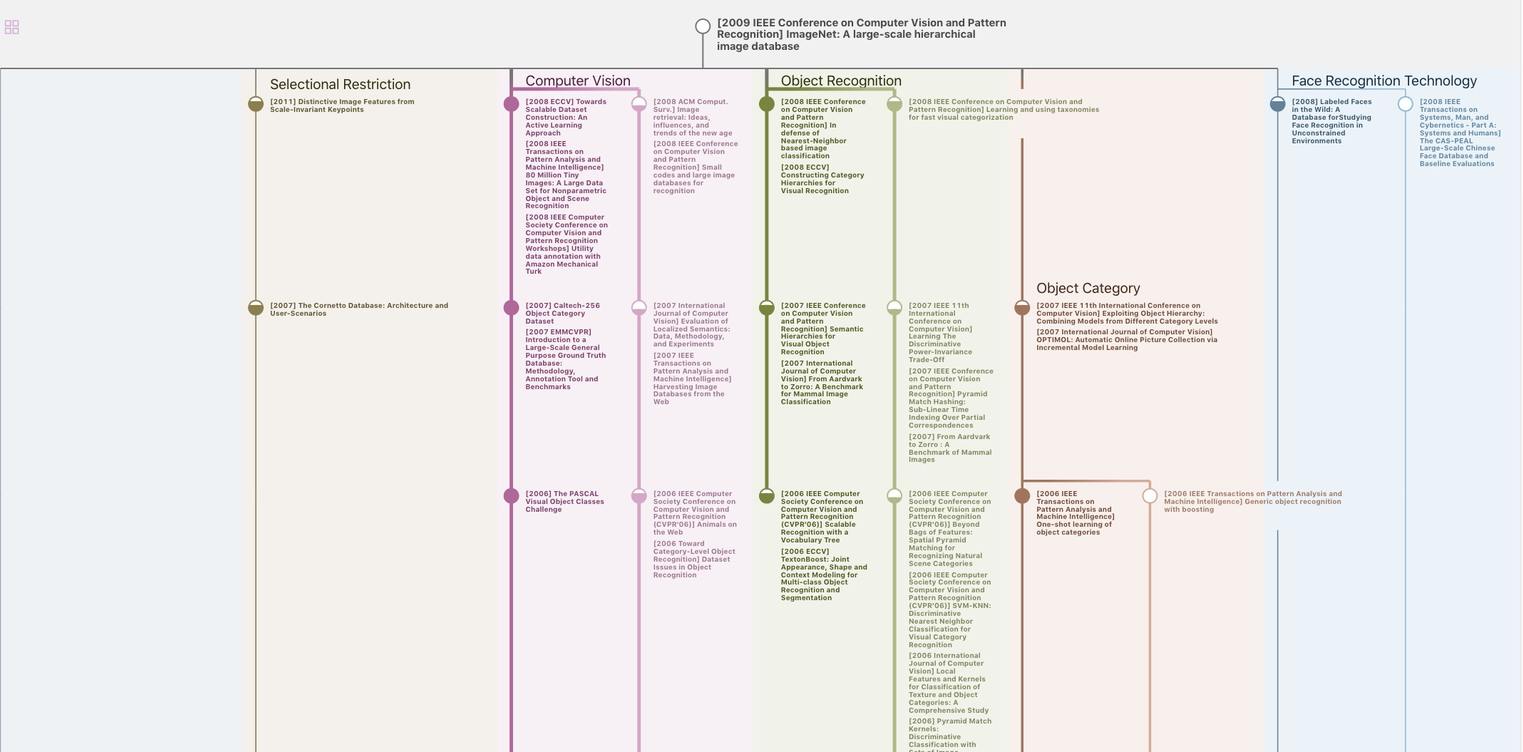
生成溯源树,研究论文发展脉络
Chat Paper
正在生成论文摘要