Evolving Lockdown Strategies to Minimize Infections in an Epidemic
2022 IEEE Conference on Computational Intelligence in Bioinformatics and Computational Biology (CIBCB)(2022)
摘要
In this paper we evaluate the impact of different lockdown strategies upon the total number of infections during an epidemic. The strategies are based upon the percentage of the population infected during a given time step, as well as upon the amount by which interactions must be reduced during lockdown. We use a weighted personal contact network to represent the population, its interactions, and the relative strengths of those interactions. During lockdown edges from this network are removed. We use an evolutionary algorithm to choose the set of edges to be removed so as to minimize infections, comparing different strategies. We show that allowing the evolutionary algorithm to choose which edges to remove significantly reduces the overall number of infections in comparison to random selection. In fact, the EA results for the least stringent conditions were similar or better to the random results for the most stringent conditions, showing that a judicious choice of restrictions during lockdown has the greatest effect on reducing infections. The evolutionary algorithm tends to favour a situation in which during lockdown individuals would reduce their number of contacts, as opposed to lessening the strength of their connections.
更多查看译文
关键词
stringent conditions,evolutionary algorithm,lockdown individuals,infections,epidemic,lockdown strategies,weighted personal contact network,lockdown edges
AI 理解论文
溯源树
样例
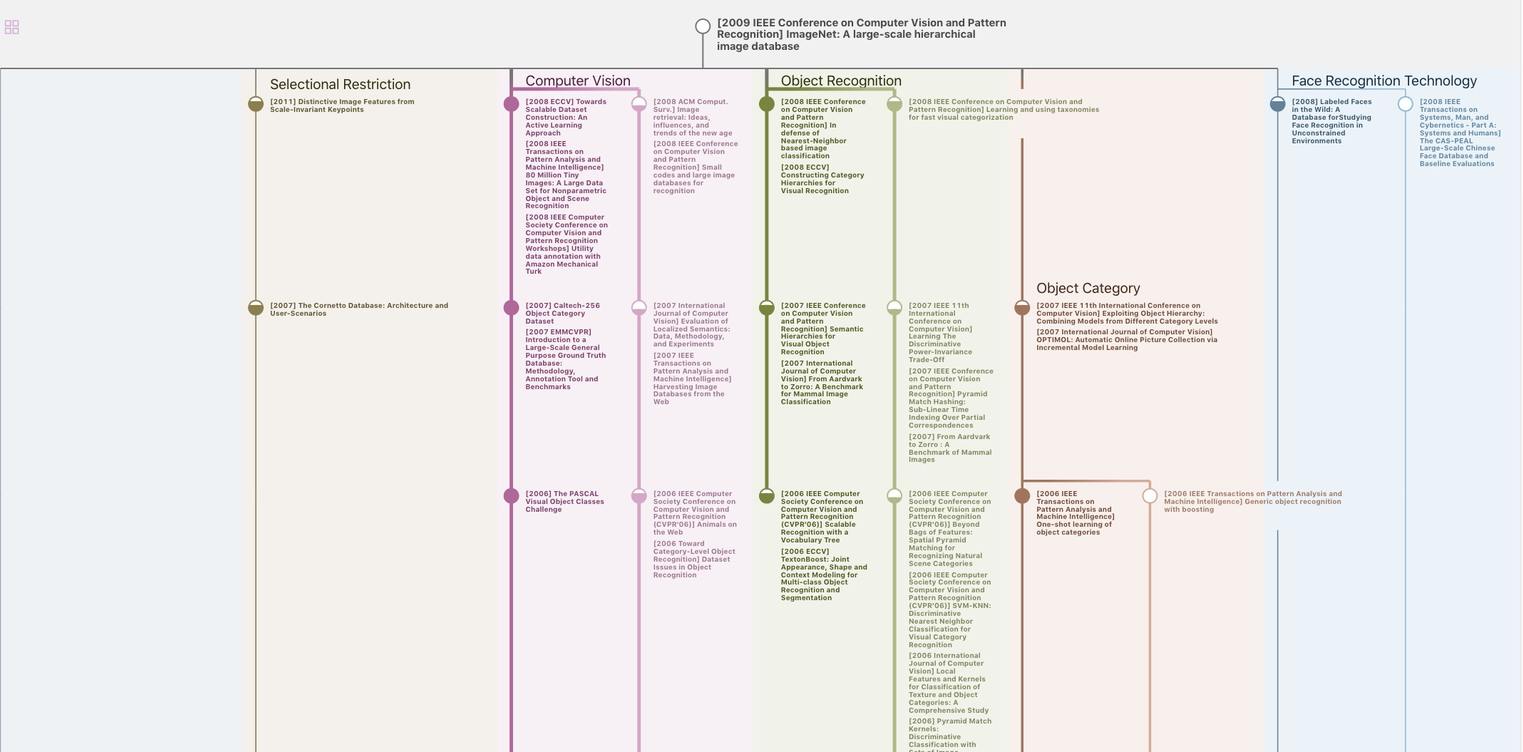
生成溯源树,研究论文发展脉络
Chat Paper
正在生成论文摘要