Disentangled Bad Weather Removal GAN for Pedestrian Detection
2022 IEEE 95TH VEHICULAR TECHNOLOGY CONFERENCE (VTC2022-SPRING)(2022)
Abstract
Bad weather such as rain and haze will lower the visibility and contrast of captured images and often occur at the same time, which makes the situation worse. Existing CNN-based methods can achieve impressive results when processing each condition individually. But few works consider removing rain and haze under a unified framework. Besides, these models will experience degraded performance when the distribution of the data changes. In this work, we attempt to find a method that can remove all bad weather without switching between single task models and paired training data for driving scene applications like pedestrian detection. In specific, we adopt a disentanglement strategy to obtain weather layer from subtraction. The statistic distance is calculated between two weather layers from each pipeline. In addition, the weather layer serves as information guidance and input to the reconstruct generator. Experiments on mixed dataset from RainCityscapes and Foggy Cityscapes show that the effectiveness of pedestrian detector is improved. Finally, we contribute a new dataset called Realistic Driving Scene under Bad Weather (RDSBW), which contains over 70K real bad weather images.
MoreTranslated text
Key words
Bad Weather Removal, Unpaired Image-to-Image Translation, Disentangled Representations, Pedestrian Detection
AI Read Science
Must-Reading Tree
Example
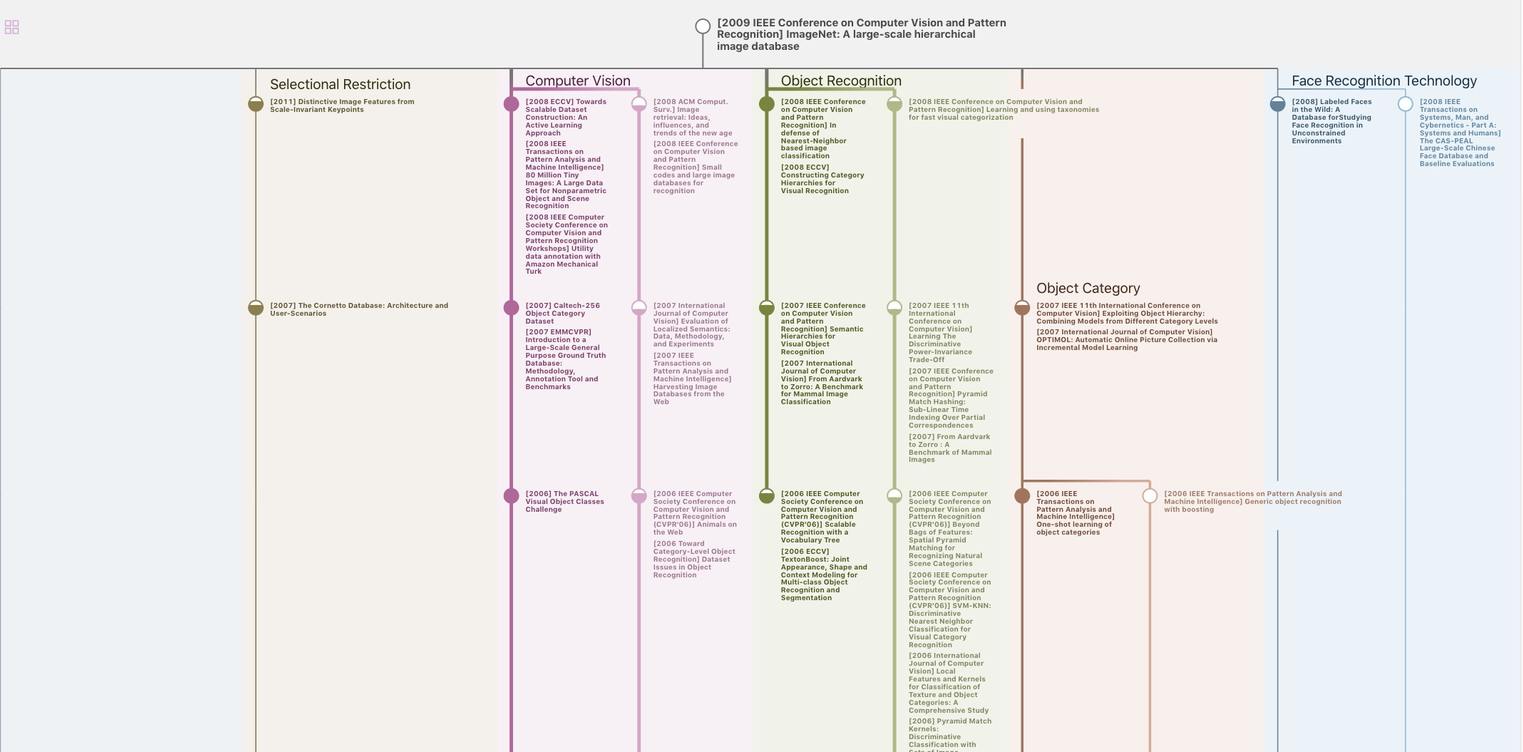
Generate MRT to find the research sequence of this paper
Chat Paper
Summary is being generated by the instructions you defined