Memristor-Based In-Circuit Computation for Trace-Based STDP
2022 IEEE 4th International Conference on Artificial Intelligence Circuits and Systems (AICAS)(2022)
摘要
Recently, memristors have been widely used to implement Spiking Neural Networks (SNNs), which is promising in edge computing scenarios. However, most memristor-based SNN implementations adopt simplified spike-timing-dependent plasticity (STDP) for the online learning process. It is challenging for memristor-based implementations to support the trace-based STDP learning rules that have been widely used in neuromorphic applications. This paper proposed a versatile memristor-based architecture to implement the synaptic-level trace-based STDP learning rules. Especially, the similarity between synaptic trace dynamics and the memristor nonlinearity is explored and exploited to emulate the trace variables of trace-based STDP. As two typical trace-based STDP learning rules, the pairwise STDP and the triplet STDP, are simulated on two typical nonlinear bipolar memristor devices. The simulation results show that the behavior of physical memristor devices can be well estimated (below 6% in terms of the relative root-mean-square error), and the memristor-based in-circuit computation for trace-based STDP learning rules can achieve a high correlation coefficient over 98%.
更多查看译文
关键词
Memristor,trace,spike-timing-dependent plasticity (STDP),online learning,spiking neural network (SNN),neuromorphic computation
AI 理解论文
溯源树
样例
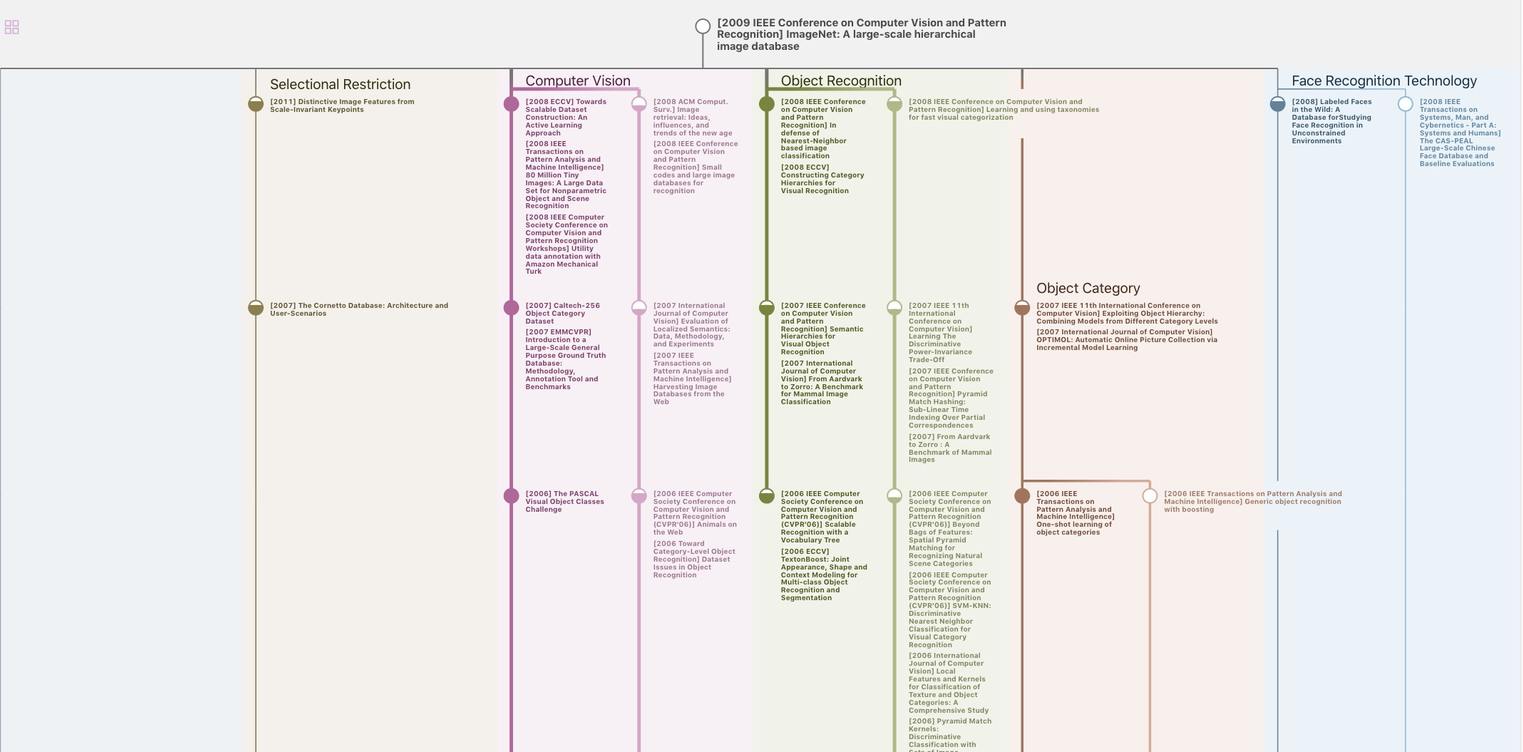
生成溯源树,研究论文发展脉络
Chat Paper
正在生成论文摘要