SUNDEW: An Ensemble of Predictors for Case-Sensitive Detection of Malware
arxiv(2022)
摘要
Malware programs are diverse, with varying objectives, functionalities, and threat levels ranging from mere pop-ups to financial losses. Consequently, their run-time footprints across the system differ, impacting the optimal data source (Network, Operating system (OS), Hardware) and features that are instrumental to malware detection. Further, the variations in threat levels of malware classes affect the user requirements for detection. Thus, the optimal tuple of is different for each malware class, impacting the state-of-the-art detection solutions that are agnostic to these subtle differences. This paper presents SUNDEW, a framework to detect malware classes using their optimal tuple of . SUNDEW uses an ensemble of specialized predictors, each trained with a particular data source (network, OS, and hardware) and tuned for features and requirements of a specific class. While the specialized ensemble with a holistic view across the system improves detection, aggregating the independent conflicting inferences from the different predictors is challenging. SUNDEW resolves such conflicts with a hierarchical aggregation considering the threat-level, noise in the data sources, and prior domain knowledge. We evaluate SUNDEW on a real-world dataset of over 10,000 malware samples from 8 classes. It achieves an F1-Score of one for most classes, with an average of 0.93 and a limited performance overhead of 1.5%.
更多查看译文
关键词
malware,predictors,ensemble,detection,case-sensitive
AI 理解论文
溯源树
样例
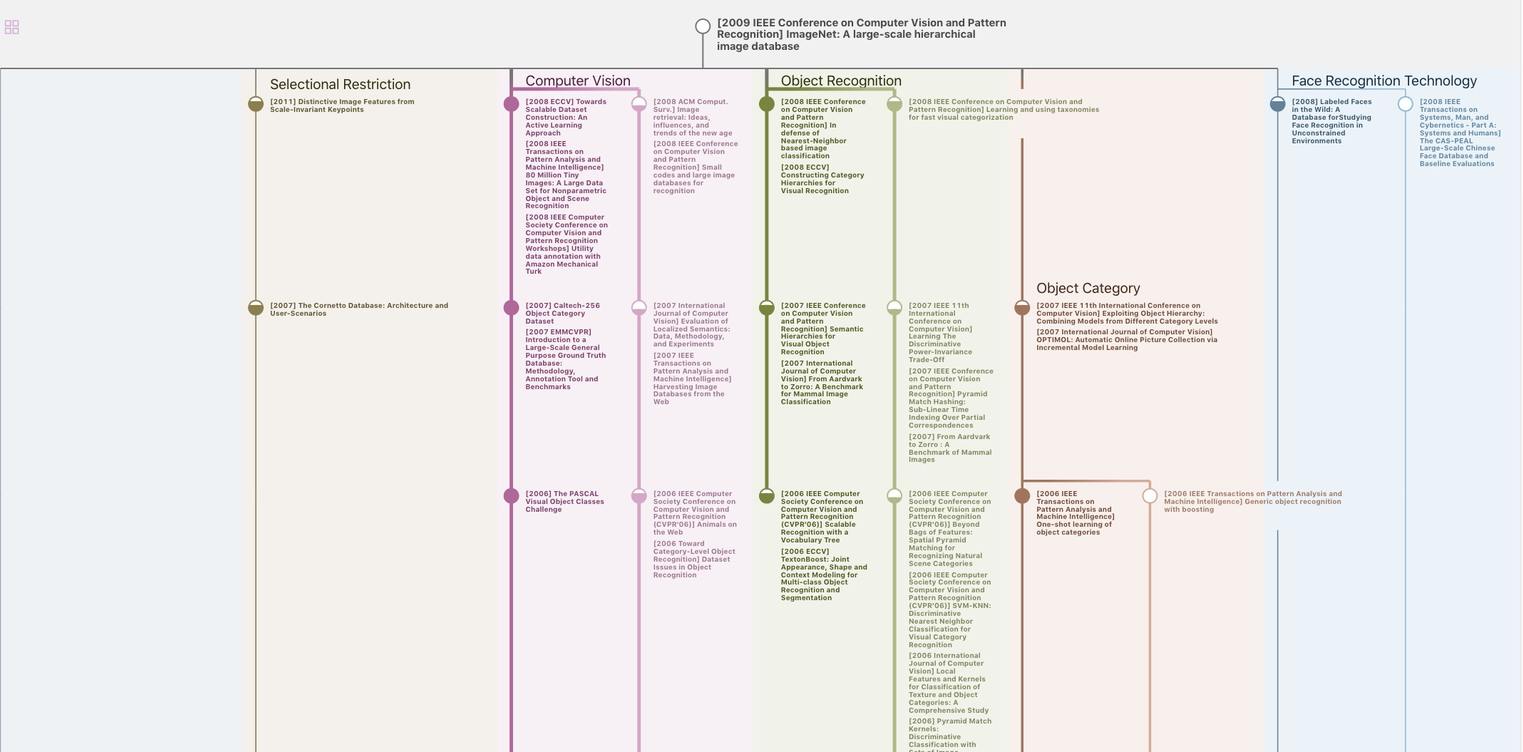
生成溯源树,研究论文发展脉络
Chat Paper
正在生成论文摘要