Vaguely quantified fuzzy dominance rough set and its incremental maintenance approximation approaches
SOFT COMPUTING(2022)
摘要
Dominance-based rough set approach (DRSA) is an effective knowledge acquisition tool for ordered data, and to meet the needs of practical applications, the DRSA-based extended models have been widely studied. Nevertheless, the collected data in practical applications are always polluted by noise and most of these extended models are very susceptible to noise. In addition, with the arrival of new attributes and the removal of obsolete attributes, the ordered data have the characteristic of attributes evolving over time ( i . e ., dynamic ordered data). How to efficiently mining knowledge from dynamic ordered data is a meaningful topic. Motivated by these two issues, we first propose a robust fuzzy dominance rough set model to combat noise interference and then develop incremental update approximation mechanisms based on the proposed model for dynamic ordered data. Firstly, the vaguely quantified fuzzy dominance rough set (VQFDRS) and its matrix representation are introduced. Subsequently, we develop the incremental mechanisms to update the approximations of VQFDRS when multiple attributes are added to or deleted from an ordered data, respectively. Then, on this basis, a static calculation approximations algorithm and two incremental calculation approximations algorithms are designed, respectively. Finally, a series of numerical comparative experiments are performed on six public datasets. Experimental results indicate that the VQFDRS has good robustness, and the incremental algorithms are highly efficient compared with static algorithm under the dynamic data environment.
更多查看译文
关键词
Robust fuzzy dominance rough set,Incremental learning,Matrix representation,Dynamic ordered data
AI 理解论文
溯源树
样例
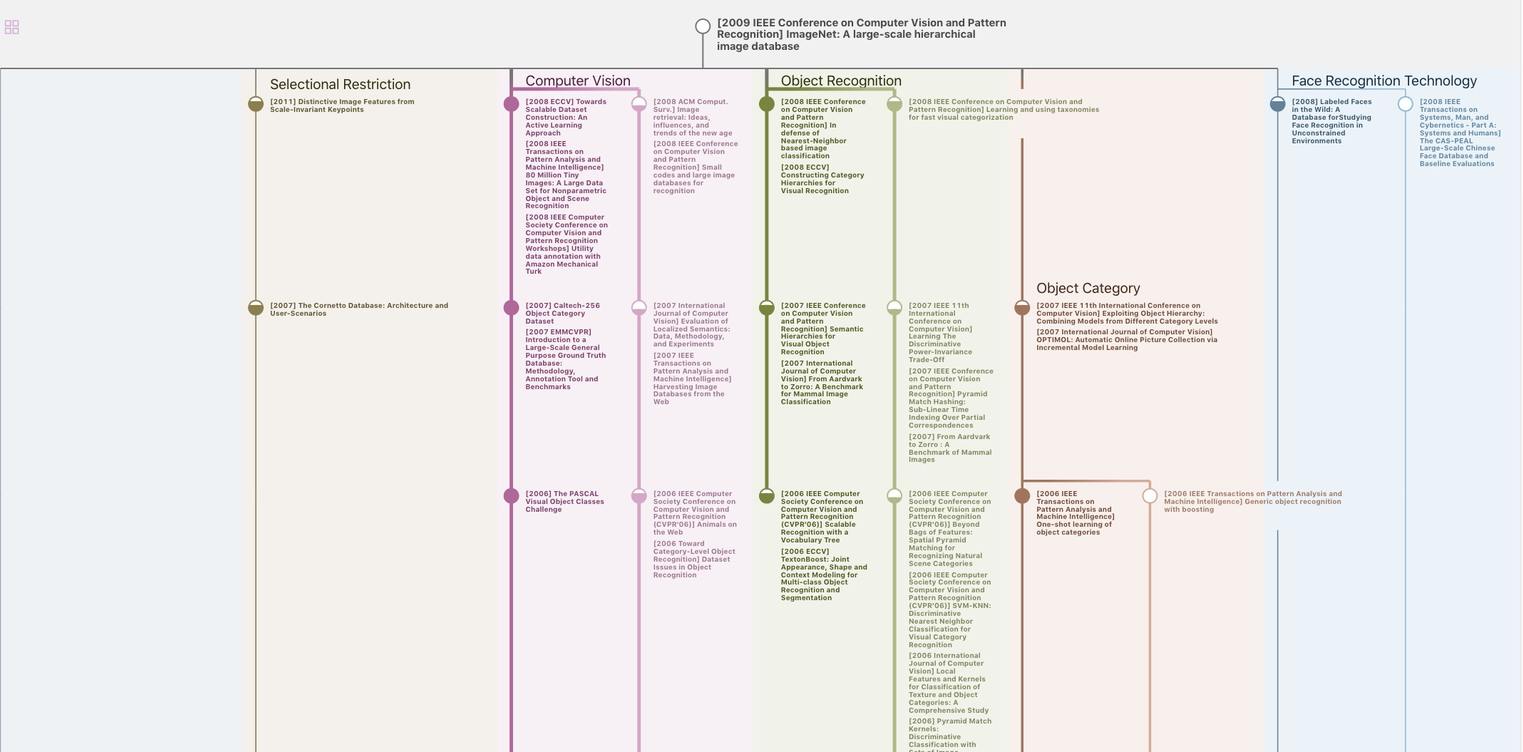
生成溯源树,研究论文发展脉络
Chat Paper
正在生成论文摘要