Detection of Atrial Fibrillation From Variable-Duration ECG Signal Based on Time-Adaptive Densely Network and Feature Enhancement Strategy
IEEE Journal of Biomedical and Health Informatics(2023)
摘要
Atrial fibrillation (AF) is one of the clinic's most common arrhythmias with high morbidity and mortality. Developing an intelligent auxiliary diagnostic model of AF based on a body surface electrocardiogram (ECG) is necessary. Convolutional neural network (CNN) is one of the most commonly used models for AF recognition. However, typical CNN is not compatible with variable-duration ECG, so it is hard to demonstrate its universality and generalization in practical applications. Hence, this paper proposes a novel Time-adaptive densely network named MP-DLNet-F. The MP-DLNet module solves the problem of incompatibility between variable-duration ECG and 1D-CNN. In addition, the feature enhancement module and data imbalance processing module are respectively used to enhance the perception of temporal-quality information and decrease the sensitivity to data imbalance. The experimental results indicate that the proposed MP-DLNet-F achieved 87.98% classification accuracy, and F1-score of 0.847 on the CinC2017 database for 10-second cropped/padded single-lead ECG fragments. Furthermore, we deploy transfer learning techniques to test heterogeneous datasets, and in the CPSC2018 12-lead dataset, the method improved the average accuracy and F1-score by 21.81% and 16.14%, respectively. Experimental results indicate that our method can update the constructed model's parameters and precisely forecast AF with different duration distributions and lead distributions. Combining these advantages, MP-DLNet-F can exemplify all kinds of varied-duration or imbalance medical signal processing problems such as Electroencephalogram (EEG) and Photoplethysmography (PPG).
更多查看译文
关键词
Atrial fibrillation,feature enhancement,time-adaptive densely network,variable-duration ECG
AI 理解论文
溯源树
样例
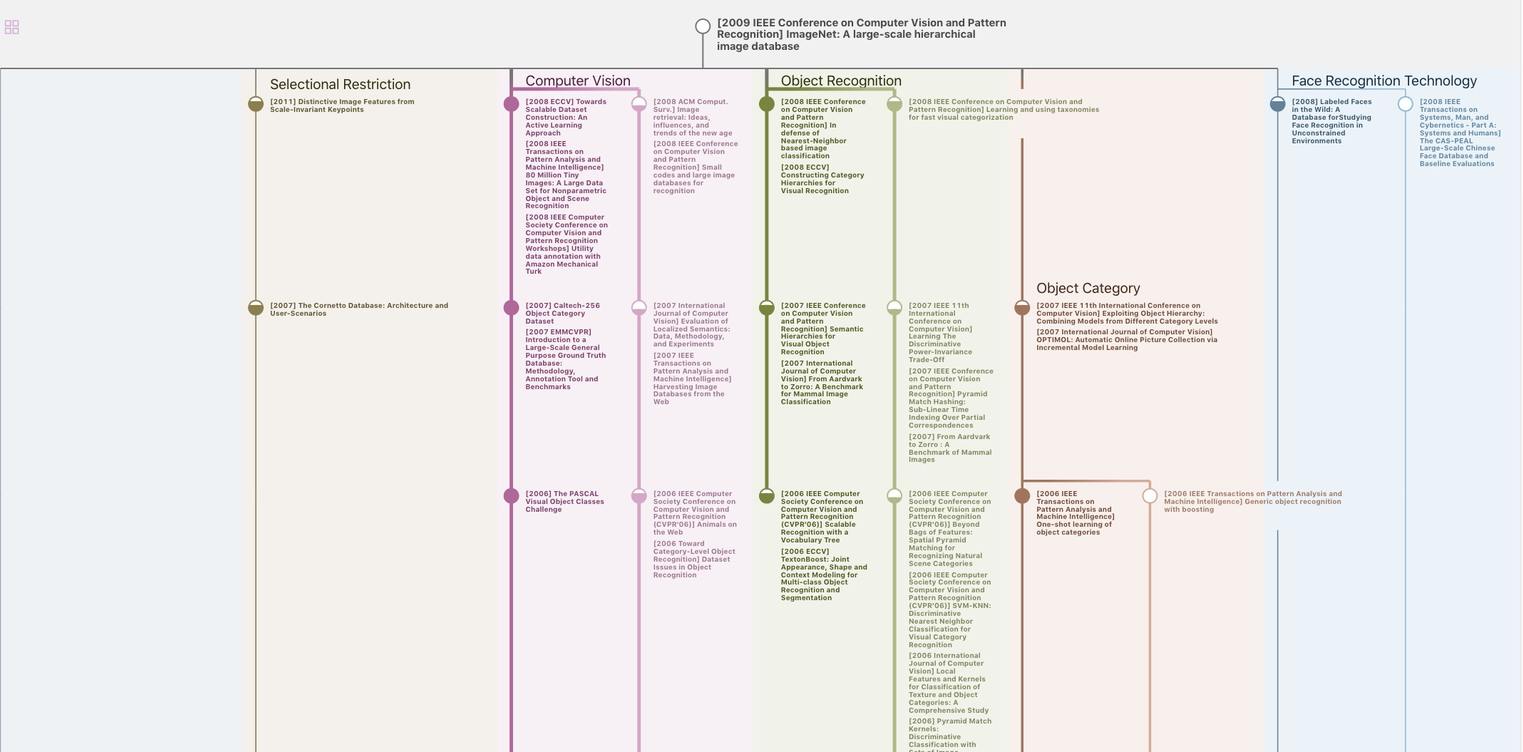
生成溯源树,研究论文发展脉络
Chat Paper
正在生成论文摘要