Weakly Supervised Instance Segmentation via Category-aware Centerness Learning with Localization Supervision
PATTERN RECOGNITION(2022)
摘要
Deep convolutional neural networks (DCNN) trained with pixel-level segmentation masks achieve high performance in the task of instance segmentation. The difficulty of acquiring such annotation limits the application and popularization of the DCNN-based approaches. To address the issue, a weakly supervised approach is proposed in the paper which performs instance segmentation only with the supervision of bounding box or coarse localization annotation. A novel DCNN model is constructed which consists of two branches: the centerness branch and the segmentation branch. The former branch is to learn the semantically spatial importance over the areas of object instances under the localization supervision. Ob-ject proposals with exact boundaries are automatically generated and are then ranked under the guidance of the output of the centerness branch. The most matched instance proposal is assigned to each object, which is then used to supervise the segmentation branch. The losses are calculated by both the outputs of the two branches and the entire DCNN model is trained end-to-end. Experiments are extensively con-ducted to verify the effectiveness. With the supervision of precise bounding box annotation, our approach achieves state-of-the-art (SOTA) accuracy in the comparison with recent related works. And in the case of coarse localization annotation, our approach only deduces a slight reduction in accuracy, which signif-icantly outperforms other approaches. The excellent performance demonstrates that our approach would be helpful to further alleviate the workload of image annotation while maintaining competitive accuracy.(c) 2022 Elsevier Ltd. All rights reserved.
更多查看译文
关键词
Weakly supervised learning,Instance segmentation,Centerness,Coarse localization annotation
AI 理解论文
溯源树
样例
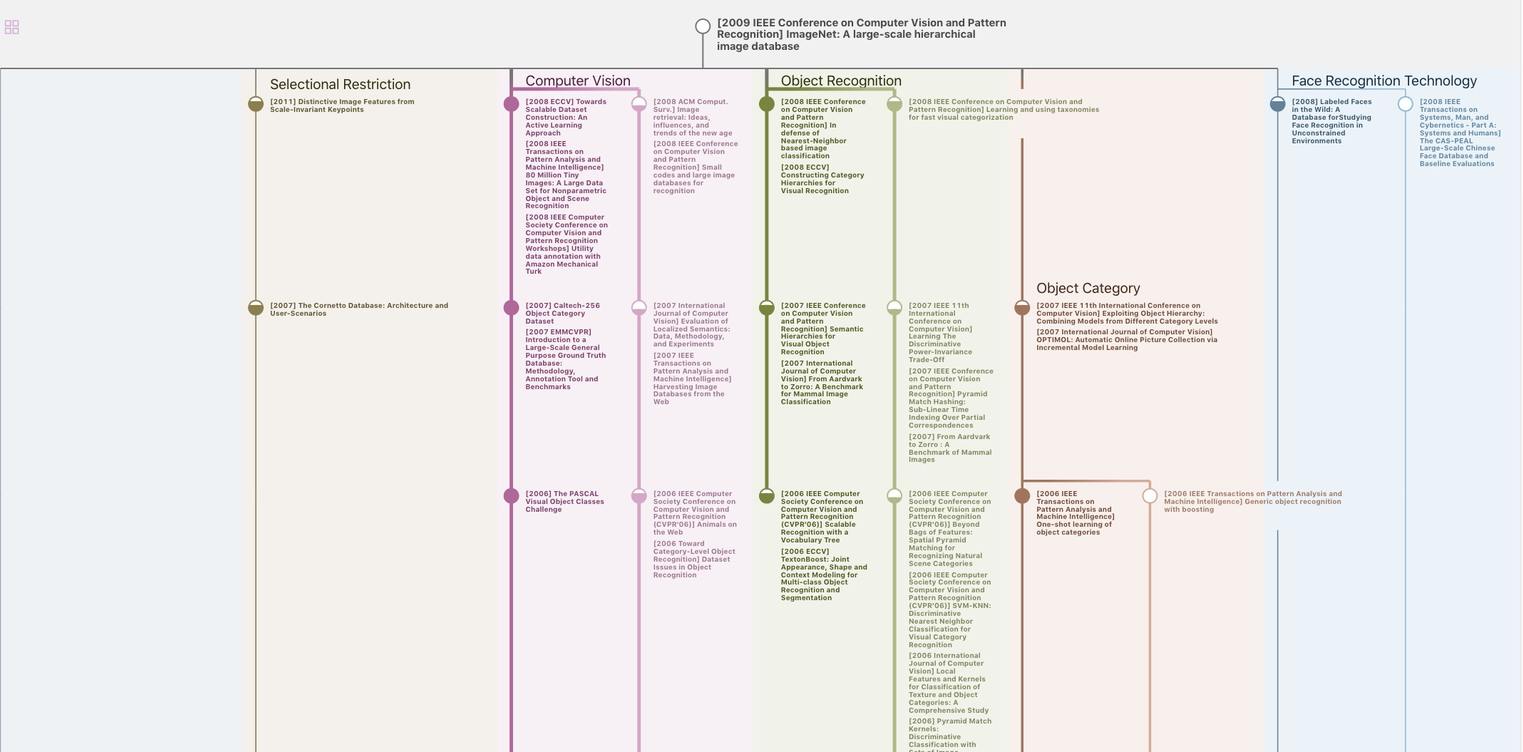
生成溯源树,研究论文发展脉络
Chat Paper
正在生成论文摘要