Dual Multiscale Mean Teacher Network for Semi-Supervised Infection Segmentation in Chest CT Volume for COVID-19
arxiv(2023)
摘要
Automated detecting lung infections from computed tomography (CT) data plays an important role for combating coronavirus 2019 (COVID-19). However, there are still some challenges for developing AI system: 1) most current COVID-19 infection segmentation methods mainly relied on 2-D CT images, which lack 3-D sequential constraint; 2) existing 3-D CT segmentation methods focus on single-scale representations, which do not achieve the multiple level receptive field sizes on 3-D volume; and 3) the emergent breaking out of COVID-19 makes it hard to annotate sufficient CT volumes for training deep model. To address these issues, we first build a multiple dimensional-attention convolutional neural network (MDA-CNN) to aggregate multiscale information along different dimension of input feature maps and impose supervision on multiple predictions from different convolutional neural networks (CNNs) layers. Second, we assign this MDA-CNN as a basic network into a novel dual multiscale mean teacher network (DM
${^{2}}\text{T}$
-Net) for semi-supervised COVID-19 lung infection segmentation on CT volumes by leveraging unlabeled data and exploring the multiscale information. Our DM
${^{2}}\text{T}$
-Net encourages multiple predictions at different CNN layers from the student and teacher networks to be consistent for computing a multiscale consistency loss on unlabeled data, which is then added to the supervised loss on the labeled data from multiple predictions of MDA-CNN. Third, we collect two COVID-19 segmentation datasets to evaluate our method. The experimental results show that our network consistently outperforms the compared state-of-the-art methods.
更多查看译文
关键词
Chest computed tomography (CT),coronavirus 2019 (COVID-19),infection segmentation,semi-supervised learning
AI 理解论文
溯源树
样例
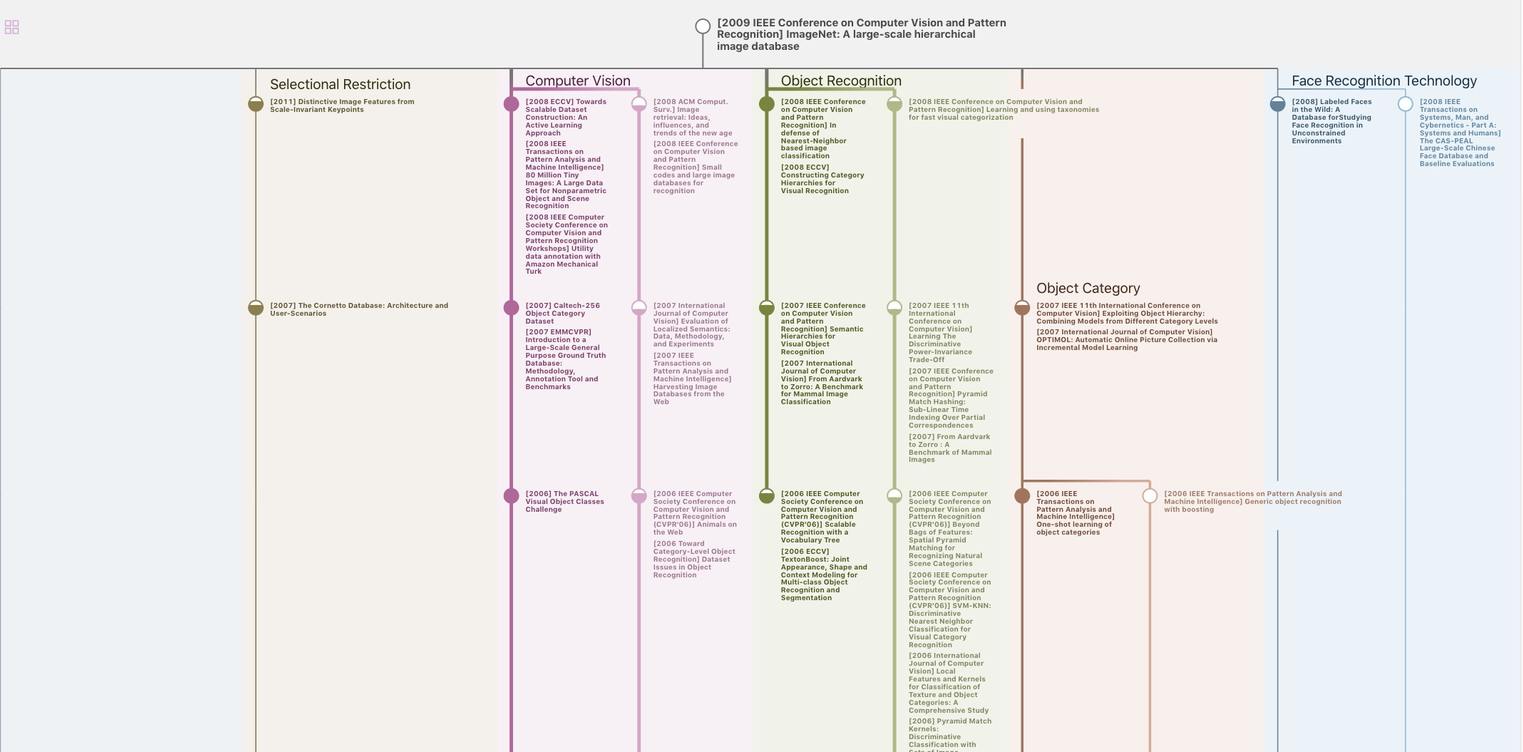
生成溯源树,研究论文发展脉络
Chat Paper
正在生成论文摘要