An Empirical Study on Clustering Pretrained Embeddings: Is Deep Strictly Better?
arxiv(2022)
摘要
Recent research in clustering face embeddings has found that unsupervised, shallow, heuristic-based methods -- including $k$-means and hierarchical agglomerative clustering -- underperform supervised, deep, inductive methods. While the reported improvements are indeed impressive, experiments are mostly limited to face datasets, where the clustered embeddings are highly discriminative or well-separated by class (Recall@1 above 90% and often nearing ceiling), and the experimental methodology seemingly favors the deep methods. We conduct a large-scale empirical study of 17 clustering methods across three datasets and obtain several robust findings. Notably, deep methods are surprisingly fragile for embeddings with more uncertainty, where they match or even perform worse than shallow, heuristic-based methods. When embeddings are highly discriminative, deep methods do outperform the baselines, consistent with past results, but the margin between methods is much smaller than previously reported. We believe our benchmarks broaden the scope of supervised clustering methods beyond the face domain and can serve as a foundation on which these methods could be improved. To enable reproducibility, we include all necessary details in the appendices, and plan to release the code.
更多查看译文
关键词
clustering pretrained embeddings,deep,empirical study
AI 理解论文
溯源树
样例
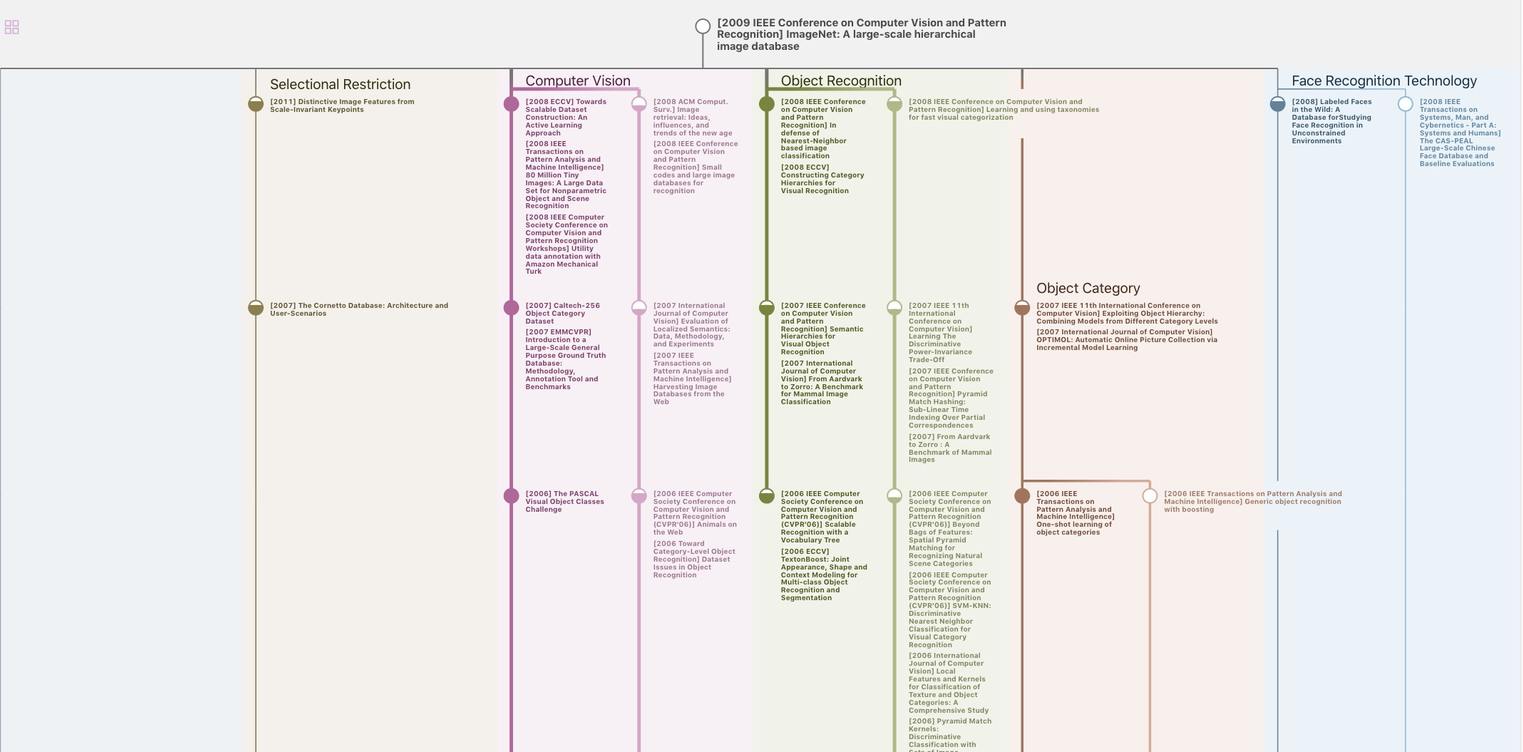
生成溯源树,研究论文发展脉络
Chat Paper
正在生成论文摘要