Can machine learning ‘transform’ peptides/peptidomimetics into small molecules? A case study with ghrelin receptor ligands
MOLECULAR DIVERSITY(2022)
摘要
There has been considerable interest in transforming peptides into small molecules as peptide-based molecules often present poorer bioavailability and lower metabolic stability. Our studies looked into building machine learning (ML) models to investigate if ML is able to identify the ‘bioactive’ features of peptides and use the features to accurately discriminate between binding and non-binding small molecules. The ghrelin receptor (GR), a receptor that is implicated in various diseases, was used as an example to demonstrate whether ML models derived from a peptide library can be used to predict small molecule binders. ML models based on three different algorithms, namely random forest, support vector machine, and extreme gradient boosting, were built based on a carefully curated dataset of peptide/peptidomimetic and small molecule GR ligands. The results indicated that ML models trained with a dataset exclusively composed of peptides/peptidomimetics provide limited predictive power for small molecules, but that ML models trained with a diverse dataset composed of an array of both peptides/peptidomimetics and small molecules displayed exceptional results in terms of accuracy and false rates. The diversified models can accurately differentiate the binding small molecules from non-binding small molecules using an external validation set with new small molecules that we synthesized previously. Structural features that are the most critical contributors to binding activity were extracted and are remarkably consistent with the crystallography and mutagenesis studies. Graphical abstract
更多查看译文
关键词
Machine learning,Drug design,Ghrelin receptor,Peptide
AI 理解论文
溯源树
样例
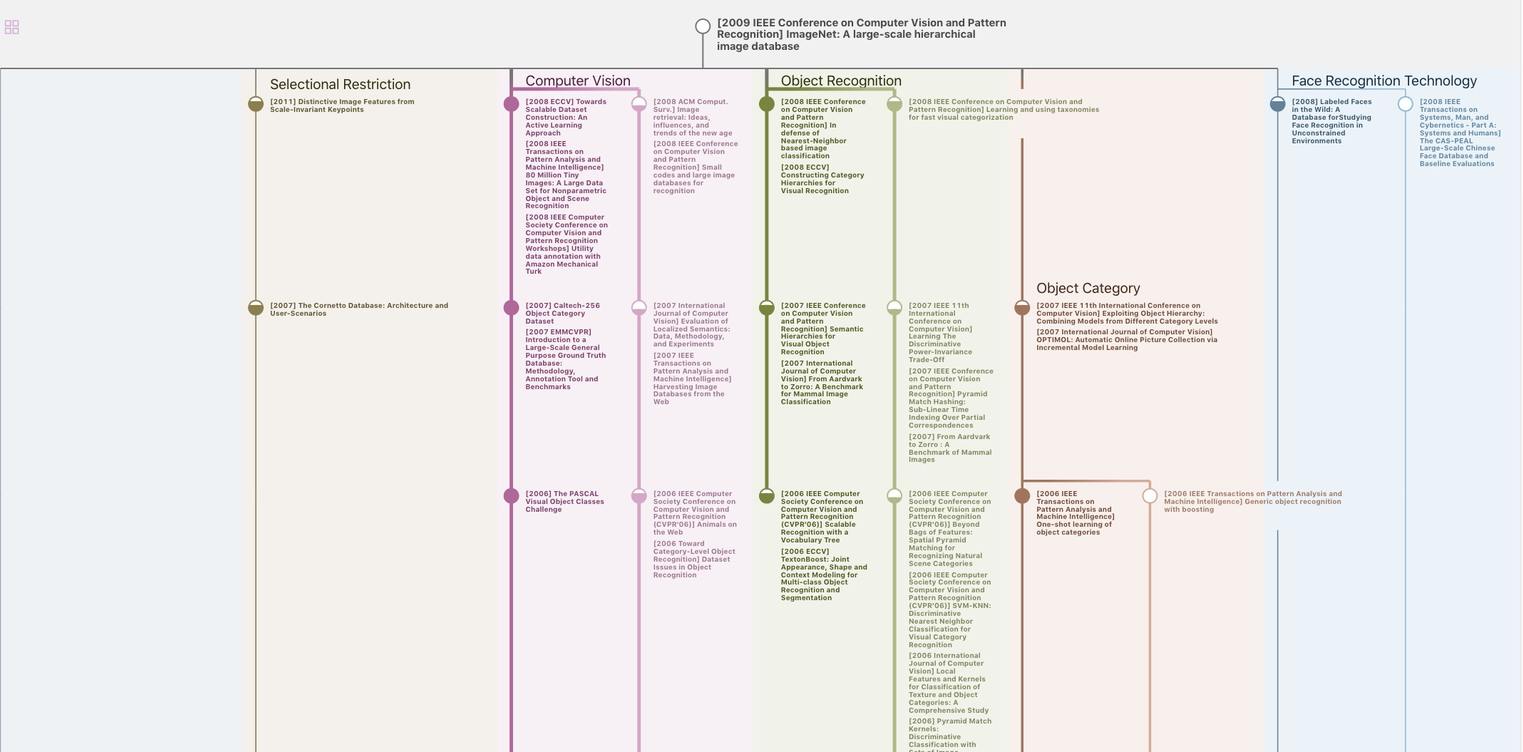
生成溯源树,研究论文发展脉络
Chat Paper
正在生成论文摘要