Hierarchical Associative Encoding and Decoding for Bottom-Up Human Pose Estimation
IEEE Transactions on Circuits and Systems for Video Technology(2023)
摘要
Bottom-up human pose estimation decouples computational complexity from the number of people but requires additional operations to match the detected keypoints to each human instance. Existing approaches treat all keypoints equally while ignoring the relationships among keypoints, which in turn limit the performance ceilings. In this work, we propose a hierarchical associative encoding and decoding framework for bottom-up human pose estimation by introducing additional prior knowledge. Specifically, in addition to keypoint-level and instance-level associations, we further divide keypoints into groups and explore group-level associations. This way, prior knowledge is incorporated to determine the keypoint groups for better associative encoding. To deal with complex poses, we introduce a focal pulling loss to focus more on the hard-to-associate keypoints. Moreover, instead of using a pre-defined order for keypoint grouping, we propose a progressive associative decoding method to dynamically determine the order of keypoints for grouping, which helps reduce isolated keypoints. Experimental results on the MS-COCO, CrowdPose and MPII datasets show superior performance of our proposed associative encoding and decoding algorithms. More importantly, we prove, through validation, that hierarchical associative encoding and decoding can be used as a plug-n-play module for performance improvement regardless of backbone architecture. Our source code and pretrained models are available at
https://github.com/ducongju/HAE
.
更多查看译文
关键词
Bottom-up human pose estimation,hierarchical associative encoding,progressive associative decoding,associative embedding
AI 理解论文
溯源树
样例
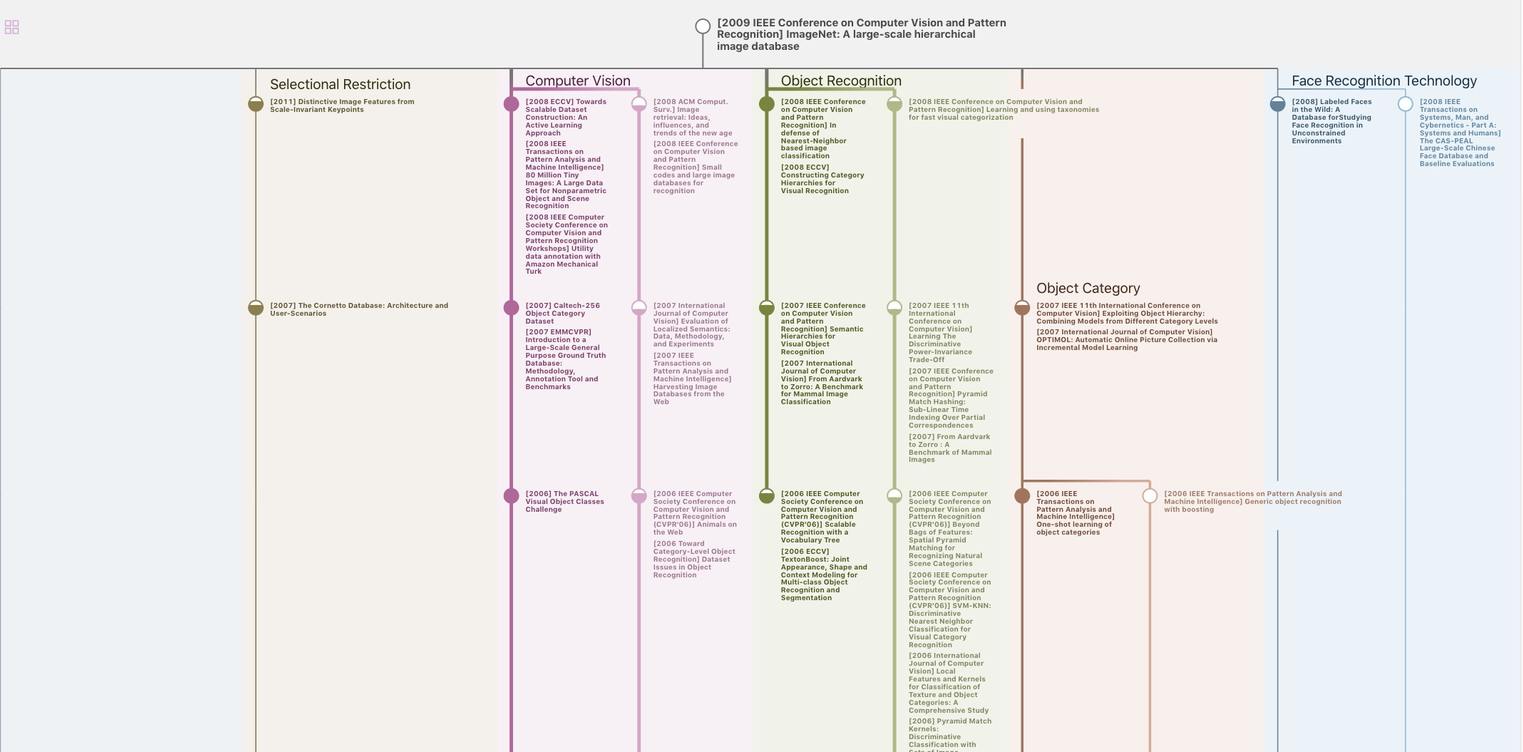
生成溯源树,研究论文发展脉络
Chat Paper
正在生成论文摘要