A Network-based Modeling Approach to Identify the Common Disease Classes in Patients with Late-stage Prostate Cancer
2022 IEEE 10th International Conference on Healthcare Informatics (ICHI)(2022)
摘要
Prostate cancer (PCa) is one of the most common non-cutaneous cancers, and the second major cause of cancer death among men in the United States. The comorbidities study of PCa patients can inform treatment priority management and patient-oriented care. In this study, using the SEER-Medicare database, we adopt a network-based modeling approach to identify the most common disease classes among male with late-stage (stage D) PCa. The dataset comprises patients diagnosed with stage D PCa between January 1, 2010, and December 31, 2015, and a control group consisted of an equal size random sample of men without cancer. For both stage D PCa and control subpopulations, we created a set of 116 networks. Each network in the set represents a disease category identified using subsections of ICD-9 disease codes. In these networks, diseases and complications (identified using ICD-9 codes) are used as networks' nodes. Two nodes are connected if their related diseases' co-occurrences are observed in the dataset. Furthermore, the edges are weighted by the co-occurrence probability of complication pairs. A statistical approach was adopted to compare the differences between corresponding networks in the two groups. Out of 116 subsections, 59 had more than 30 distinct pairs, from which 51 subsections were statistically different between the control and the stage D PCa subpopulations (p - value < 0.001). Besides, a set of patient-level networks was developed for each patient in the two groups. The properties and components of patient-level networks were used as features in machine learning algorithms for predicting stage D PCa. The results show that the characteristics of the co-occurring diseases obtained from network modeling can help discover significant health issues in patients' subpopulations,
更多查看译文
关键词
prostate cancer,network modeling,machine learning
AI 理解论文
溯源树
样例
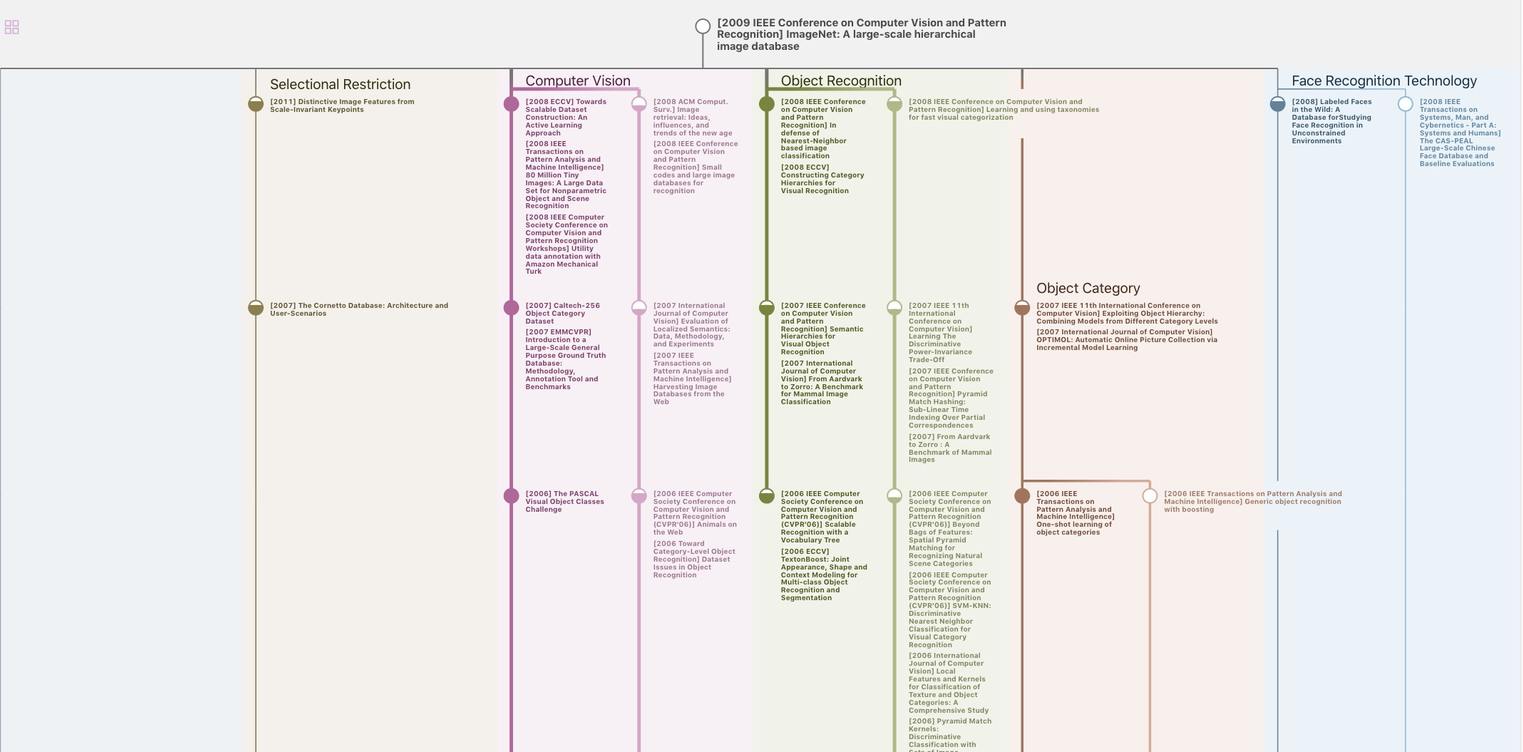
生成溯源树,研究论文发展脉络
Chat Paper
正在生成论文摘要