Understanding the effectiveness of enzyme pre-reaction state by a quantum-based machine learning model
Cell Reports Physical Science(2022)
摘要
Prediction of enzymatic stereochemistry is a significant challenge in computational chemistry because of targeting very small energy gaps in highly complicated macromolecular systems. Here, we report a scenario of four substrates (2-pentanone, 2-hexanone, 2-heptanone, and 2-octanone) within four enzyme variants (wild type, W116A, F285A, and W286A) of a medium-chain dehydroge-nase from Candida parapsilopsis. The relative stabilities of pro-R and pro-S pre-reaction states are calculated by umbrella sampling, approximately consistent with the observed stereoselectivity in experiment. Besides, a LASSO-SVM machine-learning model is con-structed with structural information of 704 pairs of quantum-mech-anistic/molecular-mechanistic transition states (TSs) and pre -reac-tion states (PRSs), achieving the explanatory power of 99.6% for the calculated barriers. Intriguingly, the explanatory power with the PRS-alone structural information reaches 90.7%, but it de-creases to 55.4% with the TS-alone structural information. Thus, the outcomes support that the enzymatic stereoselectivity is sub-stantially determined by the frontier-molecular-orbital-related pre -organization of the enzyme-substrate reacting complexes.
更多查看译文
关键词
machine learning,zinc-dependent medium-chain alcohol dehydrogenase,pre-reaction state,frontier molecular orbital,enantioselectivity,Prelog,anti-Prelog,molecular dynamics simulations,quantum mechanics/molecular mechanics,secondary orbital interaction
AI 理解论文
溯源树
样例
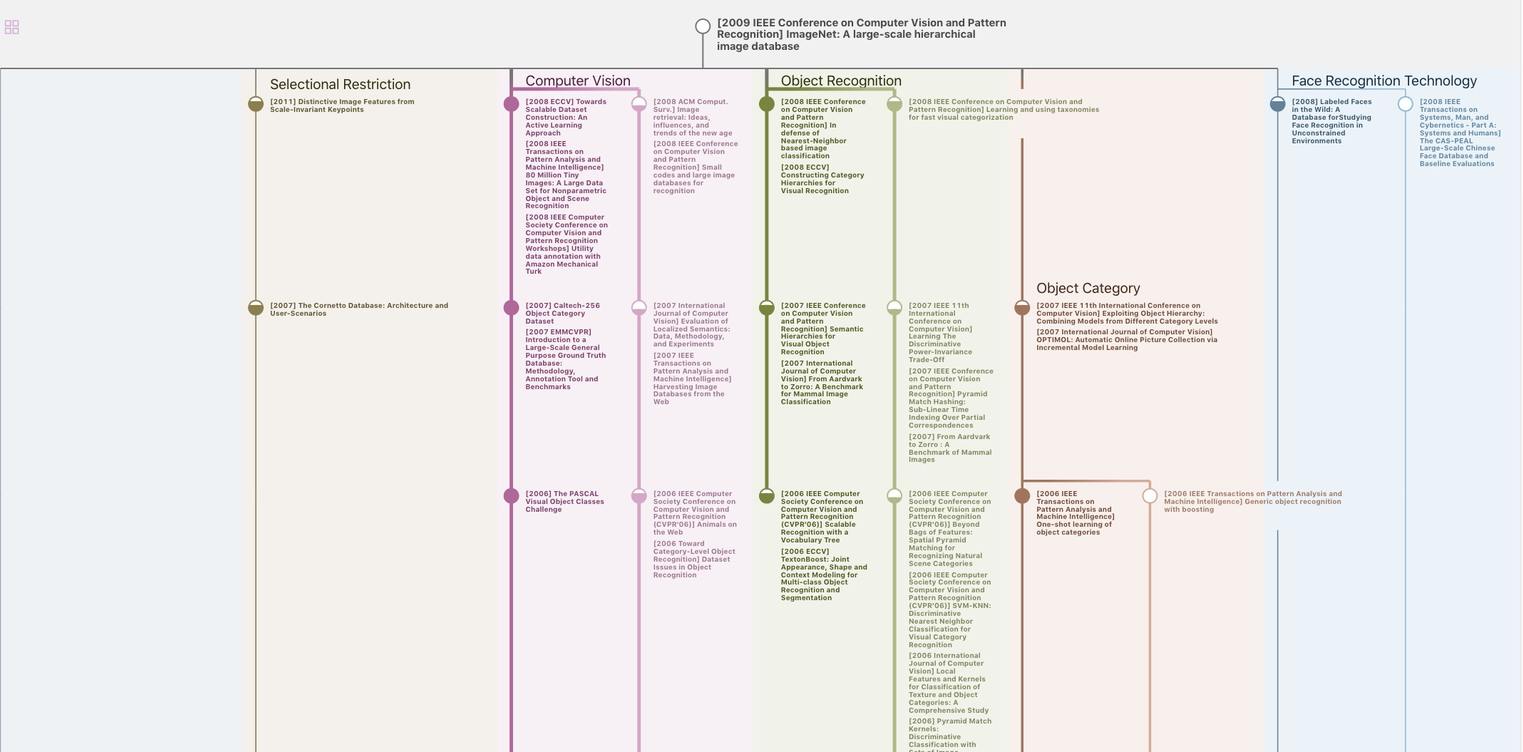
生成溯源树,研究论文发展脉络
Chat Paper
正在生成论文摘要