An autoencoder-based feature level fusion for speech emotion recognition
Digital Communications and Networks(2022)
摘要
Although speech emotion recognition is challenging, it has broad application prospects in human-computer interaction. Building a system that can accurately and stably recognize emotions from human languages can provide a better user experience. However, the current unimodal emotion feature representations are not distinctive enough to accomplish the recognition, and they do not effectively simulate the inter-modality dynamics in speech emotion recognition tasks. This paper proposes a multimodal method that utilizes both audio and semantic content for speech emotion recognition. The proposed method consists of three parts: two high-level feature extractors for text and audio modalities, and an autoencoder-based feature fusion. For audio modality, we propose a structure called Temporal Global Feature Extractor (TGFE) to extract the high-level features of the time-frequency domain relationship from the original speech signal. Considering that text lacks frequency information, we use only a Bidirectional Long Short-Term Memory network (BLSTM) and attention mechanism to simulate an intra-modal dynamic. Once these steps have been accomplished, the high-level text and audio features are sent to the autoencoder in parallel to learn their shared representation for final emotion classification. We conducted extensive experiments on three public benchmark datasets to evaluate our method. The results on Interactive Emotional Motion Capture (IEMOCAP) and Multimodal EmotionLines Dataset (MELD) outperform the existing method. Additionally, the results of CMU Multi-modal Opinion-level Sentiment Intensity (CMU-MOSI) are competitive. Furthermore, experimental results show that compared to unimodal information and autoencoder-based feature level fusion, the joint multimodal information (audio and text) improves the overall performance and can achieve greater accuracy than simple feature concatenation.
更多查看译文
关键词
Attention mechanism,Autoencoder,Bimodal fusion,Emotion recognition
AI 理解论文
溯源树
样例
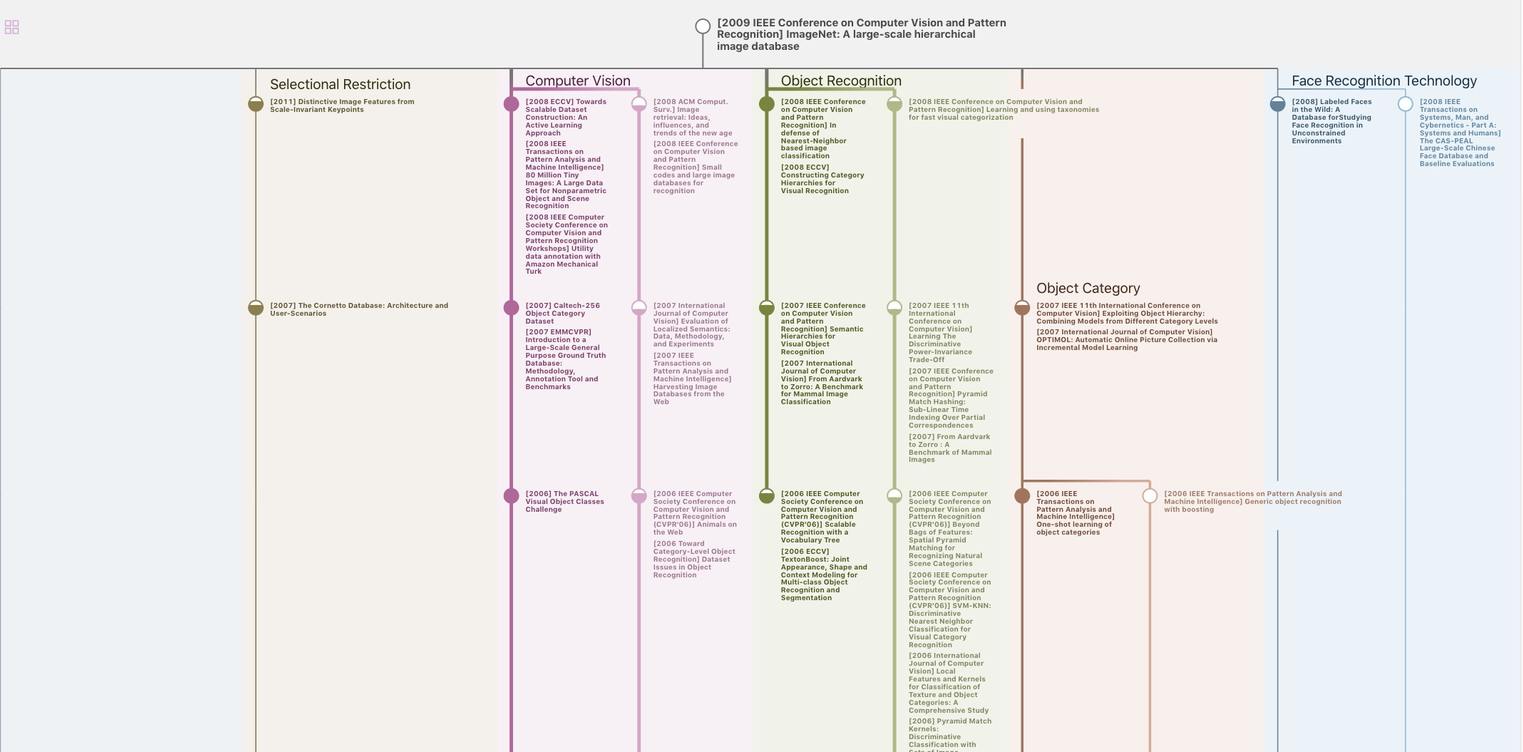
生成溯源树,研究论文发展脉络
Chat Paper
正在生成论文摘要