DIANA: An End-to-End Energy-Efficient Digital and ANAlog Hybrid Neural Network SoC.
International Solid-State Circuits Conference(2022)
摘要
Energy-efficient matrix-vector multiplications (MVMs) are key to bringing neural network (NN) inference to edge devices. This has led to a wide range of state-of-the-art MVM acceleration chips, which fall into two categories: 1) Digital NN accelerators [1]–[2], constituting widely parallel multiply-accumulate (MAC) arrays at medium (typically 4-8b) precision. 2) Analog in-memory compute (AiMC) NN accelerators [3]–[4], which enable much higher energy efficiencies and throughput per unit area at the cost of a reduced computational precision, reduced dataflow flexibility, and resulting reduced mapping efficiency for some layer configurations. Neither of these approaches dominates the other, as it depends on the layer type which approach is the optimal. The ideal processor would enable exploiting both digital and AiMC NN acceleration concepts and select the best accelerator depending on the layer characteristics. Consequently, this work presents DIANA, a low-power NN processing SoC, comprising a precision-scalable digital NN accelerator, an AiMC core, an optimized shared-memory subsystem and a RISC-V host processor to achieve SOTA end-to-end inference at the edge. This SoC includes innovations in: a) its 16x16 digital NN core with flexible dataflow for fully connected and high-precision CONV layer execution, b) its 1152x512 AiMC core with SIMD digital post-processing and support for output unrolling for improving array utilization, and c) a shared memory system supporting efficient layer-fused execution schedules, controlled by the RISC-V. This allows simultaneous execution of subsequent layers across the digital and analog cores, assigning high-precision layers and layers with limited AiMC utilization (e.g. FC layers and layers with low channel count) to the digital core, and all other intermediate layers to the AiMC core. A top-level overview of the designed system and its highlights is depicted in Fig. 15.6.1.
更多查看译文
关键词
DIANA,energy-efficient matrix-vector multiplications,neural network inference,higher energy efficiencies,reduced computational precision,reduced dataflow flexibility,reduced mapping efficiency,layer configurations,layer characteristics,low-power NN processing SoC,precision-scalable digital NN accelerator,optimized shared-memory subsystem,SOTA end-to-end inference,high-precision CONV layer execution,SIMD digital post-processing,efficient layer-fused execution schedules,digital analog cores,high-precision layers,AiMC utilization,FC layers,layers with low channel count,intermediate layers,AiMC core,digital NN core,AiMC NN acceleration concepts,MVM acceleration chips,end-to-end energy-efficient digital and analog hybrid neural network SoC
AI 理解论文
溯源树
样例
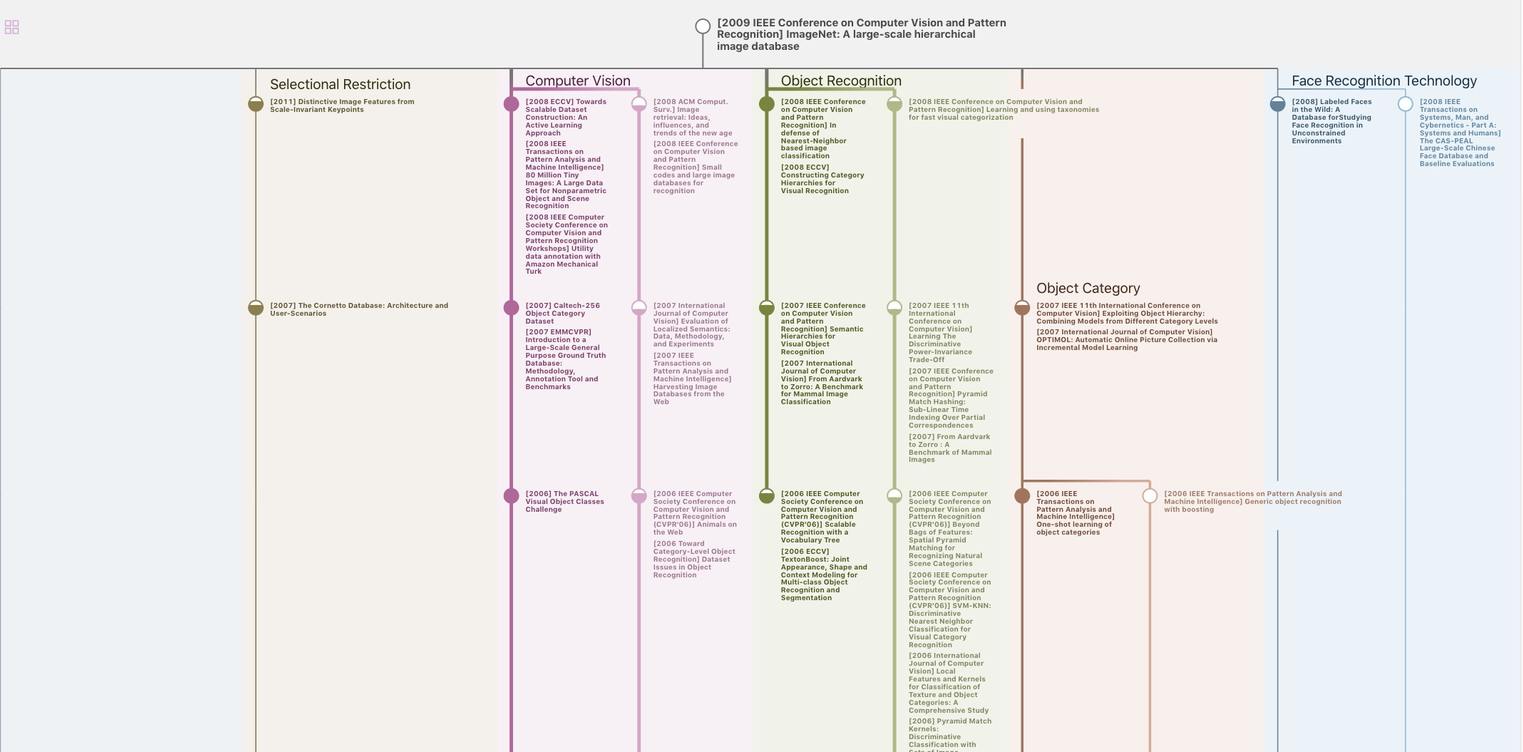
生成溯源树,研究论文发展脉络
Chat Paper
正在生成论文摘要