Multi-touch Attribution for Complex B2B Customer Journeys using Temporal Convolutional Networks.
International Workshop on Multimodal Human Understanding for the Web and Social Media(2022)
摘要
Customer journeys in Business-to-Business (B2B) transactions contain long and complex sequences of interactions between different stakeholders from the buyer and seller companies. On the seller side, there is significant interest in the multi-touch attribution (MTA) problem, which aims to identify the most influential stage transitions (in the B2B customer funnel), channels, and touchpoints. We design a novel deep learning-based framework, which solves these attribution problems by modeling the conversion of journeys as functions of stage transitions that occur in them. Each stage transition is modeled as a Temporal Convolutional Network (TCN) on the touchpoints that precede it. Further, a global conversion model Stage-TCN is built by combining these individual stage transition models in a non-linear fashion. We apply Layer-wise Relevance Propagation (LRP) based techniques to compute the relevance of all nodes and inputs in our network and use these to compute the required attribution scores. We run extensive experiments on two real-world B2B datasets and demonstrate superior accuracy of the conversion model compared to prior works. We validate the attribution scores using perturbation-based techniques that measure the change in model output when parts of the input having high attribution scores are deleted.
更多查看译文
关键词
Business-to-Business Relationships
AI 理解论文
溯源树
样例
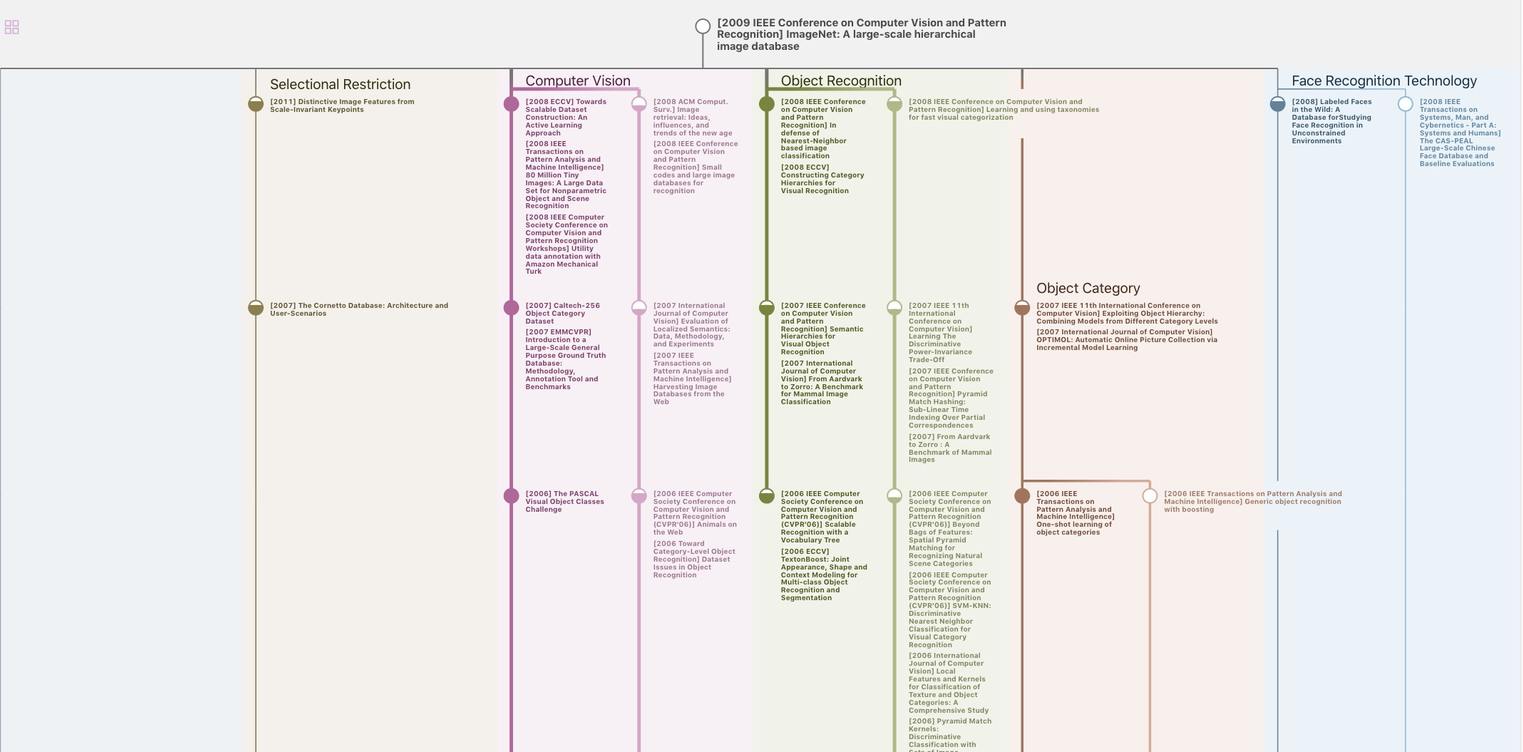
生成溯源树,研究论文发展脉络
Chat Paper
正在生成论文摘要