Ada-JSR: Sample Efficient Adaptive Joint Support Recovery From Extremely Compressed Measurement Vectors.
IEEE International Conference on Acoustics, Speech, and Signal Processing (ICASSP)(2022)
摘要
This paper considers the problem of recovering the joint support (of size K) of a set of unknown sparse vectors in R-d, each of which can be sensed using a different measurement matrix. Such models have wide applicability ranging from communication to multi-task learning. We develop an adaptive strategy called Adaptive Joint Support Recovery (AdaJSR) that enables exact support recovery in the extreme compression regime with only m = 1 measurement per unknown vector while requiring a total complexity of no more than K left perpendicular log(2) p(d) right perpendicular measurements. Unlike existing support recovery techniques which require suitable assumptions on the correlation structure or distribution of the unknown signals in order to operate in the regime m < K, we show that the flexibility of adaptive measurement design alone allows us to operate in this extreme compression regime, without the need for imposing any correlation or sub-Gaussian priors.
更多查看译文
关键词
Joint Support Recovery,Multiple Measurement Vector,Adaptive Sensing,mmWave Channel Subspace Estimation,Multi-Task Learning
AI 理解论文
溯源树
样例
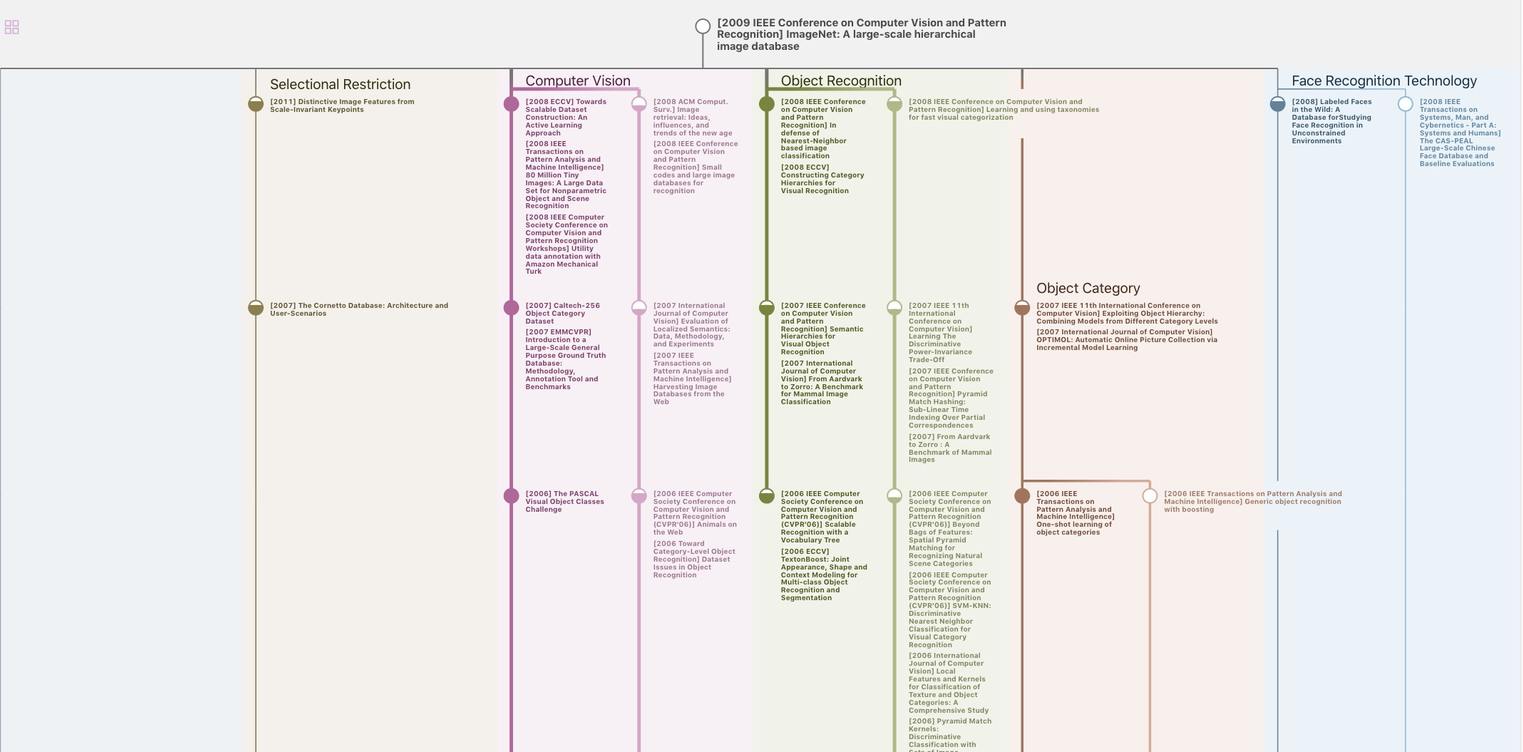
生成溯源树,研究论文发展脉络
Chat Paper
正在生成论文摘要