A Universal Identity Backdoor Attack against Speaker Verification based on Siamese Network.
Conference of the International Speech Communication Association (INTERSPEECH)(2022)
摘要
Speaker verification has been widely used in many authentication scenarios. However, training models for speaker verification requires large amounts of data and computing power, so users often use untrustworthy third-party data or deploy third-party models directly, which may create security risks. In this paper, we propose a backdoor attack for the above scenario. Specifically, for the Siamese network in the speaker verification system, we try to implant a universal identity in the model that can simulate any enrolled speaker and pass the verification. So the attacker does not need to know the victim, which makes the attack more flexible and stealthy. In addition, we design and compare three ways of selecting attacker utterances and two ways of poisoned training for the GE2E loss function in different scenarios. The results on the TIMIT and Voxceleb1 datasets show that our approach can achieve a high attack success rate while guaranteeing the normal verification accuracy. Our work reveals the vulnerability of the speaker verification system and provides a new perspective to further improve the robustness of the system.
更多查看译文
关键词
Speaker Verification, Backdoor Attack, AI Security, Deep Learning
AI 理解论文
溯源树
样例
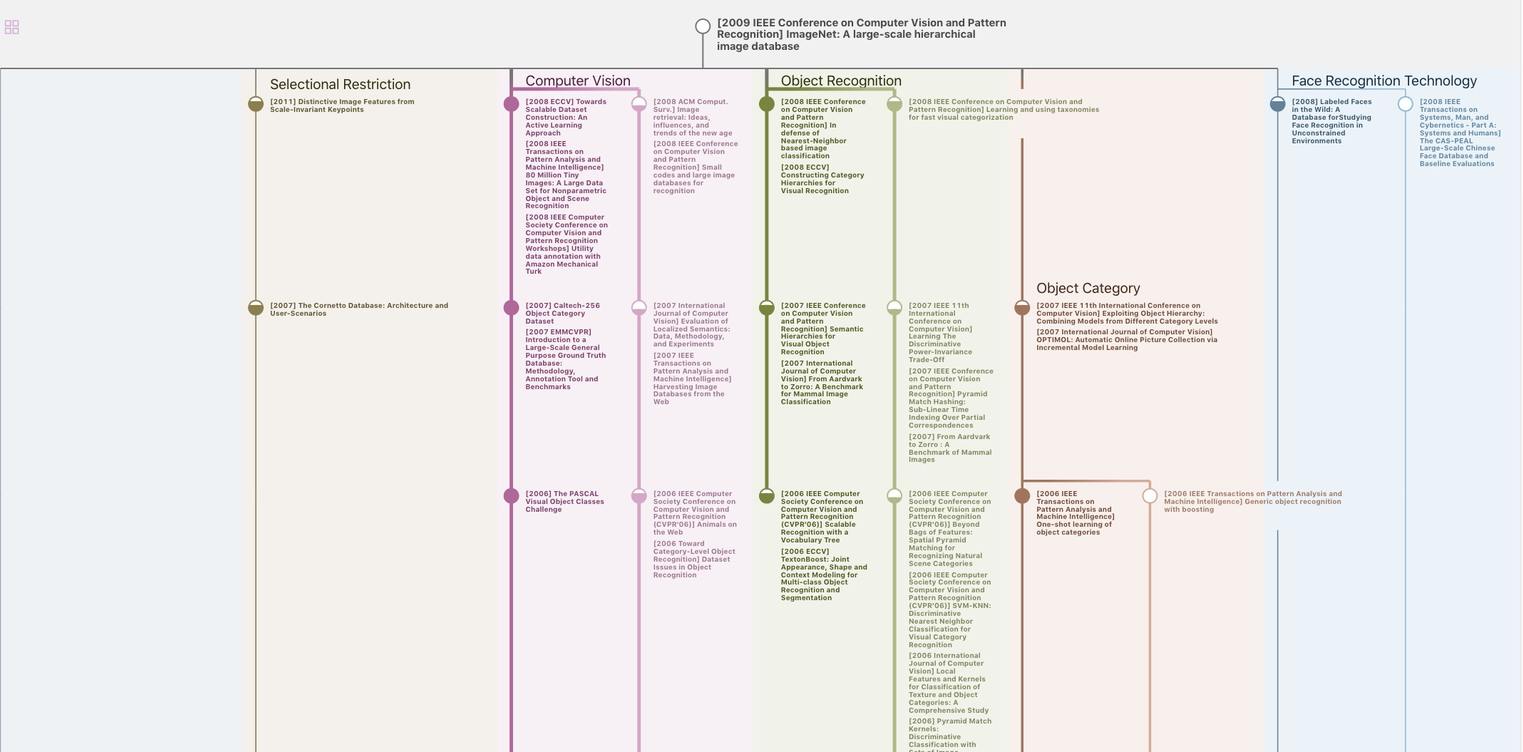
生成溯源树,研究论文发展脉络
Chat Paper
正在生成论文摘要