Improving time series features identification by means of Convolutional Neural Networks and Recurrence Plot
IFAC-PapersOnLine(2022)
摘要
Within business forecasting, the choice of the forecasting model is crucial. In fact, not all models can be functional to all time series and their applicability is based on the features of the considered time series, such as trend, seasonality and randomness. The identification of features is, then, a fundamental activity to achieve the desired forecasting performance. On the other hand, time series features identification is known to be a time-consuming activity that requires the intervention of the decision maker. Hence, performing such a step of the forecasting process in an automated and reliable way would considerably improve the whole process. To this aim, this work proposes a method that combines the Recurrence Plot (RP) and the Convolutional Neural Networks (CNNs) to perform typical business time series features identification. RP is a visual tool that represents recurrence of the state space system by means of oriented structures in 2D images. CNNs are popular deep learning models that have achieved high recognition rates performing image classification tasks. Machine learning algorithms have been already successfully applied to the business forecasting process, limited to forecasting model selection step, while the CNN have been successfully applied in different fields, such as medicine, distinguishing ischemic disease in electroencephalogram images, not yet to business time series features identification. This work applies the GoogLeNet CNN to the RPs that represent simulated time series and measures the obtained classification accuracy by means of confusion matrix.
更多查看译文
关键词
Recurrence Plot,Convolutional Neural Networks,features identification,time series,forecasting,GoogLeNet
AI 理解论文
溯源树
样例
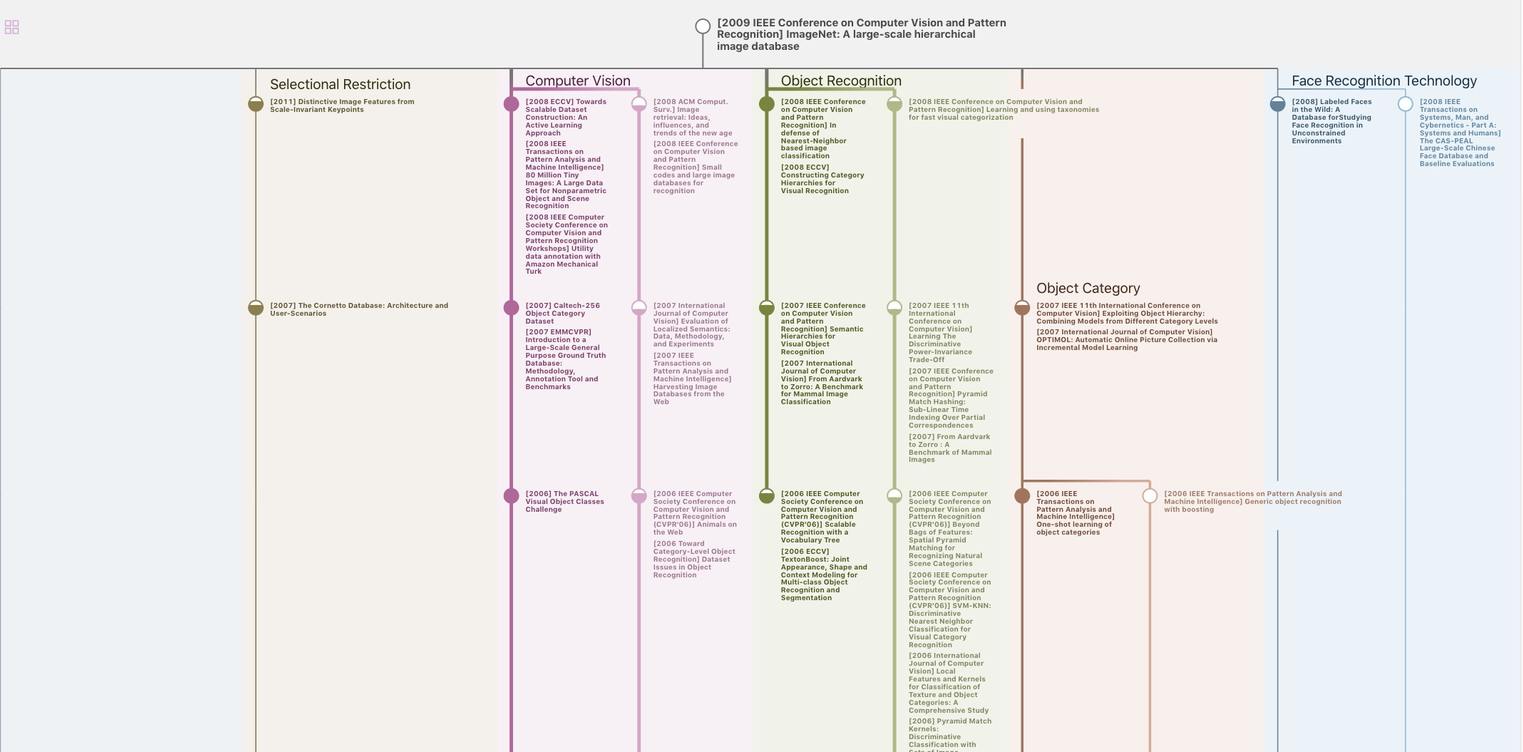
生成溯源树,研究论文发展脉络
Chat Paper
正在生成论文摘要