An Optimized Uncertainty-Aware Training Framework for Neural Networks.
IEEE transactions on neural networks and learning systems(2024)
摘要
Uncertainty quantification (UQ) for predictions generated by neural networks (NNs) is of vital importance in safety-critical applications. An ideal model is supposed to generate low uncertainty for correct predictions and high uncertainty for incorrect predictions. The main focus of state-of-the-art training algorithms is to optimize the NN parameters to improve the accuracy-related metrics. Training based on uncertainty metrics has been fully ignored or overlooked in the literature. This article introduces a novel uncertainty-aware training algorithm for classification tasks. A novel predictive uncertainty estimate-based objective function is defined and optimized using the stochastic gradient descent method. This new multiobjective loss function covers both accuracy and uncertainty accuracy (UA) simultaneously during training. The performance of the proposed training framework is compared from different aspects with other UQ techniques for different benchmarks. The obtained results demonstrate the effectiveness of the proposed framework for developing the NN models capable of generating reliable uncertainty estimates.
更多查看译文
AI 理解论文
溯源树
样例
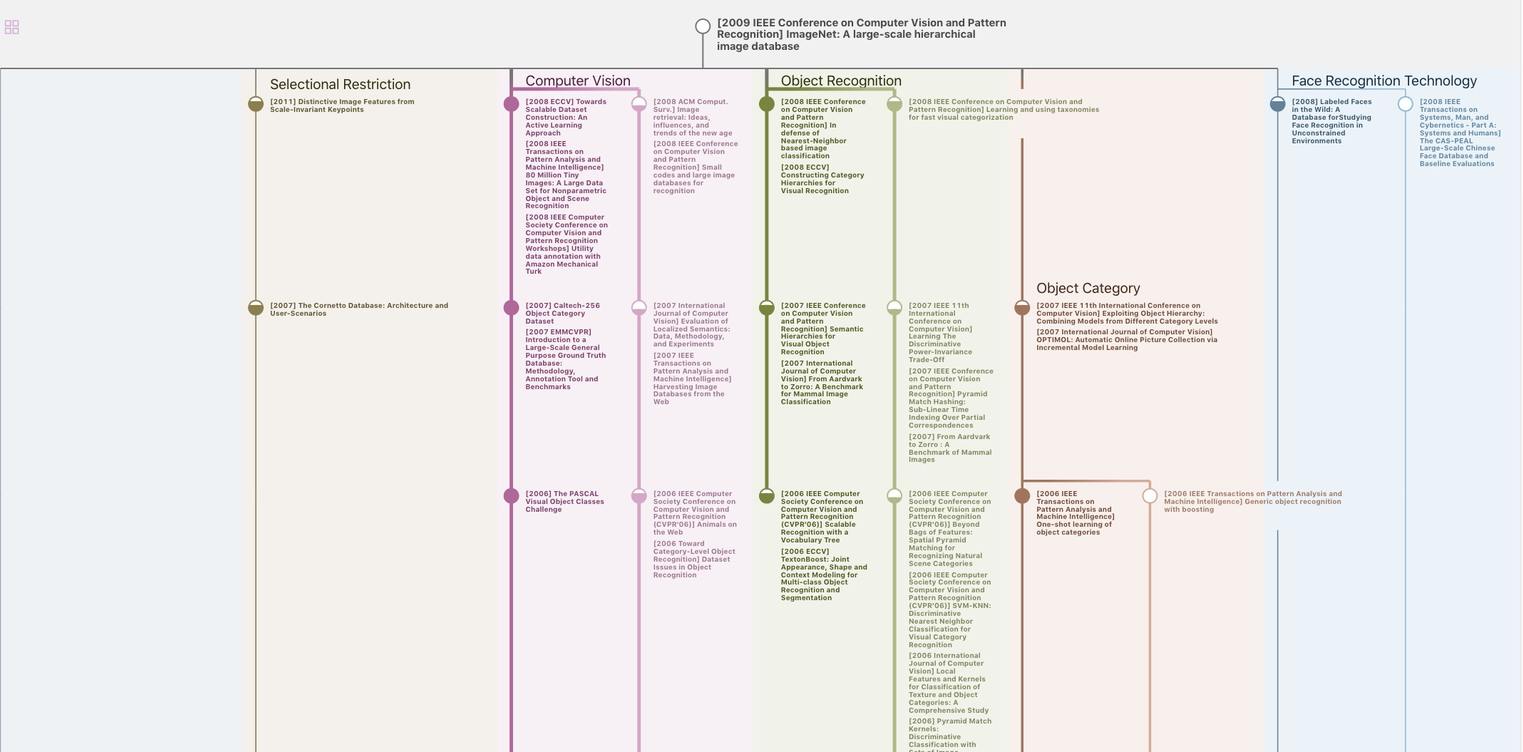
生成溯源树,研究论文发展脉络
Chat Paper
正在生成论文摘要