A new perspective for understanding generalization gap of deep neural networks trained with large batch sizes
Applied Intelligence(2022)
摘要
Deep neural networks (DNNs) are typically optimized using various forms of mini-batch gradient descent algorithm. A major motivation for mini-batch gradient descent is that with a suitably chosen batch size, available computing resources can be optimally utilized (including parallelization) for fast model training. However, many works report the progressive loss of model generalization when the training batch size is increased beyond some limits. This is a scenario commonly referred to as generalization gap. Although several works have proposed different methods for alleviating the generalization gap problem, a unanimous account for understanding generalization gap is still lacking in the literature. This is especially important given that recent works have observed that several proposed solutions for generalization gap problem such learning rate scaling and increased training budget do not indeed resolve it. As such, our main exposition in this paper is to investigate and provide new perspectives for the source of generalization loss for DNNs trained with a large batch size. Our analysis suggests that large training batch size results in increased near-rank loss of units’ activation (i.e. output) tensors, which consequently impacts model optimization and generalization. Extensive experiments are performed for validation on popular DNN models such as VGG-16, residual network (ResNet-56) and LeNet-5 using CIFAR-10, CIFAR-100, Fashion-MNIST and MNIST datasets.
更多查看译文
关键词
Neural network, Large batch size, Generalization gap, Optimization
AI 理解论文
溯源树
样例
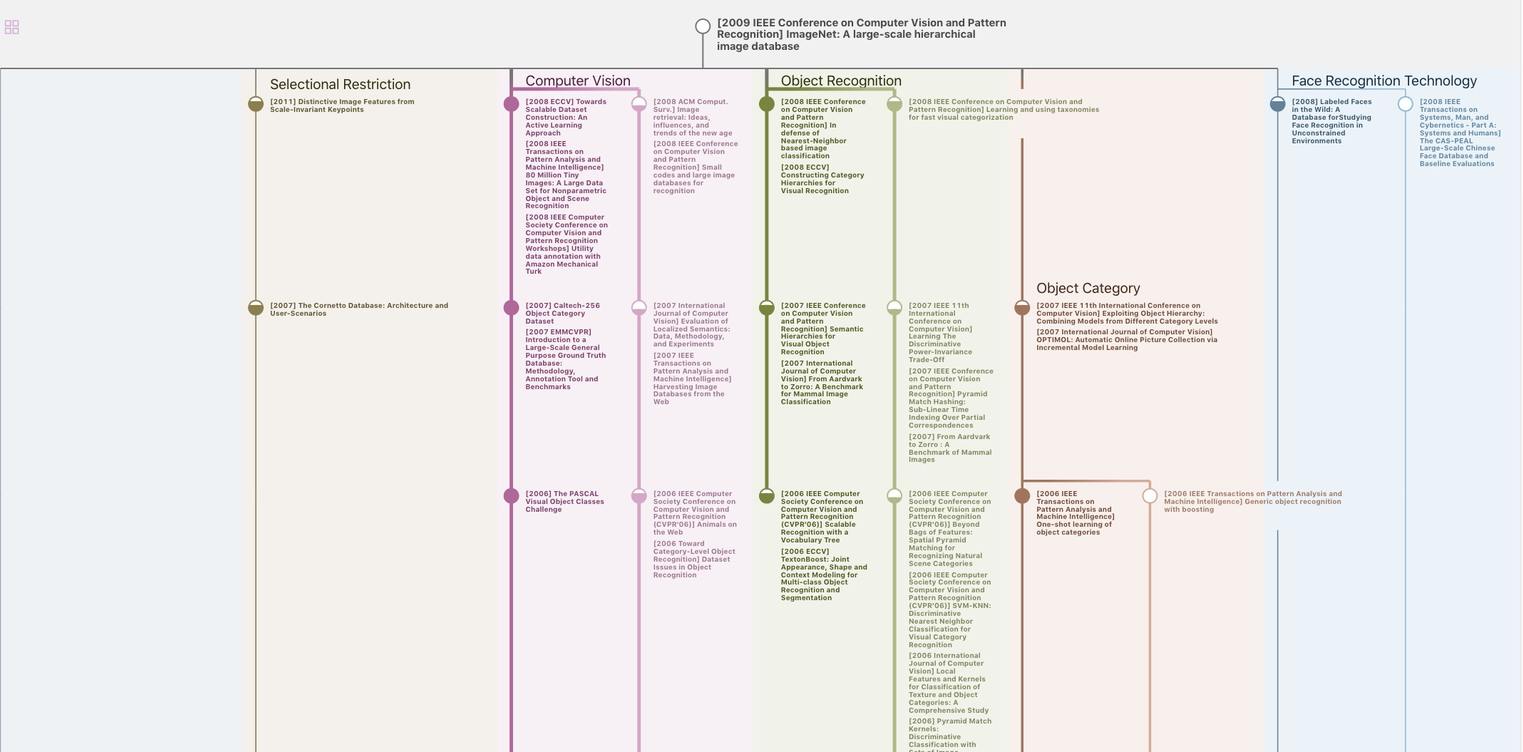
生成溯源树,研究论文发展脉络
Chat Paper
正在生成论文摘要