SMaLL-100: Introducing Shallow Multilingual Machine Translation Model for Low-Resource Languages
emnlp 2022(2022)
摘要
In recent years, multilingual machine translation models have achieved promising performance on low-resource language pairs by sharing information between similar languages, thus enabling zero-shot translation. To overcome the "curse of multilinguality", these models often opt for scaling up the number of parameters, which makes their use in resource-constrained environments challenging. We introduce SMaLL-100, a distilled version of the M2M-100 (12B) model, a massively multilingual machine translation model covering 100 languages. We train SMaLL-100 with uniform sampling across all language pairs and therefore focus on preserving the performance of low-resource languages. We evaluate SMaLL-100 on different low-resource benchmarks: FLORES-101, Tatoeba, and TICO-19 and demonstrate that it outperforms previous massively multilingual models of comparable sizes (200-600M) while improving inference latency and memory usage. Additionally, our model achieves comparable results to M2M-100 (1.2B), while being 3.6x smaller and 4.3x faster at inference. Code and pre-trained models: https://github.com/alirezamshi/small100
更多查看译文
关键词
translation,languages,low-resource
AI 理解论文
溯源树
样例
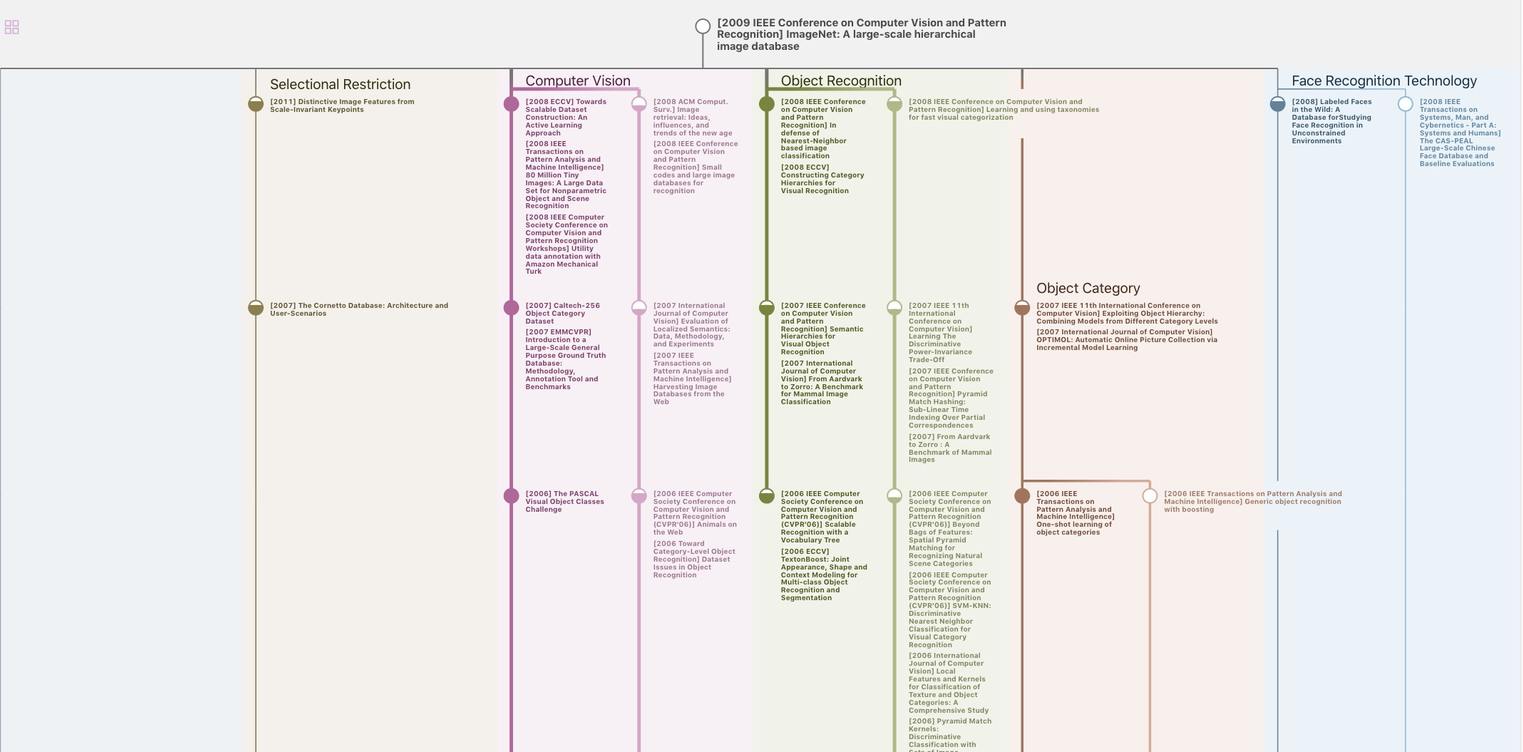
生成溯源树,研究论文发展脉络
Chat Paper
正在生成论文摘要