Physics-Constraint Variational Neural Network for Wear State Assessment of External Gear Pump.
IEEE transactions on neural networks and learning systems(2024)
摘要
Most current data-driven prognosis approaches suffer from their uncontrollable and unexplainable properties. To address this issue, this article proposes a physics-constraint variational neural network (PCVNN) for wear state assessment of the external gear pump. First, a response model of the pressure pulsation of the gear pump is constructed via a spectral method, and a compound neural network is utilized to extract features from the pressure pulsation signal. Then, the response model is formulated into an objective function to softly constrain the learning process of the neural network, forcing the learned features to have explicit physics meaning. Meanwhile, to characterize the system uncertainty, the variational inference is utilized to extend a Kullback-Leibler (KL) divergence into the objective function. Finally, the wear state is evaluated based on the distance of learned physics features. Experimental results on an external gear pump validate the merits of the proposed method in explainable representation learning and system uncertainty estimation. It also offers a controllable and explainable perspective to understand the dynamic behavior of the system.
更多查看译文
AI 理解论文
溯源树
样例
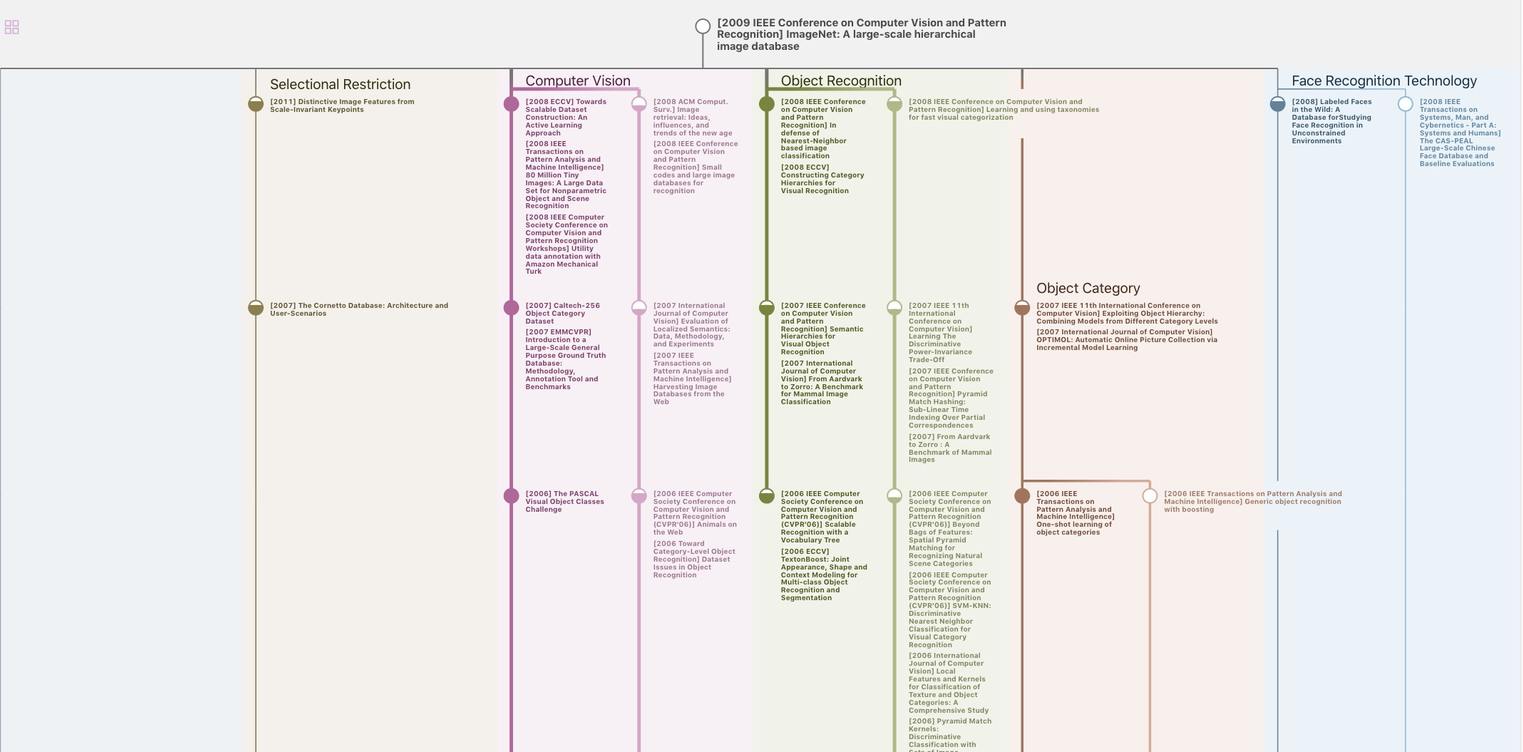
生成溯源树,研究论文发展脉络
Chat Paper
正在生成论文摘要