EEG Signal Processing for Alzheimer's Disorders Using Discrete Wavelet Transform and Machine Learning Approaches
IEEE ACCESS(2022)
摘要
The most common neurological brain issue is Alzheimer's disease, which can be diagnosed using a variety of clinical methods. However, the electroencephalogram (EEG) is shown to be effective in detecting Alzheimer's disease. The purpose of this research is to develop a computer-aided diagnosis system that can diagnose Alzheimer's disease using EEG data. In the present study, a band-pass elliptic digital filter was used to eliminate interference and disturbances from the EEG dataset. Next, the Discrete Wavelet Transform (DWT) technique has been employed to decompose the filtered signal into its frequency bands in order to extract the features of EEG signals. Then, different signal features such as logarithmic band power, standard deviation, variance, kurtosis, average energy, root mean square, and Norm have been integrated into the DWT technique to generate the feature vectors and improve the diagnosis performance. After that, nine machine learning approaches have been investigated to classify EEG features into their corresponding classes: linear discriminant analysis (LDA), quadratic discriminant analysis (QDA), support vector machine (SVM), Naive bayes (NB), k-nearest neighbor (KNN), decision tree (DT), extreme learning machine (ELM), artificial neural network (ANN), and random forests (RF). Finally, the performance of the different proposed machine learning approaches have been compared and evaluated by computing the sensitivity, specificity, overall diagnosis accuracy, and area under the receiver operating characteristic (ROC) curves and plotting the ROC curves and confusion matrices for five classification problems. These investigations aim to compare the proposed approaches and recommend the best combination method for the diagnosis of Alzheimer's disorders. According to the results, the KNN classifier achieved an average classification accuracy of 99.98% with an area under the ROC curve of 100%. Our findings show that the suggested methodologies are an appealing supplementary tool for identifying possible biomarkers to help in the clinical diagnosis of Alzheimer's disease.
更多查看译文
关键词
Electroencephalography, Feature extraction, Alzheimer's disease, Support vector machines, Diseases, Discrete wavelet transforms, Band-pass filters, Bayes methods, Alzheimer's disease, artificial neural network, average energy, decision tree, discrete wavelet transform, electroencephalogram, extreme learning machine, K-nearest neighbor, linear discrimination analysis, logarithmic band power, Naive Bayes, quadratic discriminant analysis, random forests, support vector machines
AI 理解论文
溯源树
样例
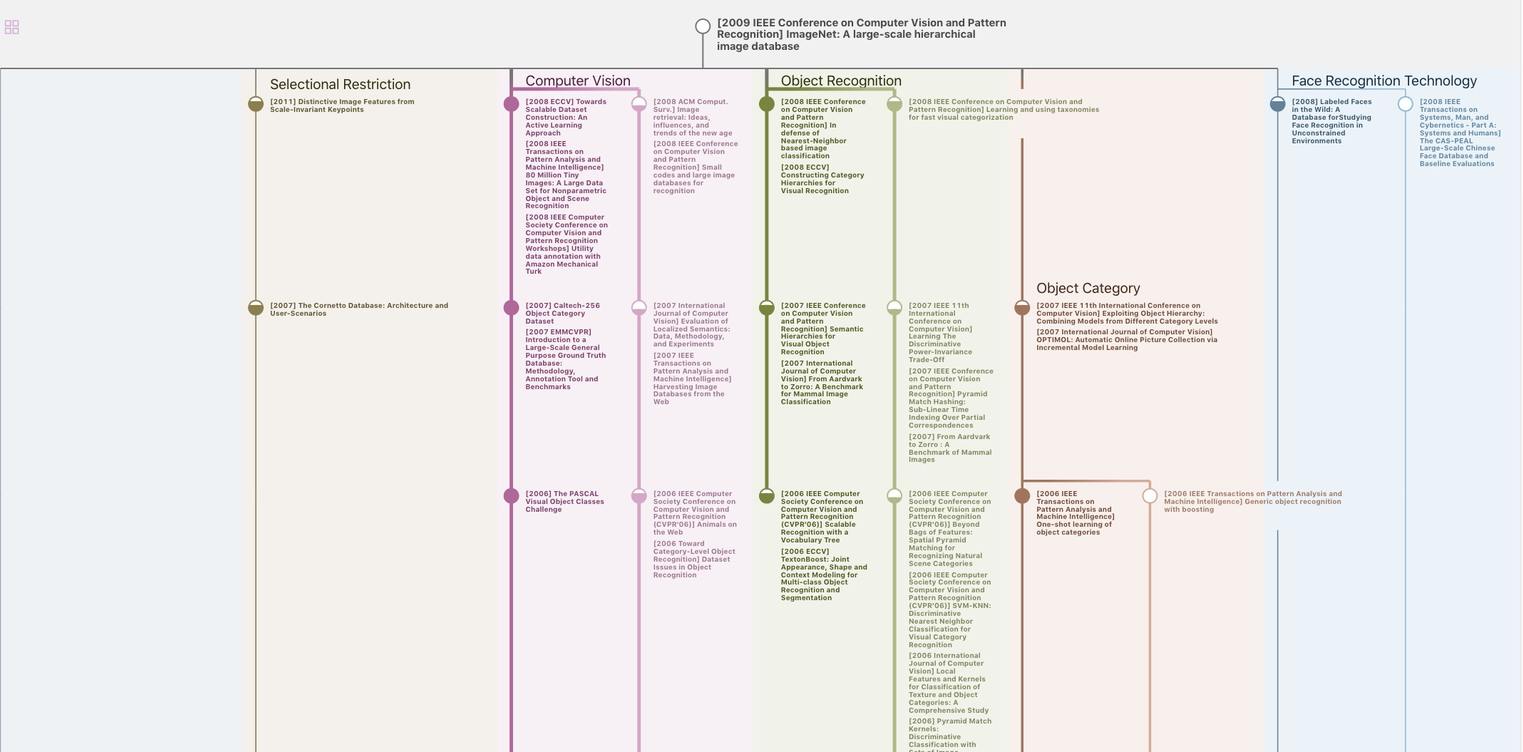
生成溯源树,研究论文发展脉络
Chat Paper
正在生成论文摘要