Neural network temporal quantized lagrange dynamics with cycloidal trajectory for a toe-foot bipedal robot to climb stairs
Applied Intelligence(2022)
摘要
A novel technique for joint angles trajectory tracking control with energy optimization is proposed for a biped robot with toe foot. For the task of climbing stairs by a 9-link biped model, an adaptive cycloid trajectory for the swing phase is planned as a function of the staircase rise/run ratio. We consider Zero Moment Point criteria for satisfying stability constraints. The paper is primarily divided into three sections: 1) Planning stable cycloid trajectory for the initial step and subsequent steps for climbing upstairs. We incorporate inverse kinematics using an unsupervised artificial neural network with a knot shifting procedure for jerk minimization. 2) Developing dynamics for toe-foot biped model using Lagrange formulation along with contact modeling using the spring-damper system. We propose Neural Network Temporal Quantized Lagrange Dynamics, which couples inverse kinematics neural network with dynamics. 3) Using Ant Colony Optimization to tune Proportional-Derivative controller and torso angle in order to minimize joint trajectory errors and total energy consumed. Three cases with variable staircase dimensions have been taken, and a comparison is made to validate the effectiveness of the proposed work. Generated patterns have been simulated in Ⓒ Matlab and MuJoCo.
更多查看译文
关键词
Cycloidal trajectory, Staircase motion planning, Bipedal robot, Unsupervised artificial neural network, Ant Colony Optimization
AI 理解论文
溯源树
样例
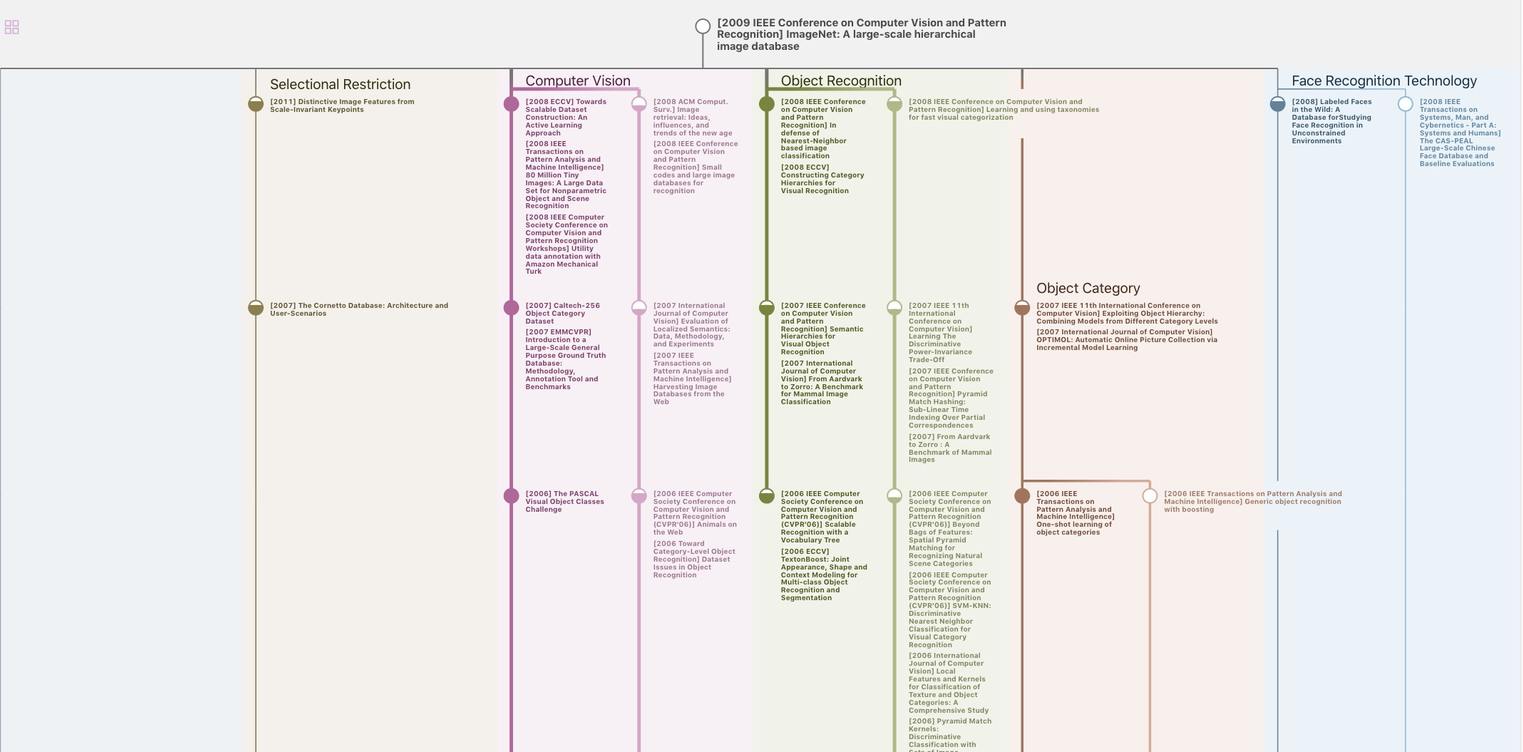
生成溯源树,研究论文发展脉络
Chat Paper
正在生成论文摘要