A Dimensionality Reduction and Reconstruction Method for Data with Multiple Connected Components
2022 IEEE 5th International Conference on Big Data and Artificial Intelligence (BDAI)(2022)
摘要
In the literature on dimensionality reduction, including Spectral Clustering and Laplacian Eigenmaps, one of the core ideas is to reconstruct data based on similarities between data points, which makes the choice of similarity matrices a key factor on the performance of a dimensionality reduction model. Traditional methods like K-nearest neighbor, ∊-neighbor, and Gaussian Kernel for constructing similarity matrices based on data distribution characteristics have been extensively studied. However, these methods usually focus on only a specific level of the data when considering the similarity between data points, which might result in a great flaw in data reconstruction when data possess hierarchical and multiple groups structure. Specifically, such methods can only characterize the similarity between data within a group, but ignore the similarity between different groups. To overcome this deficiency, this paper proposes a hierarchical way of similarity matrix construction, by introducing strong, weak, and intra- and inter-cluster similarities to describe relations between multiple levels. The proposed method can better adapt to complex data with multiple connected components, and the effectiveness of it is verified in a series of experiments on synthetic and real-world datasets.
更多查看译文
关键词
LE,Dimensionality reduction,Manifold learning,Topological connectivity
AI 理解论文
溯源树
样例
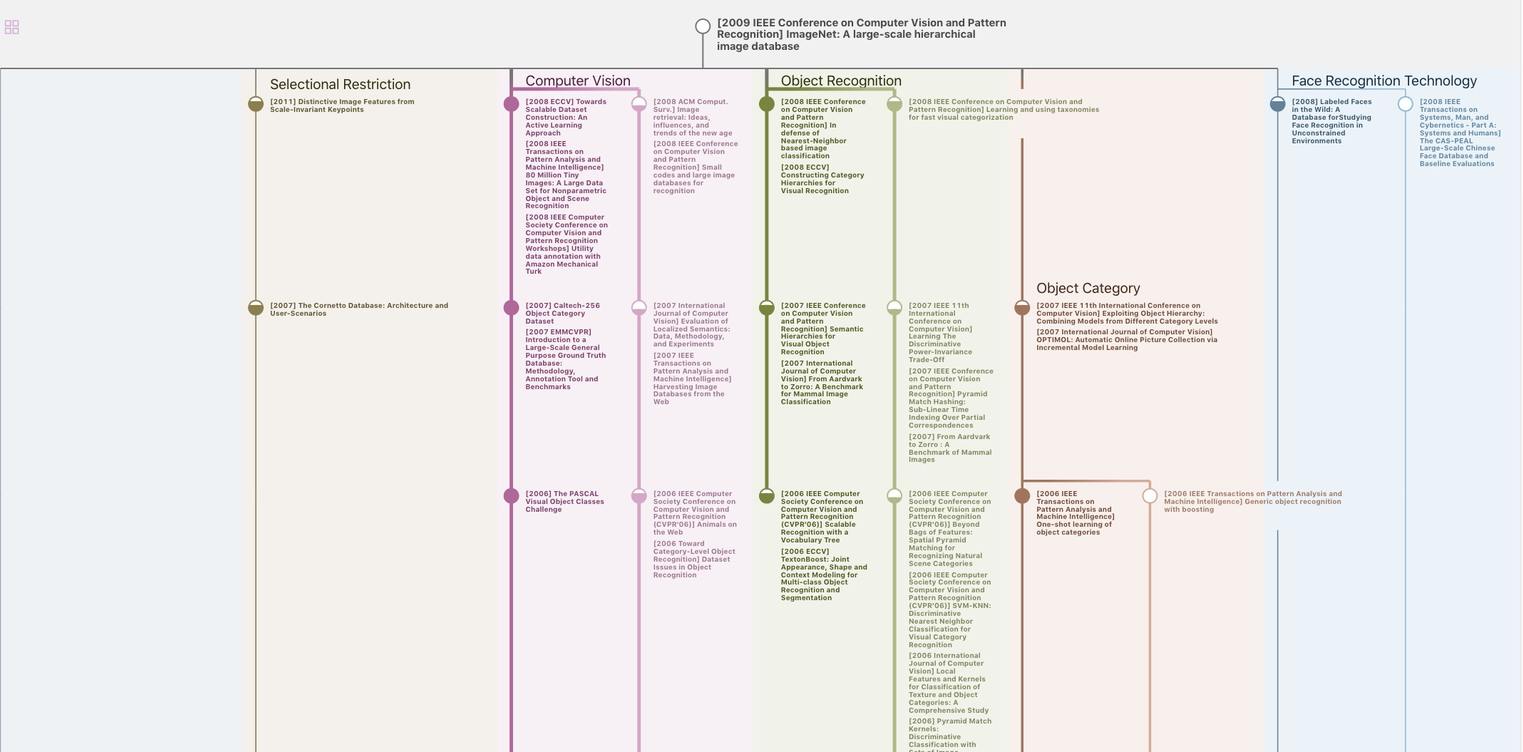
生成溯源树,研究论文发展脉络
Chat Paper
正在生成论文摘要