Multi-channel Fusion Graph Convolution based Critical Node Identification in Temporal Networks
2022 IEEE 23rd International Conference on High Performance Switching and Routing (HPSR)(2022)
摘要
Accurate identification of essential nodes in network topology is crucial to reflect network dynamic behaviors. Traditional static measuring approaches disregard the temporal attribute of node interaction, which misses underlying relevance among nodes. To answer the important evaluation problem of nodes on graphs in time dimension, we propose a new critical node identification approach (MFGCI), which integrates hyper-matrix theory and multi-channel fusion convolution. Specifically, hypermatrix is utilized to express the dynamic evolution of a temporal network, while multi-channel convolution is used to aggregate neighborhood spatial and feature information of nodes across time. Then, MFGCI model is employed to achieve comprehensive ranking of node importance. The experimental results indicate that our MFGCI model outperforms state-of-art baselines on Workspace and Enron data-sets, reflecting its effectiveness and superiority.
更多查看译文
关键词
temporal network,critical nodes identification,supra-adjacency matrix,graph convolutional neural network,global time efficiency
AI 理解论文
溯源树
样例
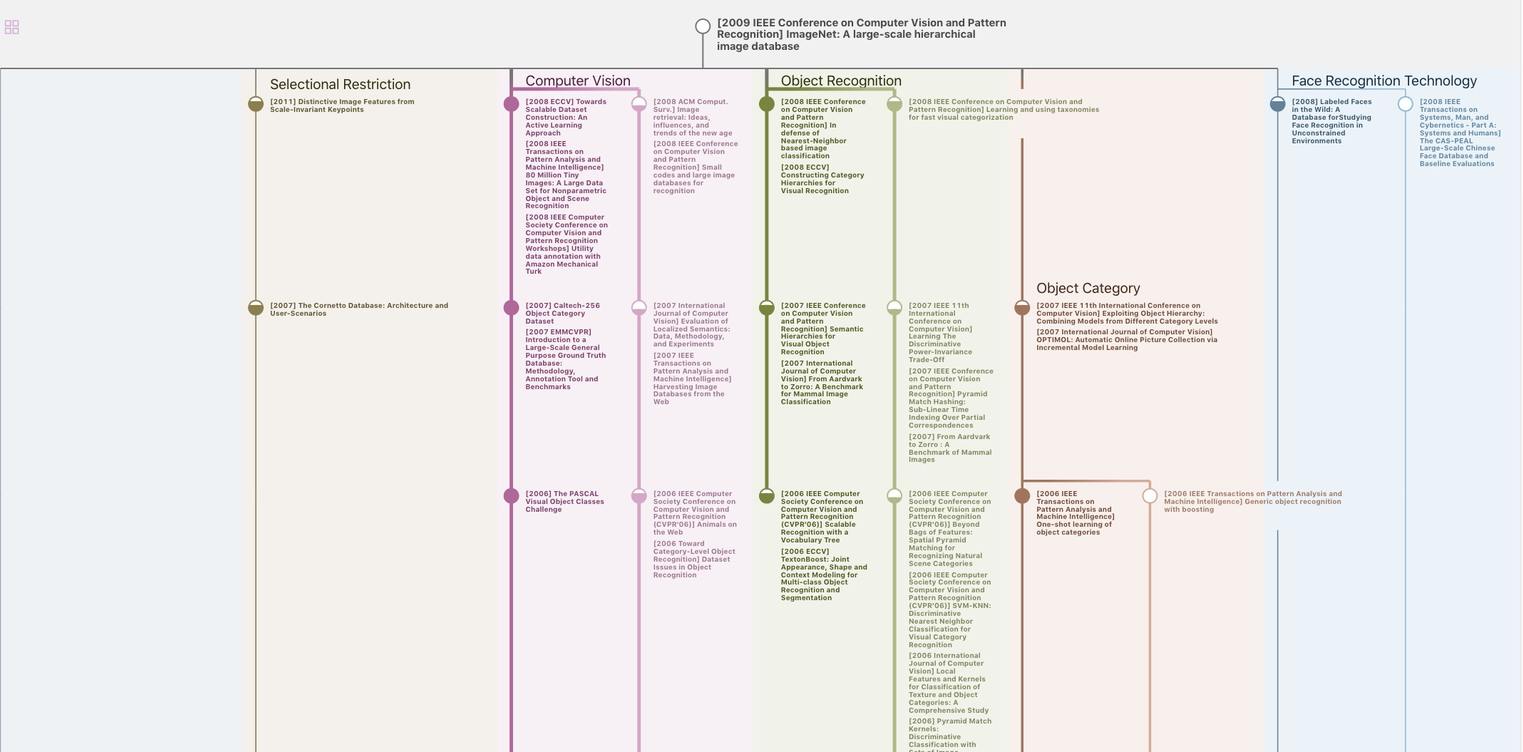
生成溯源树,研究论文发展脉络
Chat Paper
正在生成论文摘要