A Hybrid Duo-Deep Learning and Best Features Based Framework for Action Recognition
CMC-COMPUTERS MATERIALS & CONTINUA(2022)
摘要
Human Action Recognition (HAR) is a current research topic in the field of computer vision that is based on an important application known as video surveillance. Researchers in computer vision have introduced various intelligent methods based on deep learning and machine learning, but they still face many challenges such as similarity in various actions and redundant features. We proposed a framework for accurate human action recognition (HAR) based on deep learning and an improved features optimization algorithm in this paper. From deep learning feature extraction to feature classification, the proposed framework includes several critical steps. Before training fine-tuned deep learning models - MobileNet-V2 and Darknet53 - the original video frames are normalized. For feature extraction, pre-trained deep models are used, which are fused using the canonical correlation approach. Following that, an improved particle swarm optimization (IPSO)-based algorithm is used to select the best features. Following that, the selected features were used to classify actions using various classifiers. The experimental process was performed on six publicly available datasets such as KTH, UT-Interaction, UCF Sports, Hollywood, IXMAS, and UCF YouTube, which attained an accuracy of 98.3%, 98.9%, 99.8%, 99.6%, 98.6%, and 100%, respectively. In comparison with existing techniques, it is observed that the proposed framework achieved improved accuracy.
更多查看译文
关键词
Action recognition, deep learning, features fusion, features selection, recognition
AI 理解论文
溯源树
样例
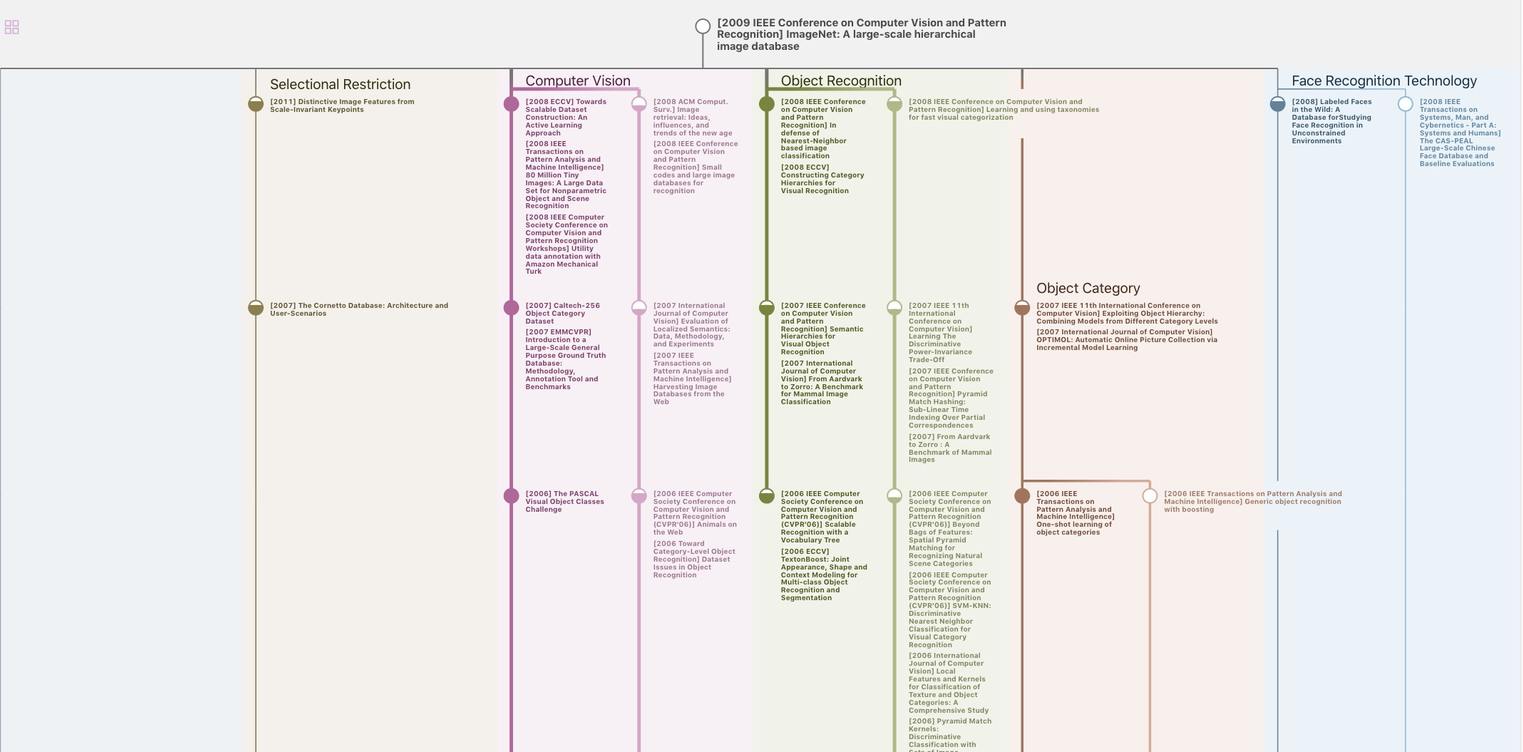
生成溯源树,研究论文发展脉络
Chat Paper
正在生成论文摘要