CNN and ensemble learning based wafer map failure pattern recognition based on local property based features
JOURNAL OF INTELLIGENT MANUFACTURING(2022)
摘要
The combination of feature extraction and classification methods is an often used approach for wafer map failure pattern recognition. Recently, the convolutional neural network (CNN) method is used to raw wafer map data without feature extraction. CNN can improve the accuracy, but the drawback is the expensive computation cost. In our study, we have extracted local property analysis based features and used them with the traditional classification methods, ensemble methods, and CNN for wafer map failure pattern recognition. The decision tree showed better performance than the other methods when evaluating single traditional classification methods. Therefore, we used the decision tree as the base classifier of the proposed ensemble learning. We built a constituent model on a single type of feature set first and then made an ensemble of models to produce the final decision. We found that it is better than directly building a model on total features. Also, we have tested and compared CNN on extracted feature sets and raw wafer map image data. The results showed that raw image based CNN outperforms extracted features under the same number of epochs. However, the training cost of the raw image is much expensive. Furthermore, the performance of extracted features becomes closer to the raw image when increasing the number of epochs with a much lower training cost.
更多查看译文
关键词
Wafer map,Defect pattern recognition,Convolutional neural network,Ensemble learning,Local property,Feature extraction
AI 理解论文
溯源树
样例
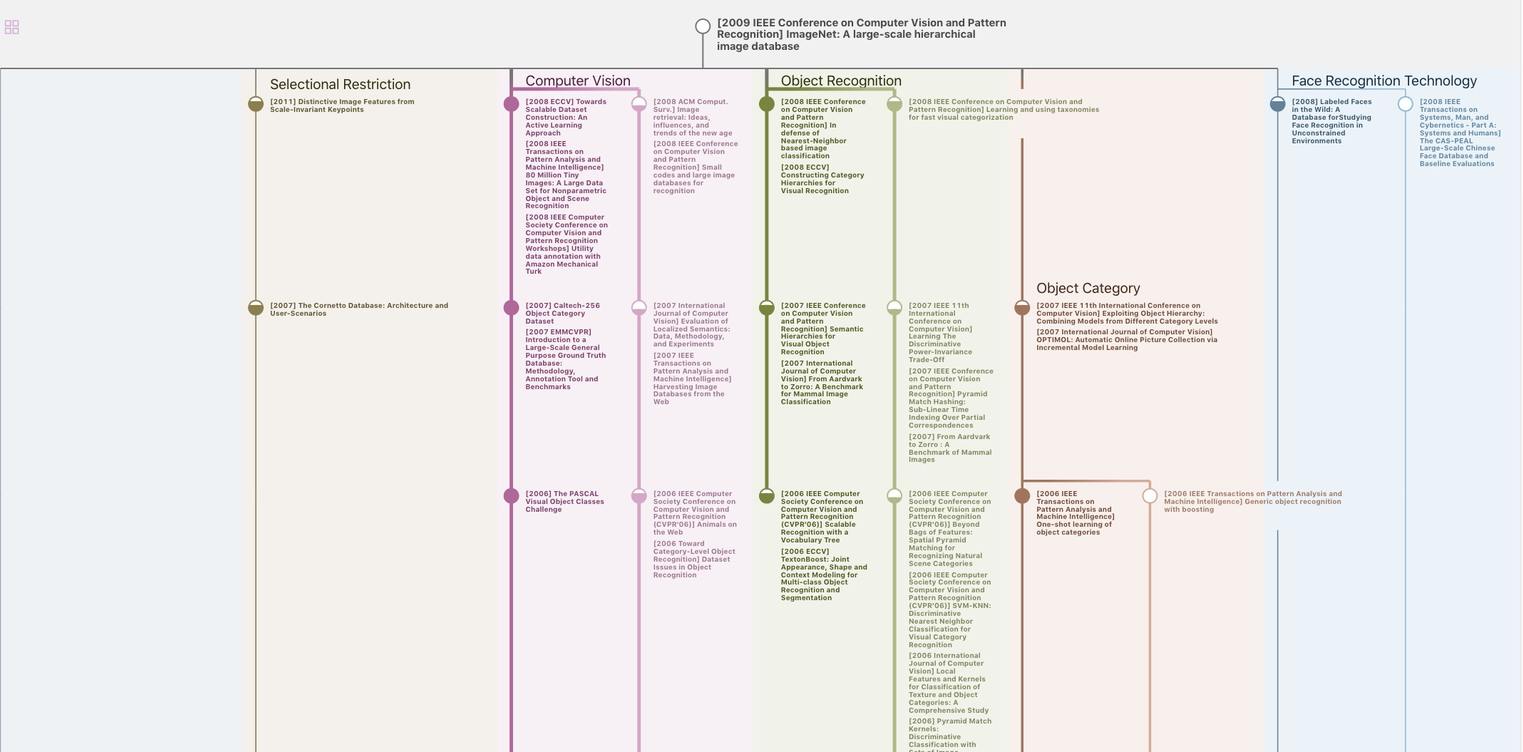
生成溯源树,研究论文发展脉络
Chat Paper
正在生成论文摘要