Defect Detection of Integrated Circuit Based on YOLOv5
2022 IEEE 2nd International Conference on Computer Communication and Artificial Intelligence (CCAI)(2022)
摘要
In Integrated Circuit (IC) manufacturing, defect detection is a necessary task. The small size and high density of IC, and the complex characteristics of various defects, which burdens the defect detection system. It is difficult for the existing detection methods to accurately detect various types of defects while ensuring the detection speed. We implement a deep convolutional neural network in IC defect detection, based on YOLOv5, we add a prediction head to detect objects at different scales. In addition, we also integrate the Squeeze-and-Excitation layer (SELayer) to help the detection network find attention region on scenarios with dense objects, extract key features, enhance the network’s ability to detect difficult-to-detect samples, and generally improve the accuracy of the detection network. Extensive experiments on the IC defect dataset made by us show that the AP result of YOLOv5 with integrated attention module in IC defect detection is 95.4%, which is 0.5% better than the network without SELayer. Compared with other detection networks, it has obvious accuracy advantage, which is encouraging and promising competitive.
更多查看译文
关键词
automatic defect detection,IC,YOLOv5,deep convolutional neural network
AI 理解论文
溯源树
样例
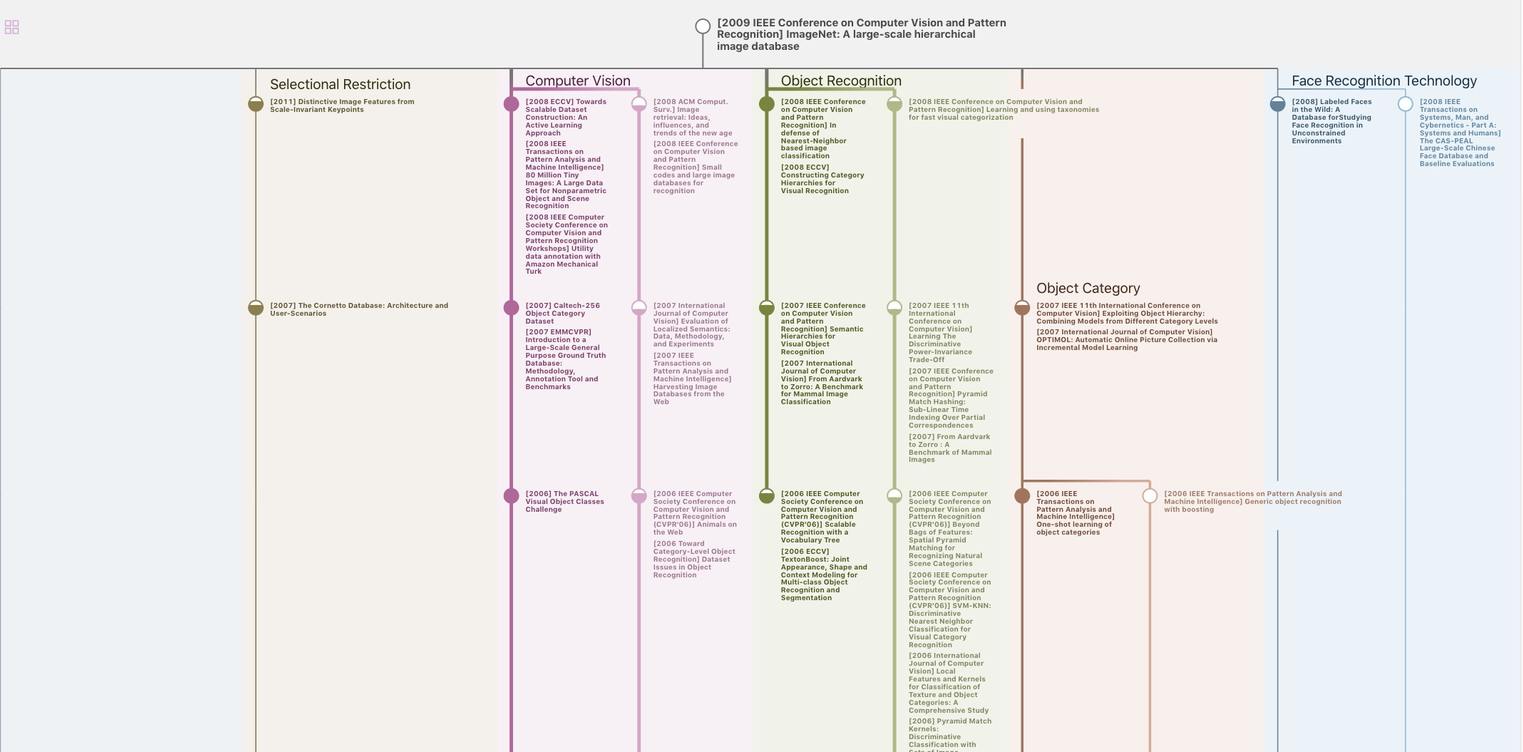
生成溯源树,研究论文发展脉络
Chat Paper
正在生成论文摘要