iSample: Intelligent Client Sampling in Federated Learning
2022 IEEE 6th International Conference on Fog and Edge Computing (ICFEC)(2022)
摘要
The pervasiveness of AI in society has made machine learning (ML) an invaluable tool for mobile and internet-of-things (IoT) devices. While the aggregate amount of data yielded by those devices is sufficient for training an accurate model, the data available to any one device is limited. Therefore, augmenting the learning at any of the devices with the experience from observations associated with the rest of the devices will be necessary. This, however, can dramatically increase the bandwidth requirements. Prior work has led to the development of Federated Learning (FL), where instead of exchanging data, client devices can only share weights to learn from one another. However, het-erogeneity in device resource availability and network conditions still impose limitations on training performance. In order to improve performance while maintaining good levels of accuracy, we introduce iSample. iSample, an intelligent sampling technique, selects clients by jointly considering known network performance and model quality parameters, allowing the minimization of training time. We compare iSample with other federated learning approaches and show that iSample improves the performance of the global model, especially in the earlier stages of training, while decreasing the training time for both CNN and VGG by 27% and 39%, respectively.
更多查看译文
关键词
federated learning,heterogeneous systems,resource constrained devices,edge computing,machine learning
AI 理解论文
溯源树
样例
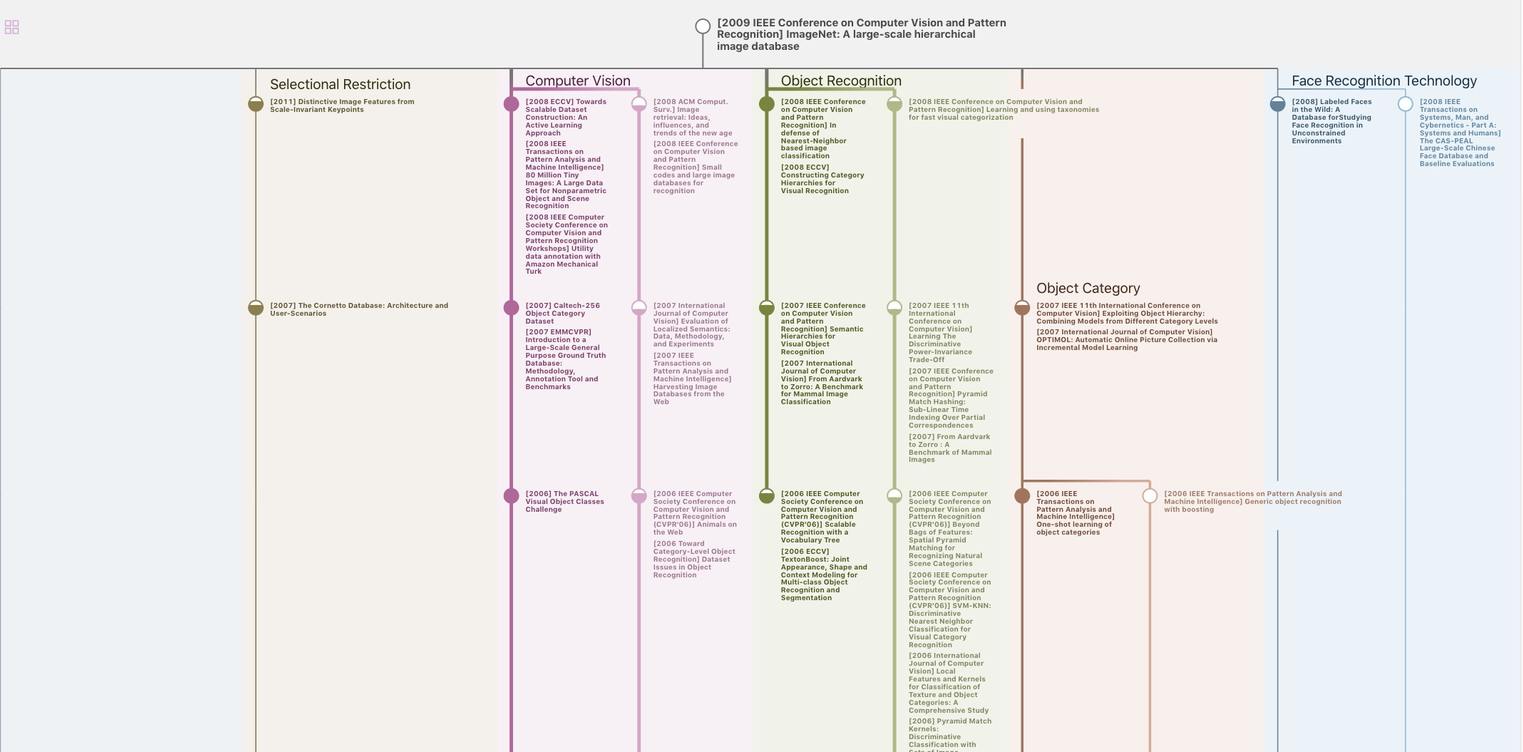
生成溯源树,研究论文发展脉络
Chat Paper
正在生成论文摘要