Kernel-based multiagent reinforcement learning for near-optimal formation control of mobile robots
Applied Intelligence(2022)
摘要
Feature representation is a major issue to be addressed for learning-based control of multiagent systems. In this paper, a kernel-based multiagent reinforcement learning (KMARL) algorithm for formation control of wheeled mobile robots with nonholonomic constraints is proposed. In the proposed algorithm, by integrating kernel machines into the basis functions, the formation controller is endowed with the ability to automatically construct the features of the coupled value function. The solution to the coupled Hamilton–Jacobi–Bellman (CHJB) equation is learned by means of a distributed actor–critic algorithm to achieve near-optimal formation control. Simulations are conducted on formation control for multiple robots to illustrate the effectiveness of the proposed algorithm. The simulation results demonstrate that compared with previous formation control methods such as displacement-based multi-agent control, and multi-agent actor-critic learning based on neural networks, the formation control costs of the proposed KMARL method can be reduced by 24.49 % and 16.59 % , respectively.
更多查看译文
关键词
Reinforcement learning, Kernel machines, Learning-based control, Mobile robots, Formation control
AI 理解论文
溯源树
样例
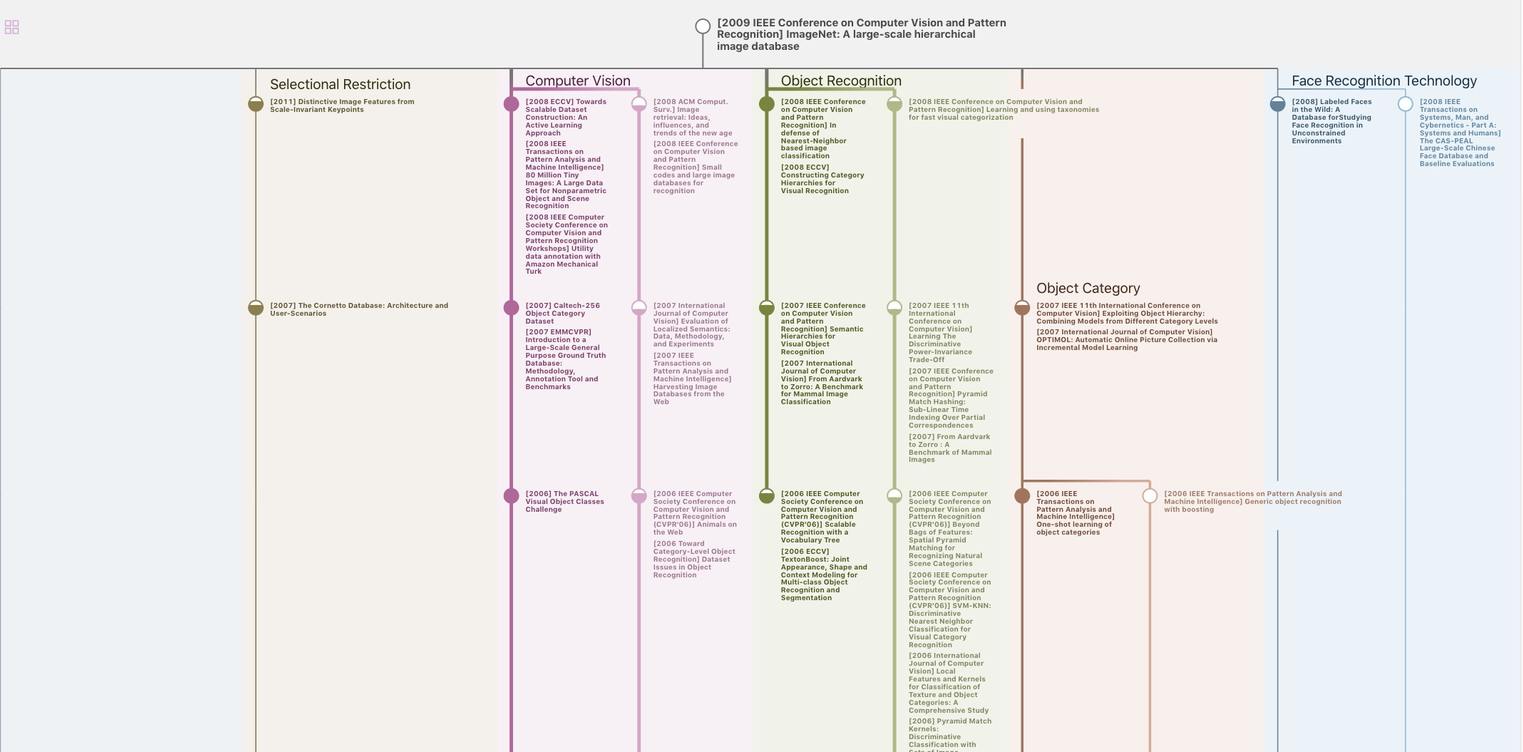
生成溯源树,研究论文发展脉络
Chat Paper
正在生成论文摘要