Contrastive Learning-Based Domain Adaptation for Semantic Segmentation
2022 National Conference on Communications (NCC)(2022)
摘要
Semantic segmentation is a crucial algorithm for identifying various objects in the surrounding of an autonomous vehicle. However, due to the limited size of real-world datasets, domain adaptation is employed. Hence, the models are made to adapt to real-world settings while being trained on large-scale synthetic datasets. In domain adaptation, domain-invariant features play a significant role in learning domain agnostic representations for each predefined category. While most of the prior work focuses on decreasing the distance between the domains, the works that utilize contrastive objectives for learning domain-invariant features depend heavily on the augmentations used. In this work, we completely eradicate the requirement of explicit data augmentations. We hypothesize that real-world images and their corresponding synthetic images are different views of the same abstract representation. To enhance the quality of domain-invariant features, we increase the mutual information between the two inputs. We first validate our hypothesis on the classification task using the standard datasets; Office31 and VisDA-2017. Further, we perform quantitative and qualitative analysis on the segmentation task using SYNTHIA, GTA and Cityscapes datasets.
更多查看译文
关键词
Autonomous Vehicles,Semantic Segmentation,Self-Supervised Learning,Domain Adaptation
AI 理解论文
溯源树
样例
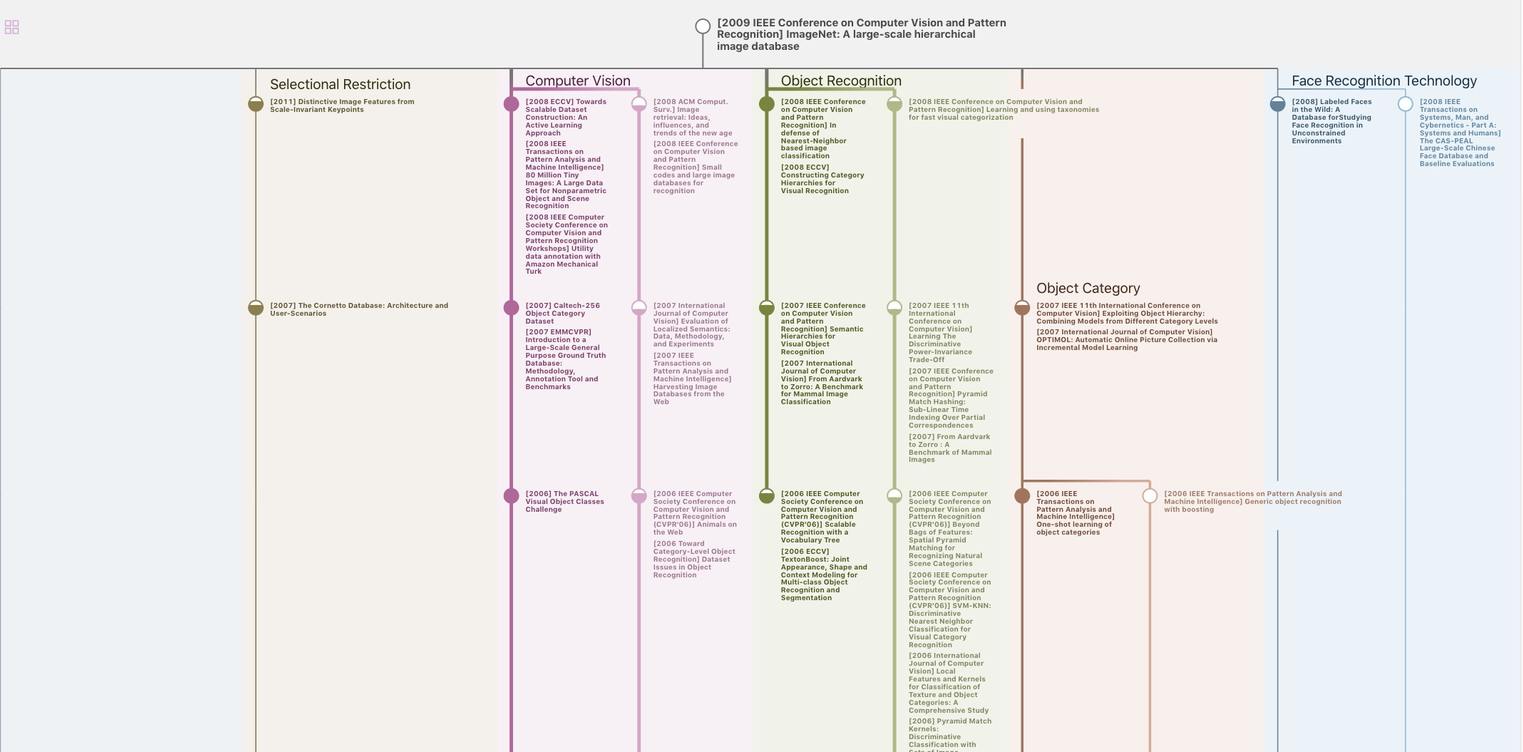
生成溯源树,研究论文发展脉络
Chat Paper
正在生成论文摘要